Vision Transformer Enhances Treatment for Recurrent Liver Cancer
In the evolving landscape of oncology and medical AI, a groundbreaking study has recently illuminated a promising advance in the treatment of recurrent hepatocellular carcinoma (HCC), one of the most challenging and deadly forms of liver cancer. Researchers led by Zhang, K., Ru, J., Wang, W., and their colleagues have utilized a sophisticated vision transformer-based […]

In the evolving landscape of oncology and medical AI, a groundbreaking study has recently illuminated a promising advance in the treatment of recurrent hepatocellular carcinoma (HCC), one of the most challenging and deadly forms of liver cancer. Researchers led by Zhang, K., Ru, J., Wang, W., and their colleagues have utilized a sophisticated vision transformer-based model to optimize curative-intent treatment strategies for patients suffering from this aggressive disease. Published in Nature Communications in 2025, this work marks a significant leap in integrating deep learning methodologies with clinical decision-making to enhance patient outcomes in oncology.
Hepatocellular carcinoma is notorious for its high recurrence rate and limited curative options once it returns, posing a significant clinical challenge worldwide. Traditional treatment approaches, including resection, ablation, and transarterial therapies, often struggle to provide durable remission due to tumor heterogeneity and complex microenvironmental factors. The advent of artificial intelligence (AI) and, more specifically, vision transformers in medical imaging offers a novel lens through which to interpret complex radiological data, potentially transforming personalized oncologic care.
Unlike conventional convolutional neural networks (CNNs), vision transformers rely on a self-attention mechanism that excels in capturing both global and local contextual information from imaging data. This ability is critical in HCC, where tumors present with varied morphologic and vascular characteristics across sequential scans. By applying vision transformers to multimodal imaging datasets, the research team developed a computational framework that not only discerns subtle imaging features linked to tumor aggressiveness but also predicts the most effective treatment path for recurrent cases.
The technical innovation lies in the model’s architecture, which divides radiological images into patches, encoding spatial relationships and integrating disparate imaging biomarkers. This method contrasts with pixel-based strategies, enabling a richer and more holistic understanding of tumor phenotype. The model was trained using a large, annotated dataset comprising dynamic contrast-enhanced MRI and CT images from patients with recurrent HCC, incorporating clinical parameters to enhance predictive accuracy.
One of the critical findings from this study is the model’s ability to stratify patients based on their response to curative-intent treatments, including repeat hepatectomy, ablation, and combined therapies. Traditionally, selecting an intervention involves balancing tumor size, location, liver function, and patient overall health, but the new model refines this process by simulating treatment outcomes with unprecedented precision. The predictive capabilities can guide clinicians towards personalized treatment choices that maximize the likelihood of long-term remission.
Furthermore, the study details rigorous validation protocols, incorporating cross-institutional cohorts to address generalizability and reduce biases often associated with AI models trained on single-center data. The model maintained robust performance metrics across diverse patient populations, signaling its potential scalability for clinical deployment. This emphasis on external validation is crucial for gaining regulatory approval and clinician trust, two barriers often limiting AI integration in healthcare.
Technical challenges, such as harmonizing imaging protocols across different scanners and centers, were overcome using novel normalization techniques embedded in the vision transformer architecture. These adaptations ensure that the model remains resilient to variations in imaging quality and parameters, a perennial issue in medical AI research. This robustness is integral to its utility in real-world clinical environments where standardization is frequently lacking.
Beyond treatment optimization, the model sheds light on underlying biological mechanisms driving treatment resistance and recurrence in HCC. By correlating imaging features with molecular data, the research offers insights into tumor heterogeneity and microenvironmental interactions that may influence therapeutic efficacy. This fusion of radiomics and genomics, mediated by advanced AI, opens new avenues for biomarker discovery and targeted therapy development.
The clinical implications extend to health economics as well. By precisely tailoring treatments, the model promises to reduce unnecessary interventions, minimize adverse effects, and improve quality-adjusted life years for patients. In healthcare systems burdened by rising costs and limited resources, such AI-driven tools represent a compelling strategy to enhance value-based care in oncology.
This research also underlines the importance of interdisciplinary collaboration between computer scientists, radiologists, oncologists, and pathologists. The integration of domain expertise into AI model training and interpretation ensures that the output is clinically meaningful and actionable. The study serves as a blueprint for future AI applications aiming to tackle complex, multifactorial diseases beyond liver cancer.
While poised to revolutionize recurrent HCC treatment paradigms, the authors caution that prospective clinical trials are essential to validate the model’s utility further and assess long-term outcomes. Ethical considerations regarding patient data privacy and algorithmic transparency were also highlighted, advocating for frameworks that safeguard patient rights while fostering innovation.
The study’s open-access publication and sharing of de-identified datasets underscore a commitment to open science, facilitating external validation and encouraging the global research community to build upon these findings. This openness is vital for accelerating AI advancements in oncology and democratizing access to novel diagnostic and therapeutic tools.
In conclusion, the deployment of a vision transformer-based model for optimizing curative-intent treatment in recurrent hepatocellular carcinoma represents a paradigm shift in precision oncology. By leveraging cutting-edge AI algorithms to decode complex imaging and clinical data, the study offers a powerful instrument to refine treatment decisions, improve patient prognoses, and ultimately transform the clinical management of one of the most intractable liver malignancies. As this technology moves closer to routine clinical application, it heralds a new era where AI serves as an indispensable partner in cancer care.
Subject of Research:
Optimization of curative-intent treatment strategies for recurrent hepatocellular carcinoma using vision transformer-based AI models.
Article Title:
Vision transformer-based model can optimize curative-intent treatment for patients with recurrent hepatocellular carcinoma.
Article References:
Zhang, K., Ru, J., Wang, W. et al. Vision transformer-based model can optimize curative-intent treatment for patients with recurrent hepatocellular carcinoma. Nat Commun 16, 4081 (2025). https://doi.org/10.1038/s41467-025-59197-0
Image Credits:
AI Generated
Tags: Advanced Imaging Techniques for CancerArtificial Intelligence in Liver Cancerclinical decision-making in oncologydeep learning for medical imagingenhancing patient outcomes with AIFuture of Oncology with AI IntegrationHigh Recurrence Rate of Liver CancerOptimizing Treatment Strategies for HCCPersonalized Cancer Care InnovationsRecurrent Hepatocellular Carcinoma TreatmentTumor Heterogeneity in Liver CancerVision Transformer in Oncology
What's Your Reaction?
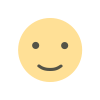
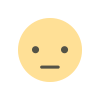
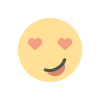
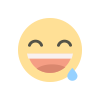
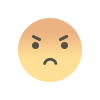
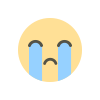
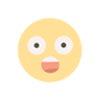