Machine Learning Predicts Lung Cancer Brain Metastases
In a groundbreaking stride towards the integration of artificial intelligence in oncology, a recent systematic review and meta-analysis has illuminated the powerful capabilities of machine learning (ML) models in predicting epidermal growth factor receptor (EGFR) mutation status in non-small cell lung cancer (NSCLC) brain metastases. This advancement holds tremendous promise in transforming clinical decision-making processes […]

In a groundbreaking stride towards the integration of artificial intelligence in oncology, a recent systematic review and meta-analysis has illuminated the powerful capabilities of machine learning (ML) models in predicting epidermal growth factor receptor (EGFR) mutation status in non-small cell lung cancer (NSCLC) brain metastases. This advancement holds tremendous promise in transforming clinical decision-making processes and tailoring personalized treatment strategies for one of the most challenging cancer complications. The comprehensive study, published in BMC Cancer, meticulously evaluated existing ML-based predictive models, demonstrating their impressive accuracy and diagnostic performance in distinguishing EGFR mutations, which are crucial biomarkers linked to patient prognosis.
Non-small cell lung cancer remains a leading cause of cancer-related mortality worldwide, with brain metastases representing a frequent and serious progression of the disease. EGFR mutations, prevalent in a substantial subset of NSCLC patients, profoundly influence therapeutic responses and survival outcomes. Accurate identification of these genetic alterations is paramount yet often hindered by the invasive nature and logistical limitations of biopsy procedures. Herein lies the transformative potential of machine learning models: by harnessing vast datasets and imaging biomarkers, ML offers a non-invasive, reliable alternative for inferring EGFR status, thereby optimizing patient stratification and clinical management.
The authors conducted an exhaustive search across four major scientific databases—PubMed, Embase, Scopus, and Web of Science—up to December 20, 2024, to collate and analyze studies that assessed predictive models for EGFR mutation status specifically in NSCLC patients with brain metastases. In total, twenty studies encompassing 3517 patients and 6205 metastatic lesions were incorporated into the meta-analysis, underscoring the robustness of the dataset. The synthesis of this data revealed that the majority of top-performing models utilized traditional machine learning algorithms, while deep learning (DL) approaches represented a significant but smaller fraction.
Performance metrics of the best models were notably high, with area under the curve (AUC) values ranging from 0.765 to 1 and accuracy percentages between 69% and 93%. The pooled analyses across studies underscored an exceptional pooled AUC of 0.91 and an accuracy of 82%. Additionally, the models exhibited high sensitivity and specificity, at 87% and 86% respectively. These results mark a critical validation checkpoint, indicating that ML models can reliably discern EGFR mutation status from clinical and radiological data in patients suffering from brain metastases. Notably, no statistically significant performance difference was found between classical ML and deep learning models, suggesting that both methodologies have matured to a comparable efficacy in this domain.
The significance of this finding cannot be overstated. Machine learning algorithms facilitate rapid integration of multi-modal data—ranging from radiographic imaging to genomic features—enabling the construction of predictive frameworks that transcend conventional diagnostic barriers. By capitalizing on pattern recognition and feature extraction capacities inherent to these algorithms, clinicians are equipped with potent tools capable of overcoming the heterogeneity of NSCLC brain metastases and delivering precision oncology care. Moreover, the non-invasive nature of these techniques circumvents the risks and systemic burdens of invasive tissue sampling.
Machine learning’s ascendancy in medical diagnostics aligns with the broader paradigm shift towards personalized medicine. As precision oncology evolves, the need for accurate, timely, and patient-friendly diagnostic approaches is escalating. This study’s affirmation of ML’s predictive prowess in EGFR mutation status is thus a clarion call for the integration of AI-powered models into routine clinical workflows. Such integration promises not only enhanced therapeutic targeting but also potential reductions in healthcare costs and improved patient quality of life.
Beyond predictive accuracy, the ability of ML models to glean intricate biological insights from imaging and clinical data offers the potential for novel biomarker discovery. The meta-analysis highlights how supervised learning methods can parse subtle radiomic features invisible to the human eye, capturing the complex tumor microenvironment and genetic signatures associated with metastasis and treatment resistance. Importantly, these capabilities open avenues for real-time monitoring of tumor evolution and dynamic treatment adaptation, areas ripe for future research and clinical innovation.
One challenge highlighted by the systematic review is the need for standardized protocols and annotated datasets to optimize the training and validation of machine learning algorithms. Variability in data quality, imaging modalities, and patient demographics across studies can introduce biases and affect generalizability. Addressing these issues through collaborative multi-institutional efforts and broader data-sharing initiatives will be essential to fully realize the clinical translation of these tools.
Furthermore, the interpretation and transparency of machine learning models remain critical considerations in clinical adoption. While deep learning architectures, such as convolutional neural networks, provide remarkable accuracy, their “black-box” nature can hinder clinician trust and regulatory approval. The study’s observation that traditional machine learning methods perform comparably opens the door for employing more interpretable models that balance predictive power with explainability, fostering confidence among healthcare providers.
The successful application of ML in predicting EGFR status in NSCLC brain metastases also underscores the expanding role of artificial intelligence in oncology beyond primary tumors. Metastatic lesions present unique biological characteristics and therapeutic challenges, and these findings affirm that AI-driven diagnostics can adeptly handle these complexities. This paves the way for comprehensive, AI-assisted cancer care that spans the entire disease spectrum, from diagnosis through treatment and follow-up.
Clinicians and researchers should be encouraged by the meta-analysis’s demonstration that AI-based predictive tools have reached a maturity level conducive to clinical implementation. The convergence of machine learning technology with routine neuro-oncological practice could revolutionize care by enabling earlier identification of actionable mutations, personalized treatment plans, and improved patient prognosis. Ultimately, this translates into a more efficient, precise, and patient-centric oncology landscape.
Significantly, the study concludes with optimism regarding the integration of ML models into everyday clinical practice. The promise of these tools lies not only in their diagnostic performance but also in their potential to optimize treatment pathways, reduce unnecessary procedures, and enhance radiological assessment accuracy. This multifaceted impact signifies a leap forward in managing one of the most daunting complications of lung cancer—brain metastasis.
As medical science continues to harness the power of machine learning, the collaboration between data scientists, oncologists, and radiologists will be paramount. Such interdisciplinary approaches are vital to refine algorithms, expand applicability, and ensure ethical deployment in clinical environments. The meta-analysis stands as a testament to the fruits of such cooperation, showcasing how advanced computational methods can deliver tangible benefits for patient care.
Looking ahead, future research should focus on prospective validation studies and the integration of multi-omics data to enhance the robustness of predictive models. Incorporating real-world clinical data and exploring adaptive, self-learning algorithms could further elevate performance and clinical relevance. These initiatives will be critical in transitioning these promising ML approaches from research settings to frontline clinical decision-making tools.
In conclusion, this comprehensive evaluation of machine learning-based models marks a significant milestone in the quest to improve diagnostic precision for EGFR mutations in NSCLC brain metastases. It provides compelling evidence that AI-driven technologies are not just theoretical constructs but practical, impactful instruments capable of reshaping cancer diagnostics and treatment. As the clinical oncology community embraces these advances, patients stand to benefit from more informed, targeted, and effective therapeutic interventions.
Subject of Research: Prediction of epidermal growth factor receptor (EGFR) mutation status in non-small cell lung cancer (NSCLC) brain metastases using machine learning-based models.
Article Title: Machine learning in prediction of epidermal growth factor receptor status in non-small cell lung cancer brain metastases: a systematic review and meta-analysis.
Article References:
Hajikarimloo, B., Mohammadzadeh, I., Tos, S.M. et al. Machine learning in prediction of epidermal growth factor receptor status in non-small cell lung cancer brain metastases: a systematic review and meta-analysis. BMC Cancer 25, 818 (2025). https://doi.org/10.1186/s12885-025-14221-w
Image Credits: Scienmag.com
DOI: https://doi.org/10.1186/s12885-025-14221-w
Tags: advancements in cancer researchartificial intelligence in cancer carecancer biomarkers and prognosisdiagnostic performance of ML modelsEGFR mutation status predictionmachine learning in oncologynon-invasive cancer diagnosticsnon-small cell lung cancer treatmentoptimizing patient stratificationpersonalized cancer treatment strategiespredicting lung cancer brain metastasessystemic review of predictive models
What's Your Reaction?
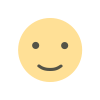
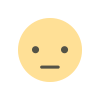
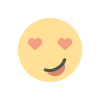
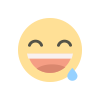
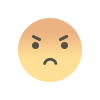
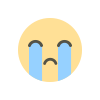
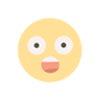