Rewrite Gadoxetic acid-enhanced MRI for identifying cholangiocyte phenotype hepatocellular carcinoma by interpretable machine learning: individual application of SHAP as a headline for a science magazine post, using no more than 7 words
Gadoxetic acid-enhanced MRI for identifying cholangiocyte phenotype hepatocellular carcinoma by interpretable machine learning: individual application of SHAP BMC Cancer volume 25, Article number: 788 (2025) Cite this article Purpose Cholangiocyte phenotype hepatocellular carcinoma (HCC) is highly invasive. This study aims to develop and validate an optimal machine learning model to predict cholangiocyte phenotype HCC based on T1 mapping […]

Gadoxetic acid-enhanced MRI for identifying cholangiocyte phenotype hepatocellular carcinoma by interpretable machine learning: individual application of SHAP
BMC Cancer
volume 25, Article number: 788 (2025)
Cite this article
Purpose
Cholangiocyte phenotype hepatocellular carcinoma (HCC) is highly invasive. This study aims to develop and validate an optimal machine learning model to predict cholangiocyte phenotype HCC based on T1 mapping gadoxetic acid-enhanced MRI and to implement individual applications via the Shapley Additive explanation (SHAP).
Methods
We included 180 patients with histologically confirmed HCC from two institutions. Clinical and MRI imaging features were screened for predicting cholangiocyte phenotype hepatocellular carcinoma using Least Absolute Shrinkage and Selection Operator (LASSO) and the logistic regression analysis. Five machine learning models were constructed based on these features. A Kaplan–Meier survival analysis aims to compare prognostic differences between cholangiocyte phenotype-positive HCC groups and classical (cholangiocyte phenotype-negative) HCC groups, and was conducted to explore the prognostic information of the optimal model.
Results
The most significant clinicoradiological features, including the platelet-to-lymphocyte ratio (PLR), tumor capsule, target sign on hepatobiliary phase (HBP), and T1 relaxation time of 20 min (T1rt-20 min), were selected to construct the prediction model. Finally, we selected the eXtreme Gradient Boosting (XGBoost) model as the optimal predictive model, which achieved AUCs of 0.835, 0.830, 0.816 and 0.776 in training, internal validation, external validation, and prospective validation cohorts, respectively, for visual analysis via SHAP, in which T1rt-20 min made a significant contribution. Survival analysis showed a statistically significant difference in relapse-free survival (RFS) between cholangiocyte phenotype-positive HCC groups and classical HCC groups from institution I (hazard ratio [HR] 1.994; 95% CI, 1.059–3.758; P = 0.027), and the construction XGBoost model can be used to stratify RFS according to prognosis (HR, 1.986; 95% CI, 1.061–3.717; P = 0.029).
Conclusion
The machine learning model utilizing T1 mapping gadoxetic acid-enhanced MRI demonstrates significant potential in identifying cholangiocyte phenotype HCC. Furthermore, personalized prediction is enhanced through the application of SHAP, providing valuable insights to support clinical decision-making processes.
Liu, W., Cai, Z., Chen, Y. et al. Gadoxetic acid-enhanced MRI for identifying cholangiocyte phenotype hepatocellular carcinoma by interpretable machine learning: individual application of SHAP.
BMC Cancer 25, 788 (2025). https://doi.org/10.1186/s12885-025-14147-3
https://doi.org/10.1186/s12885-025-14147-3 bu içeriği en az 2500 kelime olacak şekilde ve alt başlıklar ve madde içermiyecek şekilde ünlü bir science magazine için İngilizce olarak yeniden yaz. Teknik açıklamalar içersin ve viral olacak şekilde İngilizce yaz. Haber dışında başka bir şey içermesin. Haber içerisinde en az 14 paragraf ve her bir paragrafta da en az 50 kelime olsun. Cevapta sadece haber olsun. Ayrıca haberi yazdıktan sonra içerikten yararlanarak aşağıdaki başlıkların bilgisi var ise haberin altında doldur. Eğer bilgi yoksa ilgili kısmı yazma.:
Subject of Research:
Article Title:
Article References:
Liu, W., Cai, Z., Chen, Y. et al. Gadoxetic acid-enhanced MRI for identifying cholangiocyte phenotype hepatocellular carcinoma by interpretable machine learning: individual application of SHAP.
BMC Cancer 25, 788 (2025). https://doi.org/10.1186/s12885-025-14147-3
Image Credits: Scienmag.com
DOI: https://doi.org/10.1186/s12885-025-14147-3
Keywords
Tags: cholangiocyte phenotype identificationGadoxetic acid-enhanced MRIhepatocellular carcinoma diagnosisindividual patient applications in oncologyinterpretable machine learning applicationsinvasive liver cancer detectionLASSO regression in cancer researchmachine learning in medical imagingMRI imaging features analysispredictive modeling in healthcareSHAP method in oncologyT1 mapping in MRI
What's Your Reaction?
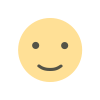
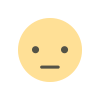
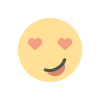
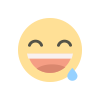
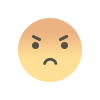
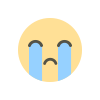
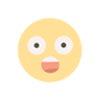