Revolutionary Nano-Architected Materials: Combining Machine Learning and Nano-3D Printing for Unmatched Strength and Lightness
Researchers at the University of Toronto are pioneering a groundbreaking approach to the development of nano-architected materials, successfully combining lightweight characteristics akin to Styrofoam with the remarkable strength of carbon steel. Through the innovative application of machine learning, their findings promise to revolutionize multiple industries, particularly those reliant on advanced materials such as aerospace and […]

Researchers at the University of Toronto are pioneering a groundbreaking approach to the development of nano-architected materials, successfully combining lightweight characteristics akin to Styrofoam with the remarkable strength of carbon steel. Through the innovative application of machine learning, their findings promise to revolutionize multiple industries, particularly those reliant on advanced materials such as aerospace and automotive engineering, where both strength and weight are critical considerations.
In a recent study published in the esteemed journal Advanced Materials, the research team, led by Professor Tobin Filleter, unveiled their insights on how they engineered materials that uniquely blend strength, lightweight, and customizable features. This research addresses a longstanding challenge in material science—the production of incredibly strong materials that do not compromise on the necessary lightness essential for practical applications. Nano-architected materials utilize small, repeating units, which at times can be mere fractions of the size of human hairs, to produce structures capable of enduring substantial mechanical loads without significant weight.
The principles behind nano-architected materials lean heavily on structural mechanics, specifically the concept that “smaller is stronger.” This notion suggests that when structures are designed at the nanoscale, certain physical phenomena allow them to exhibit heightened strength-to-weight ratios. Yet, researchers have historically faced challenges with standard lattice shapes, particularly regarding stress concentrations resulting from sharp corners and intersections that lead to material failure. These shortcomings have hindered the broader application of such promising materials.
To tackle these challenges, the research team effectively utilized machine learning as a tool for designing innovative lattice geometries. This strategic decision stemmed from recognizing the potential for machine learning algorithms to analyze vast data sets and identify optimal solutions— a perfect fit for the complexities involved in materials design. The algorithm specifically developed for this study is a multi-objective Bayesian optimization system that can effectively learn from simulated geometries. As a result, it can predict the most effective lattice designs to distribute stress more evenly and enhance overall strength.
In collaboration with international partners from the Korea Advanced Institute of Science and Technology (KAIST), the research leveraged expertise from various disciplines. The collaboration was initiated through the University of Toronto’s International Doctoral Clusters program, which encourages interdisciplinary research among doctoral students and faculty. The KAIST team’s application of the Bayesian optimization algorithm was crucial, enabling the generation of innovative designs while minimizing the volume of data needed for machine learning.
This cutting-edge research employs advanced manufacturing processes, particularly a two-photon polymerization 3D printing technique. This technology is invaluable since it allows researchers to create intricate structures at both the microscale and nanoscale. The team utilized this process to prototype their newly designed nanolattices, which outperform existing materials by exhibiting over twice the strength. Withstanding a stress of 2.03 megapascals per cubic meter per kilogram of density places these materials among the highest-performing options available, even surpassing titanium—a material long considered a standard for strength in weight-sensitive applications.
The potency of this research does not solely lie in its findings; it also reflects the promise of machine learning in optimizing materials science. The machine learning model devised in this study did not merely replicate existing designs; it intelligently assessed and generated entirely novel lattice shapes that achieved remarkable performance improvements. Traditional methods often require extensive data for training machine learning models, but the Bayesian approach utilized in this research was able to function effectively with merely 400 data points, contrasting with the usual requirement of thousands.
The implications of such research extend far beyond the laboratory. Applied to sectors like aerospace, lightweight components derived from the newly designed materials could lead to significant fuel savings—potentially reducing carbon footprints during flight. Researchers estimate that if components made of titanium in aircraft were substituted with their advanced nanolattice materials, it could yield annual savings of up to 80 liters of fuel for each kilogram replaced, underlining the substantial economic and environmental advantages this development could deliver.
The project has drawn contributions from a wide network of experts, including faculty members from the University of Toronto and leading institutions like Karlsruhe Institute of Technology, Massachusetts Institute of Technology, and Rice University. This diverse collaboration combines knowledge from material science, machine learning, chemistry, and mechanics, showcasing the interdisciplinary nature of modern research. The collective expertise fostered throughout this endeavor has bolstered understanding and implementation of advanced material technologies.
As the research team looks to the future, their focus will shift towards scaling up the production of these novel materials, aiming for cost-effective applications at the macroscale. This ambition is central to translating the promising laboratory findings into practical solutions that can be utilized in everyday applications, particularly in the aerospace industry, where material weight and strength will continue to play pivotal roles.
Further exploration is also planned into new designs that prioritize lower densities while maintaining strength and stiffness. As the research evolves, achieving these milestones will transform the landscape of material science, potentially unlocking new applications and innovations across various sectors.
This work undoubtedly sets the stage for a new era of material design and engineering, showcasing the synergies between advanced computational strategies and material science. The potential to redefine how industries think about strength and weight could echo through technological advancements in a broad spectrum of applications.
Researchers anticipate that their pioneering work on machine learning-enhanced nano-architected materials will not only illuminate paths for more efficient designs in aerospace but also inspire future innovations across diverse fields. With a growing demand for sustainable solutions, these advancements may very well contribute positively to mitigating the environmental impacts of modern technologies.
Subject of Research: The intersection of machine learning and nano-architected material design.
Article Title: Engineering Strength: How Machine Learning is Shaping Nano-Architected Materials.
News Publication Date: October 2023.
Web References: University of Toronto Faculty of Applied Science and Engineering.
References: Serles, P., Filleter, T., Ryu, S., Yeo, J., et al. (2023). “Machine Learning-Optimized Nano-Architected Materials.” Advanced Materials. DOI: 10.1002/adma.202410651.
Image Credits: Photos by Peter Serles / University of Toronto Engineering.
Keywords
Machine Learning, Nano-architected Materials, Strength-to-weight Ratio, Aerospace, Additive Manufacturing, Bayesian Optimization, Material Science, Interdisciplinary Research.
Tags: advanced materials engineeringapplications of nanotechnology in industrycarbon steel alternativescustomizable material propertiesinnovative material designlightweight materials for aerospacemachine learning in materials sciencenano-3D printing technologynano-architected materialsProfessor Tobin Filleter researchstrength-to-weight ratio optimizationstructural mechanics in engineering
What's Your Reaction?
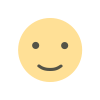
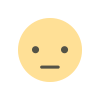
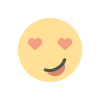
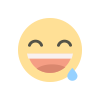
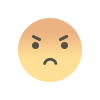
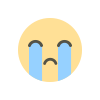
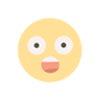