Enhancing Neuromorphic Computing: Paving the Way for Ubiquitous and Efficient AI
Neuromorphic computing has emerged as a transformative field aiming to revolutionize the way we think about computational efficiency and the mimicry of human cognition. By leveraging principles derived from neuroscience, neuromorphic systems are designed to replicate the brain’s architecture and functioning, thereby offering remarkable advancements in processing capabilities. The latest review in Nature highlights the […]

Neuromorphic computing has emerged as a transformative field aiming to revolutionize the way we think about computational efficiency and the mimicry of human cognition. By leveraging principles derived from neuroscience, neuromorphic systems are designed to replicate the brain’s architecture and functioning, thereby offering remarkable advancements in processing capabilities. The latest review in Nature highlights the need for a scalable approach that can keep up with the burgeoning demands of modern computing, particularly in the realm of artificial intelligence and data processing applications.
The core idea behind neuromorphic computing is to create systems that function similarly to neural networks found in the human brain. This involves the innovation of hardware that allows for parallel processing akin to the way neurons communicate and interact within the brain’s dense network. Researchers argue that neuromorphic chips, such as the NeuRRAM chip developed by a team at the University of California San Diego, present a compelling alternative to traditional digital chips by providing enhanced energy efficiency and adaptability without sacrificing accuracy.
In the recent systematic review, researchers delve into the specific architectural advancements necessary to make neuromorphic computing more scalable. This includes optimizing critical features like sparsity—where the system can maintain functional efficiency while minimizing energy consumption through selective neural connection pruning. The authors suggest that mimicking the brain’s selective firing of neurons could yield a new generation of computational devices that not only conserve power but also improve performance across various applications, from artificial intelligence to smart devices.
The implications of scaling neuromorphic computing technology are profound, potentially impacting fields such as healthcare, robotics, and advanced scientific computing. As the electricity demands of traditional AI systems reportedly double by 2026, neuromorphic computing presents an urgent and promising solution to meet the growing resource challenges. The researchers are optimistic that with further collaborations between academia and industry, new applications for neuromorphic systems can be fast-tracked into commercial realities.
Furthermore, the paper underscores that a singular solution may not suffice for every application, which indicates the necessity for an array of neuromorphic devices tailored to different operational needs. Each type of neuromorphic hardware could focus on specific applications, offering a variety of characteristics that can be matched to the desired computational tasks. This modular approach fosters a broad spectrum of innovative solutions to tackle distinct challenges.
The research team also emphasizes the importance of developing user-friendly programming languages and tools to lower the barriers to entry into neuromorphic computing. By encouraging inter-disciplinary collaboration, they aim to foster greater participation across different fields—from neuroscience to computer science—ultimately enriching the neuromorphic ecosystem. The establishment of dedicated research networks, such as THOR: The Neuromorphic Commons, embodies this collaborative vision by providing essential resources and access to neuromorphic computing hardware.
As the pace of innovation accelerates, the need for neuromorphic systems that can handle both the massive scale and energy efficiency reflective of biological learning systems has never been more apparent. The intricate balance of dense and sparse neural connections, inspired by the architecture of the human brain, sets the groundwork for developing future computational models that can self-learn and adapt in real-time environments.
In the coming years, neuromorphic systems are poised to become invaluable tools, offering an essential edge in computing capabilities that can outperform traditional systems on various metrics. The implications for artificial intelligence, where efficiency directly translates into cost savings and environmental impact, cannot be understated. As these technologies evolve, they hold the potential to redefine our relationship with machines, transforming them into collaborative partners rather than mere tools.
An additional focus on optimizing interconnectivity among neuromorphic cores will enhance communication speed and data handling capabilities. High-bandwidth reconfigurable interconnects are key to achieving this goal, allowing for complex interactions among cores that mimic the sophisticated signaling of the brain. This design consideration ensures that neuromorphic systems do not merely replicate brain functionality; they also improve upon it by enabling faster learning and adaptation.
The participation of a diverse group of researchers from various institutions further enriches this discourse. The collaboration highlights the multifaceted approach needed to tackle the challenges of scaling neuromorphic computing—a synthesis of expertise will be vital in pushing these innovations into practical exploitation across industries. This united front marks a significant step forward in establishing neuromorphic computing as not just a theoretical concept but a real-world solution.
Arguably, the development of neuromorphic chips embodies a shift toward a more sustainable form of computing that aligns with global goals for energy efficiency and resource management. As society increasingly integrates advanced technologies, the demand for systems that not only meet performance benchmarks but also minimize ecological footprints will shape the future of computing. Neuromorphic computing is firmly positioned to lead this charge, advocating for a paradigm shift in how we design and utilize computing systems.
The continued exploration and investment into neuromorphic technology herald a new era in computing. With promising frameworks and collaborative efforts in place, researchers envision breakthroughs that might entirely redefine our understanding of artificial intelligence and computational efficiency. The potential for neuromorphic chips to execute complex tasks more efficiently opens the door for innovations that were previously unimaginable, making this field worthy of close attention.
In summary, neuromorphic computing stands at a crucial intersection of neuroscience and computer engineering, poised to redefine technological landscapes. As developments unfold, the future seems ripe with possibilities for scalable, energy-efficient computing that mirrors the brain’s capabilities. With concerted efforts from both academic and industrial sectors, there is a strong likelihood that these technologies will soon transition from research papers to practical applications, making significant impacts across various domains.
Subject of Research: Neuromorphic Computing
Article Title: Neuromorphic Computing at Scale
News Publication Date: 22-Jan-2025
Web References: Nature Article
References: Various research papers referenced within the article.
Image Credits: Credit: David Baillot/University of California San Diego
Keywords
Computational Efficiency, Neuromorphic Systems, Artificial Intelligence, Energy Efficiency, Neural Networks, Sparse Connectivity, Brain Architecture, Interdisciplinary Collaboration, Sustainable Computing, Real-world Applications, High-bandwidth Interconnects, Commercial Applications.
Tags: artificial intelligence scalabilitycognitive computing efficiencyenergy-efficient AI systemsneural network architecture replicationneuromorphic chip innovationsneuromorphic computing advancementsneuroscience-inspired computingNeuRRAM chip technologyparallel processing in AIreducing energy consumption in computingscalable computing solutionstransformative computing technologies
What's Your Reaction?
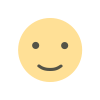
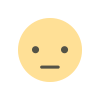
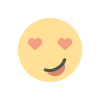
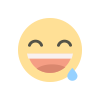
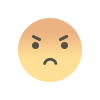
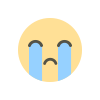
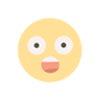