UKB-MDRMF: New Framework for Multi-Disease Risk
In a groundbreaking development that stands to revolutionize the field of personalized medicine, researchers have unveiled the UKB-MDRMF, an innovative multi-disease risk and multimorbidity framework derived from the extensive data of the UK Biobank. This pioneering model offers an unprecedented lens through which the intricate and often overlapping propensities for multiple diseases within individuals can […]

In a groundbreaking development that stands to revolutionize the field of personalized medicine, researchers have unveiled the UKB-MDRMF, an innovative multi-disease risk and multimorbidity framework derived from the extensive data of the UK Biobank. This pioneering model offers an unprecedented lens through which the intricate and often overlapping propensities for multiple diseases within individuals can be understood, predicted, and potentially mitigated. The implications of this research extend far beyond academic curiosity, positioning the medical community to radically enhance predictive healthcare, especially for populations where the interplay of multiple chronic conditions has long complicated diagnostic and therapeutic approaches.
At the heart of this new framework lies the integration of multi-dimensional data harvested from the UK Biobank, one of the largest and most comprehensive biomedical databases worldwide, encompassing genetic, environmental, lifestyle, and clinical information for over 500,000 participants. Unlike traditional risk models that focus on single diseases, UKB-MDRMF leverages complex algorithms to capture the synergistic interactions between co-occurring diseases—what clinicians refer to as multimorbidity. This approach acknowledges that the presence of one condition often influences the risk and progression of others, creating a web of pathological interplay previously challenging to quantify.
The technical core of UKB-MDRMF is a sophisticated machine learning architecture capable of processing and modeling heterogeneous data inputs. By incorporating polygenic risk scores alongside longitudinal phenotypic data, the framework effectively models temporal disease trajectories, capturing not only static risk factors but also dynamic changes over time. This temporal dimension is critical, enabling predictions that reflect how risks evolve as a patient ages or as environmental exposures accumulate. Furthermore, the model’s construction involved rigorous validation steps, including cross-validation within subsamples and external validation in independent cohorts, ensuring robustness and generalizability.
One of the most striking features of this framework is its capability to distinguish between risk factors that are causative in one disease but may be protective or neutral in another, an insight of considerable clinical relevance. For example, certain genetic variants may predispose individuals to cardiovascular disease while simultaneously affording reduced risk for particular cancers, a nuance that UKB-MDRMF can tease apart through its multi-dimensional modeling. Clinicians and researchers can thus gain a more nuanced understanding of personalized risk profiles, enabling tailored preventative strategies that consider the aggregate burden of disease rather than isolated conditions.
The implications of UKB-MDRMF for public health are profound. Aging populations globally face increasing prevalence of multimorbidity, where patients often juggle multiple chronic diseases requiring complex management. Traditional healthcare models, largely siloed by disease category, frequently fall short in addressing the compound risk these patients face. The UKB-MDRMF offers a data-driven foundation upon which integrated care pathways could be designed, optimizing interventions to simultaneously target clusters of diseases and thereby improving patient outcomes while potentially reducing healthcare costs.
Implementation of such complex risk frameworks, naturally, raises challenges in clinical deployment. Translating the predictive outputs of UKB-MDRMF into actionable recommendations requires developing clinician-friendly interfaces and decision-support tools. These tools must not only communicate risk in intuitive terms but also provide evidence-based guidelines for intervention prioritization. The research team behind UKB-MDRMF acknowledges this and plans future collaborations with healthcare providers to co-develop user-centric platforms that seamlessly blend into clinical workflows.
Another critical avenue illuminated by the UKB-MDRMF is the identification of novel multimorbidity patterns and disease clusters previously unappreciated. By sifting through the vast UK Biobank dataset with their advanced algorithms, the researchers have uncovered unique associations between certain neurodegenerative diseases and metabolic syndromes, for example, which may suggest shared pathogenic pathways amenable to targeted therapeutics. Such discoveries open promising research directions, potentially fueling the development of multi-purpose drugs or repurposing existing medications to tackle intertwined disease processes.
The ethical and privacy considerations surrounding the use of large-scale biobank data for constructing predictive models like UKB-MDRMF are also remarkably important. The research team employed rigorous de-identification protocols and maintained compliance with ethical guidelines, ensuring participant confidentiality. Moreover, as personalized medicine tools become more prevalent, safeguarding against discriminatory practices based on predicted disease risk will be paramount. The transparency of UKB-MDRMF’s algorithms and the inclusivity of its training data across diverse demographics are therefore vital factors in promoting equitable healthcare advancements.
From a technical standpoint, the framework’s ability to handle high-dimensional data with substantial missingness is noteworthy. Real-world biomedical datasets are often plagued by incomplete records and varying data quality. UKB-MDRMF utilizes advanced imputation techniques coupled with feature selection methodologies to maintain predictive accuracy despite these imperfections. This resilience bodes well for applying the framework to heterogeneous datasets beyond the UK Biobank, enhancing its utility across different populations and healthcare systems.
The collaborative ethos underlying the development of UKB-MDRMF is also worth emphasizing. The multidisciplinary research team combined expertise in genomics, bioinformatics, clinical medicine, and machine learning to surmount the myriad challenges of modeling multimorbidity. Their integrated approach ensured that the framework is not merely a computational exercise but grounded firmly in biological plausibility and clinical relevance. This model collaboration exemplifies the future of biomedical research, where diverse disciplines converge to unravel complex health puzzles.
Future iterations of UKB-MDRMF are expected to integrate additional data modalities, such as microbiome profiles, metabolomics, and wearable device metrics. Such enhancements promise to capture even richer layers of biological variance and lifestyle factors, refining risk predictions further. The continuous influx of real-world data coupled with advances in artificial intelligence will likely transform these frameworks into highly adaptive systems capable of real-time risk assessment and personalized intervention guidance.
Importantly, UKB-MDRMF also opens new horizons for preventive medicine. By identifying high-risk individuals well before disease onset, healthcare providers can implement early interventions—ranging from lifestyle modifications to pharmacological strategies—that effectively alter disease trajectories. This proactive approach contrasts starkly with reactive treatments and holds the key to reducing the growing burden of chronic multimorbidity in aging societies.
As the framework gains traction, health policy implications are also coming into focus. Integrating such multi-disease risk assessments into national screening programs could optimize resource allocation, prioritize high-risk individuals, and enhance population health outcomes. Policymakers may thus view tools like UKB-MDRMF as invaluable components of precision public health initiatives, balancing individual care with community-wide health strategies.
Finally, the public’s role and perceptions regarding the use of their health data in frameworks like UKB-MDRMF cannot be overstated. Building trust through transparency, clear communication of benefits, and robust data governance will be essential to harness the full potential of biobank-based predictive models. The research team’s commitment to open science and ongoing dialogue with participants underscores a progressive model for ethical biomedical innovation.
In summary, the advent of the UKB-MDRMF framework marks a pivotal milestone in understanding and managing multimorbidity through the lens of big data and machine learning. By capturing the complex interplay of genetic, environmental, and clinical factors across multiple diseases, it promises to transform risk prediction, clinical decision-making, and public health strategies. As this technology matures and finds its way into routine healthcare, it heralds a new era of truly personalized, proactive, and integrative medicine.
Subject of Research: Multi-disease risk prediction and multimorbidity modeling using UK Biobank data.
Article Title: UKB-MDRMF: a multi-disease risk and multimorbidity framework based on UK Biobank data.
Article References:
Jiang, Y., Zhao, B., Wang, X. et al. UKB-MDRMF: a multi-disease risk and multimorbidity framework based on UK biobank data. Nat Commun 16, 3767 (2025). https://doi.org/10.1038/s41467-025-58724-3
Image Credits: AI Generated
Tags: algorithms for multimorbidity assessmentbiomedical research breakthroughschronic condition management strategieschronic disease interaction analysiscomplex disease risk modelinghealthcare data integration techniquesmachine learning in healthcaremulti-disease risk assessmentmultimorbidity prediction frameworkpersonalized medicine advancementspredictive healthcare innovationsUK Biobank data utilization
What's Your Reaction?
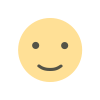
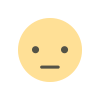
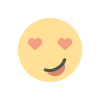
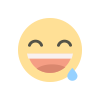
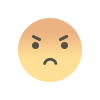
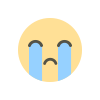
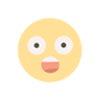