Tsunami Casualty Estimates: Agent-Based vs. Simplified
In the realm of natural disaster preparedness and response, accurately estimating casualties during a tsunami event remains a pivotal challenge. Recent research spearheaded by Takabatake, Hasegawa, Yamaguchi, and colleagues unveiled a comparative analysis that delves deeply into the efficacy of two prominent casualty estimation methodologies. This study scrutinizes the agent-based modeling (ABM) approach alongside more […]

In the realm of natural disaster preparedness and response, accurately estimating casualties during a tsunami event remains a pivotal challenge. Recent research spearheaded by Takabatake, Hasegawa, Yamaguchi, and colleagues unveiled a comparative analysis that delves deeply into the efficacy of two prominent casualty estimation methodologies. This study scrutinizes the agent-based modeling (ABM) approach alongside more traditional, simplified estimation techniques, focusing particularly on their implementation within Japanese coastal urban settings. The findings shed new light on how advances in computational power and behavioral simulation enhance our understanding of tsunami risks and could revolutionize how communities anticipate and mitigate tragic losses.
Tsunamis, by their very nature, strike with immense suddenness and ferocity, often leaving little margin for error in emergency response planning. Historically, casualty estimations in coastal cities have relied on simplified models that use broad assumptions about population vulnerability, evacuation speed, and inundation extents. While these simplified approaches provide quick, often ready-to-use predictions, they fall short in capturing the intricate behaviors and interactions within communities during such crises. The research team highlights that oversimplifications can lead to either underestimates or overestimates, both of which critically impair disaster management.
Agent-based modeling represents a paradigm shift by simulating the actions and interactions of autonomous agents — in this case, individual residents, responders, and environmental variables — to assess their impact on casualty outcomes. Each “agent” is programmed with behavioral rules and decision-making capabilities that mirror real human responses, including evacuation timing, route selection, and social influences. The study meticulously constructed an agent-based framework tailored for Japanese coastal cities, accommodating their unique demographic, geographical, and infrastructural characteristics. This granularity enables a dynamic, nuanced exploration of how tsunamis propagate through complex urban environments and how people react under extreme stress.
The researchers articulate the core strengths of ABM in capturing emergent phenomena, such as traffic congestion during evacuation, bottlenecks in shelter accessibility, and the interplay of social networks in communication of danger. Unlike simplified approaches that apply uniform averages or static assumptions, ABM’s flexibility accommodates heterogeneity in age, mobility, risk perception, and social ties. This heterogeneity proves fundamental in realistically predicting casualty numbers because events unfold not as isolated acts but through collective and individual decisions that can diverge widely.
A key technical facet of the study involved validating the ABM outputs against historical tsunami data and casualty records in several Japanese coastal cities notorious for their vulnerability. The model drew on extensive datasets incorporating topographic mapping, demographic profiles, real-world evacuation drills, and historical event logs. This integrative approach allowed the team to calibrate parameters that govern agent behavior, such as reaction time to warnings, walking speeds, and decision thresholds. Validation exercises confirmed that the ABM notably outperformed simplified casualty estimation models by narrowing confidence intervals and producing casualty estimates closer to recorded fatalities.
One of the most compelling insights from the research concerns how spatial layout influences evacuation efficacy and consequent survival rates. Japanese coastal cities often feature complex urban designs with varying densities, road network patterns, and proximity to hills or elevated shelters. The ABM simulations revealed that subtle differences in urban morphology could cause markedly different evacuation dynamics. For instance, in neighborhoods where roads funnel toward single exit points, congestion surfaced as a critical bottleneck, elevating casualty risks. Conversely, regions with decentralized access routes demonstrated more efficient flux of evacuees, resulting in markedly reduced fatalities. Such findings empower urban planners and disaster mitigation specialists to rethink city design, incorporating evacuation-centric principles to save lives.
Moreover, the team investigated the role of social behavior and communication within the agent networks. In the throes of disaster, misinformation, rumors, and panic can severely hamper response coordination. Through the ABM framework, agents were programmed to exchange information with their neighbors, mimicking realistic social interactions and decision dependencies. It was observed that social cohesion and clear, credible communication chains significantly truncated evacuation times. Conversely, agents isolated from effective information flow exhibited delayed responses and increased risk exposure. This aspect suggests that investment in community engagement and reliable alert systems could yield outsized benefits in casualty reduction.
Contrastingly, the simplified approach examined in the study typically employs deterministic or statistical formulas integrating hazard intensity, population exposure, and vulnerability indices without representing behavioral variability or spatial-temporal dynamics. While expedient and less computationally demanding, these methods lack the sensitivity required to capture complex human-environment interactions. The research explicitly demonstrated scenarios where simplified models systematically underestimated casualties, especially when unpredictable social behaviors or infrastructural impediments emerged. Such underestimations could jeopardize allocation of emergency resources and public safety interventions.
The comparative analysis also touches upon computational resources and usability challenges. Although ABM offers superior accuracy and detail, it demands extensive data inputs and substantial computational power. The study discusses how advances in cloud computing, GIS integration, and machine learning techniques mitigate these constraints, enabling practical implementations at city-wide scales. Nonetheless, the authors emphasize that simplified approaches still hold utility as initial screening tools or in resource-limited contexts, suggesting a tiered or hybrid modeling framework for comprehensive disaster planning.
Beyond technological and methodological evaluation, the implications of this study resonate deeply at the policy level. Enhanced casualty estimation models enable authorities to develop sharper evacuation guidelines, optimize shelter placements, and tailor public education campaigns. For example, precise identification of high-risk zones combined with simulated evacuation routes can inform targeted infrastructure investments such as additional pedestrian bridges or community alert systems. Furthermore, the ABM’s capability to simulate evacuation drills offers a powerful platform for emergency preparedness training, allowing scenarios to be tested and refined virtually before disasters strike.
Importantly, the research underscores the value of multidisciplinary collaboration. Disaster risk science intertwines geophysics, urban engineering, social psychology, and computer science. The team’s composition, featuring experts across these domains, facilitated a holistic modeling approach that transcends siloed perspectives. Such integrative efforts prove essential as natural hazards escalate in frequency and intensity under climate change realities, demanding nuanced tools that reflect the multifaceted nature of human vulnerability and resilience.
The timing of this study is particularly significant for Japan, a nation frequently battered by seismic and tsunami events. The 2011 Tohoku earthquake and tsunami dramatically illustrated the devastating human toll these catastrophes can inflict, exposing limitations in crisis response and risk estimation methods. The research by Takabatake and colleagues represents a critical step forward in refining predictive capabilities, ultimately contributing to safer, more resilient coastal communities. Japan’s experience serves as a global benchmark, and the insights garnered here are adaptable to other tsunami-prone regions worldwide.
Technologically, the agent-based models crafted for this study integrate Geographic Information Systems (GIS) data, demographic surveys, and real-time hazard projections. By layering spatial data with behavioral algorithms, the models generate dynamic casualty heatmaps evolving over time during tsunami scenarios. These maps pinpoint vulnerable clusters and evacuation chokepoints, providing actionable intelligence to stakeholders. The approach exemplifies the fusion of big data analytics with social simulation to tackle intricate disaster challenges.
The study also explores sensitivity analyses within the agent-based framework, examining how variations in key parameters affect outcomes. Parameters such as agent reaction delays, mobility impairments, and social influence weights were systematically altered to assess model robustness and identify critical leverage points. Findings reveal that even modest improvements in early warning dissemination or mobility assistance can produce substantial casualty reductions. This highlights the potential for targeted interventions grounded in model insights to save hundreds or thousands of lives.
Looking ahead, the authors advocate for integration of real-time sensor networks and mobile data streams into ABM platforms. Such integration could enable adaptive simulations that respond dynamically to unfolding events, providing emergency managers with live casualty forecasts and evacuation status metrics. The vision is to transition from static planning tools to responsive decision-support systems powered by artificial intelligence and Internet-of-Things connectivity, heralding a new era in disaster risk management.
In conclusion, the comparative analysis by Takabatake et al. champions agent-based modeling as a transformative advance in tsunami casualty estimation. By embracing the complexity of human behavior, spatial heterogeneity, and social networks, ABMs offer a powerful alternative to traditional simplified models. Though computationally intensive, their increased accuracy and granularity equip policymakers and practitioners with the insights needed to design more effective evacuation strategies and safeguard lives. As natural hazards loom larger globally, investments in such sophisticated modeling frameworks will be indispensable in building resilient societies.
Subject of Research: Tsunami casualty estimation methodologies and their comparative effectiveness in Japanese coastal cities.
Article Title: Comparative Analysis of Tsunami Casualty Estimation Approaches: Agent-Based Modeling versus Simplified Approach in Japanese Coastal Cities
Article References:
Takabatake, T., Hasegawa, N., Yamaguchi, K. et al. Comparative Analysis of Tsunami Casualty Estimation Approaches: Agent-Based Modeling versus Simplified Approach in Japanese Coastal Cities. Int J Disaster Risk Sci 15, 719–737 (2024). https://doi.org/10.1007/s13753-024-00586-2
Image Credits: AI Generated
Tags: advances in disaster response technologyagent-based modeling for disaster responsebehavioral simulation in natural disasterscoastal city tsunami preparednesscommunity interactions during tsunamiscomputational power in emergency planningdisaster management strategies for tsunamisenhancing tsunami risk understandinginaccuracies in casualty estimationJapanese coastal urban tsunami studiessimplified models in tsunami predictionstsunami casualty estimation methodologies
What's Your Reaction?
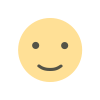
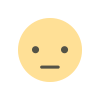
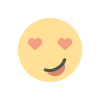
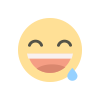
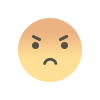
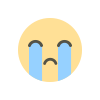
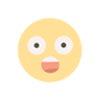