CNN-Enhanced Model Accelerates Urban Flood Prediction
In the rapidly urbanizing landscapes of the 21st century, effective prediction of urban pluvial flooding—a phenomenon increasingly aggravated by climate change and impervious city surfaces—has become a critical challenge. Recent advancements from a research team led by Yang, J., Liu, K., and Wang, M. introduce a groundbreaking model that harnesses the power of deep learning […]

In the rapidly urbanizing landscapes of the 21st century, effective prediction of urban pluvial flooding—a phenomenon increasingly aggravated by climate change and impervious city surfaces—has become a critical challenge. Recent advancements from a research team led by Yang, J., Liu, K., and Wang, M. introduce a groundbreaking model that harnesses the power of deep learning integrated with cellular automata to forecast these complex flood dynamics with unprecedented speed and accuracy. Their study, titled “A Convolutional Neural Network-Weighted Cellular Automaton Model for the Fast Prediction of Urban Pluvial Flooding Processes,” published in the International Journal of Disaster Risk Science, represents a promising leap forward in disaster risk management and urban hydrology.
Urban pluvial flooding occurs when intense rainfall overwhelms drainage systems, causing surface water accumulation that can disrupt city infrastructure and endanger millions. Traditional hydrodynamic models, though accurate, often require intensive computational resources and time, limiting their utility for real-time disaster response. To overcome these limitations, the team devised a hybrid modeling framework that synergizes convolutional neural networks (CNNs)—a class of deep learning algorithms known for extracting spatial features from complex data—and cellular automata (CA), which simulate the spatially distributed evolution of flood dynamics over discrete time steps.
The heart of this innovation lies in coupling the data-driven capacities of CNNs with the spatially explicit and rule-based characteristics of cellular automata. Unlike conventional models that rely purely on physical parameters and extensive simulations, this approach applies CNNs to dynamically weight the transition rules governing the CA, effectively learning and adapting to the nuanced patterns of water flow in urban settings. This dynamic weighting enables the CA to model the flooding process more realistically and accurately, accounting for the heterogeneous nature of urban terrain, drainage networks, and rainfall distribution.
Importantly, the model was trained and validated using high-resolution datasets obtained from a metropolitan area prone to pluvial flooding events. This dataset included digital elevation models, land use maps, rainfall time series, and historical flood records. By integrating these heterogeneous data sources, the CNN component could discern key hydrological features that govern flood propagation while the CA component efficiently simulated the temporal evolution of the floodwaters across the urban terrain. This hybridization results in a robust predictive tool capable of considerably reducing computational costs compared to traditional numerical simulations.
The researchers highlight that the CNN-weighted CA model exhibits remarkable speed, achieving real-time or near-real-time forecasting capabilities, a feature critical for emergency management and urban planning agencies. Such rapid predictions allow for timely warnings and the implementation of flood mitigation strategies such as dynamic traffic rerouting, emergency evacuations, and water diversion measures. The scalability of the model also means it can be adapted to cities worldwide, provided that sufficient local data is available for training.
Another core advancement offered by this research is the model’s resilience to data gaps and uncertainties often encountered in urban hydrological data collection. Traditional hydraulic models typically require continuous, high-quality input data, but the neural network training phase endows the system with the ability to generalize from incomplete or noisy data, maintaining reliable prediction performance. This adaptability is a game-changer in disaster-prone urban environments where sensor failures, communication breakdowns, or unexpected meteorological events may hamper data availability.
Moreover, the model’s modular design enables seamless integration with other urban management platforms. By serving as the forecasting engine, the CNN-weighted CA model can feed predictions into geographic information systems (GIS), smart city dashboards, and decision support tools, empowering stakeholders with actionable, spatially explicit flood risk assessments. This integrated approach paves the way for more responsive, data-driven urban resilience frameworks that leverage both cutting-edge artificial intelligence and established hydrological modeling methods.
The study’s extensive validation experiments demonstrated that predictions generated by the CNN-weighted CA model align closely with observed flooding extent and depth metrics from past flood events. The model outperformed conventional hydrodynamic solvers in both computational efficiency and predictive accuracy, particularly in complex urban microtopographies where traditional methods struggle. Its ability to represent localized pooling effects, flow paths through urban canyons, and rapid shifts in flood extents under varying rainfall intensities marks a significant advance in urban flood science.
Beyond emergency forecasting, such a model provides urban planners and engineers with a powerful tool to evaluate the impacts of land use changes, drainage system upgrades, and climate adaptation measures. By simulating different scenarios, the CNN-weighted CA system can inform infrastructure investments and regulatory policies aimed at reducing flood vulnerability and enhancing the sustainability of urban environments. This capability represents a crucial intersection between scientific innovation and practical urban governance.
This pioneering research also opens new avenues for interdisciplinary collaboration, merging expertise from hydrology, computer science, urban studies, and environmental engineering. The successful development and deployment of such a hybrid model demonstrate the transformative potential of artificial intelligence techniques when creatively applied to long-standing environmental challenges. As urban centers continue to grapple with the consequences of extreme weather, models like this one will be indispensable in shaping resilient and adaptive cities.
Looking ahead, the research team suggests several directions for future work, including extending the modeling framework to incorporate subsurface water flow, sediment transport, and pollutant dispersion during flood events. They also aim to improve the model’s interpretability, providing end-users with clearer insights into how specific urban features and rainfall inputs influence flood outcomes. Such transparency is essential for fostering trust and facilitating the adoption of AI-driven models within policy and operational contexts.
The algorithm’s design also lends itself to continuous updating as new data becomes available, making it suitable for learning and evolving in response to changing urban environments and climatic conditions. This dynamic learning aspect promises a long-term, sustainable approach to urban flood risk management, where predictive models improve incrementally based on real-world feedback and monitoring data streams.
In essence, the CNN-weighted cellular automaton model represents a paradigm shift in urban flood forecasting, demonstrating how artificial intelligence can revolutionize environmental hazard prediction. Its blend of speed, accuracy, and adaptability equips cities with vital knowledge to safeguard lives, property, and economic vitality against the mounting threat of pluvial floods. By blending physics-based modeling traditions with machine learning advances, Yang, Liu, Wang, and their colleagues offer a promising blueprint for the urban resilience challenges of the future.
As cities worldwide confront escalating flood risks due to climate change-induced shifts in precipitation patterns and urban expansion, tools like this new model will become ever more critical. The research not only advances scientific understanding but also sets a foundation for more informed urban planning and disaster preparedness. By facilitating rapid, actionable predictions, the CNN-weighted CA approach could save lives, reduce economic losses, and enable smarter urban development in the decades to come.
Ultimately, this study embodies the convergence of technology and society, illustrating how innovative computational approaches can transform how communities anticipate and respond to natural disasters. The model underscores the importance of interdisciplinary research and offers a powerful example of AI-driven science serving the public good. As cities grow and climate hazards intensify, such advanced forecasting tools may become the bedrock of 21st-century urban resilience strategies.
Subject of Research: Urban pluvial flooding prediction using hybrid AI and cellular automaton modeling.
Article Title: A Convolutional Neural Network-Weighted Cellular Automaton Model for the Fast Prediction of Urban Pluvial Flooding Processes.
Article References: Yang, J., Liu, K., Wang, M. et al. A Convolutional Neural Network-Weighted Cellular Automaton Model for the Fast Prediction of Urban Pluvial Flooding Processes. Int J Disaster Risk Sci 15, 754–768 (2024). https://doi.org/10.1007/s13753-024-00592-4
Image Credits: AI Generated
Tags: cellular automata for flood forecastingclimate change and urban floodingCNN-enhanced flood modelcomputational efficiency in flood modelingdeep learning in hydrologydisaster risk science advancementshybrid modeling frameworksreal-time flood risk managementsurface water accumulation challengesurban flood predictionurban infrastructure resilienceurban pluvial flooding
What's Your Reaction?
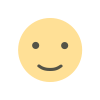
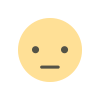
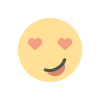
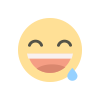
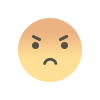
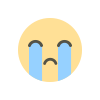
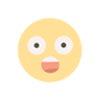