St. Jude Researchers Develop Scalable Method for Analyzing Single-Cell Data
Researchers are continuously striving to unravel the complexities of human biology, and recent advancements have brought them closer to this goal. A team at St. Jude Children’s Research Hospital has developed a transformative machine-learning algorithm designed specifically for analyzing vast single-cell gene expression datasets. The accuracy and efficiency of this new method address longstanding challenges […]

Researchers are continuously striving to unravel the complexities of human biology, and recent advancements have brought them closer to this goal. A team at St. Jude Children’s Research Hospital has developed a transformative machine-learning algorithm designed specifically for analyzing vast single-cell gene expression datasets. The accuracy and efficiency of this new method address longstanding challenges in the field, making it a significant contribution to computational biology. The algorithm, known as Consensus and Scalable Inference of Gene Expression Programs (CSI-GEP), sets new benchmarks for data analysis in an era where large datasets are becoming the norm.
The landscape of gene expression analysis has been revolutionized by the advent of single-cell sequencing technology. Traditionally, bulk gene expression data provided insights that were often too generalized, failing to capture the nuances at the cellular level. In contrast, single-cell analysis allows scientists to examine the behaviors and characteristics of individual cells. This level of detail is comparable to scrutinizing a single kernel of corn within an expansive field, providing researchers with the ability to identify distinct cellular processes and their roles in various diseases.
Despite the promise of single-cell technologies, researchers have faced significant hurdles in data analysis. With the explosion of cellular data, conventional methods have often struggled to keep pace, leading to analyses that are not only biased but also contradictory. By leveraging machine learning, the St. Jude team aims to streamline this analysis, allowing for deeper insights without the pitfalls associated with standard techniques. Their new algorithm is capable of efficiently processing the large volumes of data generated in single-cell studies, serving as a beacon of hope for researchers grappling with computational limitations.
The chief architect behind the CSI-GEP algorithm is Paul Geeleher, PhD, from the Department of Computational Biology at St. Jude. He emphasizes the tool’s capability to scale alongside growing datasets. The significance of this cannot be overstated; as the amount of single-cell RNA sequencing data grows, so too does the computational power required to analyze it effectively. With traditional methods, researchers often had to make trade-offs that led to inaccurate interpretations of the data. CSI-GEP, on the other hand, is designed to restore accurate analysis to a manageable timeframe, a feat that could vastly improve the outcomes of single-cell studies.
Central to the success of CSI-GEP is its innovative use of graphics processing units (GPUs). This unconventional approach allows the algorithm to harness the power typically reserved for high-performance computing tasks, enabling it to handle the massive datasets that characterize single-cell sequencing. GPUs excel at parallel processing, making them an ideal choice for the computational challenges posed by this type of analysis. By integrating GPUs into their workflow, the researchers have managed to enhance both the speed and efficiency of their data processing capabilities.
One of the core features of the CSI-GEP algorithm is its reliance on unsupervised machine learning principles. Traditional analysis methods often introduce biases, as they require researchers to pre-select parameters and make subjective choices about how to group cells. In contrast, the CSI-GEP algorithm operates without the need for oversight, autonomously deriving the best analysis parameters directly from the data itself. This methodology not only minimizes arbitrary decision-making but also enhances the robustness of the findings generated by the analysis.
The CSI-GEP algorithm has demonstrated its efficacy across various substantial single-cell RNA sequencing datasets. In trials, this innovative tool outperformed existing methodologies, revealing previously hidden cell types and biological processes. The success of CSI-GEP is grounded in its capacity to adapt and learn from the datasets it encounters, positioning it as a versatile tool applicable to a multitude of research areas. This flexibility could significantly advance our understanding of a wide array of diseases and conditions through more precise cellular investigations.
The collaborative nature of the research has resulted in a diverse author group, including notable contributors such as Xueying Liu, PhD, and several others from St. Jude and the University of Tennessee Health Science Center. Together, they have combined their expertise to develop a tool that is not only technically proficient but also crucial for scientific inquiry. Their work emphasizes the importance of interdisciplinary collaboration in tackling complex biological questions, particularly as the demands of single-cell research continue to evolve.
Supported by significant grants from various institutions, including the National Cancer Institute and the National Human Genome Research Institute, the CSI-GEP algorithm represents a culmination of dedicated research efforts aiming to push the boundaries of computational biology. The funding underscores the importance of advancements in this domain, particularly as researchers seek to translate these tools into actionable insights for medical research and treatment protocols.
The implications of such advancements are profound, particularly in the oncology domain where understanding the underlying cellular behaviors can lead to groundbreaking treatment strategies. St. Jude Children’s Research Hospital, recognized as a leader in pediatric research, plays a pivotal role in translating these scientific discoveries into practical applications. The hospital’s ongoing commitment to sharing their findings with the broader scientific community ensures that other researchers can benefit from these developments, ultimately enhancing global health outcomes.
As the demand for advanced analytical tools continues to rise in the field of gene expression, it is likely that the CSI-GEP algorithm will change the way researchers approach single-cell studies. The commitment to open access further democratizes this knowledge, allowing scientists around the world to utilize and build upon their work. This accessibility fosters an environment of collaboration and innovation, crucial for advancing medical science.
In summary, the introduction of the CSI-GEP algorithm represents a significant milestone in the study of single-cell gene expression. Its ability to provide accurate, scalable, and unbiased analyses of vast datasets positions it as a transformative tool in computational biology. This breakthrough not only enhances our understanding of cellular mechanisms but also sets the stage for future discoveries in disease research and treatment, paving the way for improved health outcomes.
Subject of Research: Single-Cell RNA Sequencing Analysis
Article Title: CSI-GEP: A GPU-based unsupervised machine learning approach for recovering gene expression programs in atlas-scale single-cell RNA-seq data
News Publication Date: 8-Jan-2025
Web References: https://github.com/geeleherlab/CSI-GEP
References: 10.1016/j.xgen.2024.100739
Image Credits: Credit: St. Jude Children’s Research Hospital
Keywords: Computational biology, Gene expression, RNA sequencing
What's Your Reaction?
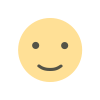
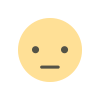
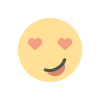
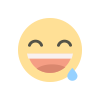
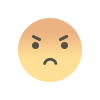
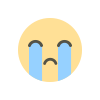
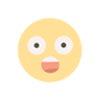