Revolutionary AI from Stanford Medicine Enhances Cancer Prognosis Predictions and Treatment Response Insights
Recent advancements in artificial intelligence (AI) have presented groundbreaking opportunities in the field of oncology, particularly regarding the prognostic capabilities of models utilized in cancer care. Traditionally, clinicians have relied heavily on various types of data, including diagnostic imaging and clinical reports, to make informed decisions regarding patient treatment. However, a novel approach has emerged […]

Recent advancements in artificial intelligence (AI) have presented groundbreaking opportunities in the field of oncology, particularly regarding the prognostic capabilities of models utilized in cancer care. Traditionally, clinicians have relied heavily on various types of data, including diagnostic imaging and clinical reports, to make informed decisions regarding patient treatment. However, a novel approach has emerged from Stanford Medicine, involving a comprehensive AI model capable of integrating both visual and textual data into a cohesive predictive framework. This significant leap enables a level of precision previously unattainable, enhancing prognostic assessments and refining therapeutic decision-making for patients battling different forms of cancer.
The newly developed AI model, designated as MUSK, stands out in its ability to analyze an extensive array of data types simultaneously. Faced with the complexity of cancer care, researchers have sought to develop a model that transcends the limitations of traditional methods, which typically rely on either imaging or textual data in isolation. By examining over 50 million medical images alongside more than 1 billion pieces of pathology-related text, MUSK is engineered to recognize patterns and produce predictions about cancer prognosis with remarkable accuracy. This duality of data not only enriches the learning process but also facilitates a more nuanced understanding of individual patient profiles.
One of the notable strengths of MUSK lies in its training methodology. Unlike existing models that often depend on curated datasets with labeled and paired information—such as annotated images alongside corresponding clinical notes—MUSK can utilize unpaired multimodal data for its initial training. By doing so, researchers have dramatically expanded the volume of data available for the model’s learning phase, effectively allowing it to build a foundational understanding without the constraints of conventional pairing requirements. This shift heralds a new era in AI development, where the focus can shift from curating extensive labeled datasets to leveraging the abundant, unlabelled data available in clinical settings.
The implications of this model extend beyond mere predictive accuracy; it promises to transform the clinical decision-making landscape in oncology. As Ruijiang Li, the lead researcher at Stanford Medicine, points out, the pressing need within clinical practice is for tools that accurately guide treatment strategies for patients. By integrating a diverse array of data inputs—ranging from histopathological slides to patient demographics and treatment histories—MUSK can offer tailored therapeutic recommendations that resonate with the complexities of individual cases.
Various studies conducted using MUSK have shown promising results. In evaluations concerning disease-specific survival across multiple cancer types, the model accurately predicted outcomes with a success rate of 75%. In stark contrast, traditional prediction methods that rely solely on established clinical risk factors achieved a significantly lower accuracy of 64%. This disparity emphasizes the potential of AI models like MUSK to refine prognostic capabilities and enhance clinical outcomes for cancer patients at various stages of their disease.
Moreover, MUSK’s capabilities extend to determining which patients might benefit most from specific treatments, such as immunotherapy. By analyzing expansive datasets that incorporate various clinical elements, the model can ascertain responses to therapies with a higher degree of certainty. Notably, in the realm of non-small cell lung cancer, MUSK identified patients likely to benefit from immunotherapy with an impressive accuracy rate of 77%, while conventional methods based on singular biomarkers, like PD-L1 expression, returned a mere 61% accuracy.
In addressing melanoma, researchers again witnessed MUSK’s capabilities shine, as the model effectively identified patients at heightened risk for cancer recurrence within a five-year span following their initial treatment. The accuracy of 83% achieved in this scenario surpasses predictions made by other foundation models—further solidifying MUSK as a formidable tool in the arsenal for oncologists seeking to optimize patient care.
The uniqueness of MUSK is further accentuated by its capacity to integrate unpaired multimodal data into its pretraining process. This innovation significantly amplifies the scale of data leveraged compared to competing models reliant on more traditional methods. The consistent demonstration of superior predictive power when employing multifaceted data underscores the critical role of such AI advancements in refining patient care strategies.
As the adoption of AI in clinical practice continues to accelerate, MUSK stands at the forefront of this evolution. The capacity to process and analyze complex data landscapes will undoubtedly impact how clinicians approach therapeutic decisions moving forward. With promising statistics highlighting the model’s predictive accuracy, healthcare providers have compelling evidence to support the integration of AI tools within oncology.
The collaborative effort involving researchers from both Stanford Medicine and Harvard Medical School signals a broader commitment to advancing the capabilities of AI in medicine. By harnessing extensive federal funding through grants from the National Institutes of Health, significant resources have been allocated to push the boundaries of what is possible with AI technologies in cancer care, paving the way for improved patient outcomes on a large scale.
In conclusion, Stanford’s introduction of MUSK represents a compelling advancement in the domain of precision medicine, particularly in oncology. The ability to meld diverse data types into one coherent framework not only enhances predictive accuracy but also enriches the entire clinical decision-making process. As more clinicians begin to recognize the potential benefits of AI models like MUSK, the future of cancer treatment looks increasingly promising, characterized by improved prognostics, tailored therapies, and ultimately, more favorable patient outcomes.
Subject of Research: Human tissue samples
Article Title: A vision–language foundation model for precision oncology
News Publication Date: 8-Jan-2025
Web References: Stanford Medicine News
References: Nature Journal
Image Credits: Stanford Medicine
Keywords: Artificial Intelligence, Cancer Research, Precision Oncology, Prognostic Models, Multimodal Data.
What's Your Reaction?
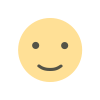
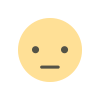
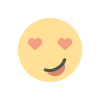
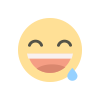
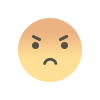
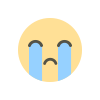
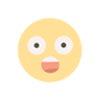