Researchers Introduce AI to ‘Kindergarten’ Concepts to Enhance Its Learning of Complex Tasks
In a recent groundbreaking study published in the prestigious journal Nature Machine Intelligence, a research team from New York University has unveiled a novel strategy for training artificial intelligence systems that mirrors the learning pathways of human cognition. This innovative approach, dubbed “kindergarten curriculum learning,” sheds light on how foundational skills can be developed and […]

In a recent groundbreaking study published in the prestigious journal Nature Machine Intelligence, a research team from New York University has unveiled a novel strategy for training artificial intelligence systems that mirrors the learning pathways of human cognition. This innovative approach, dubbed “kindergarten curriculum learning,” sheds light on how foundational skills can be developed and refined before tackling more complex tasks. The researchers, led by Cristina Savin, an associate professor in NYU’s Center for Neural Science and Center for Data Science, drew parallels between early childhood education and AI training, demonstrating that these sequential learning stages can significantly enhance the performance of recurrent neural networks (RNNs).
At the core of this study lies the observation that much like children must first grasp the basics of letters and numbers to advance to reading and mathematics, AI models can similarly benefit from a structured learning process. RNNs are specifically designed to handle sequential data and are extensively applied in areas such as speech recognition and language translation. However, traditional training methods have struggled to replicate the nuanced learning patterns observed in humans and animals, particularly when it comes to executing complex cognitive tasks. The research team embarked on a series of experiments to explore how instilling a clear understanding of basic tasks can lead to improved performance on intricate problems.
The researchers began their exploration with a series of laboratory experiments using rats. In a controlled setting, the rats were trained to locate a hidden water source using a box with several compartmentalized ports. This setup required the rodents to develop an understanding that specific sounds and illuminated lights were associated with the availability of water, and that they could not immediately rush toward the source after these cues. The results of these experiments illustrated that the rats successfully combined knowledge of these fundamental principles to refine their behavior and achieve their goal of water retrieval, thus emulating a form of cognitive learning process.
Translating these findings into the realm of artificial intelligence, the NYU team applied the same principles to train RNNs. Instead of focusing on basic water retrieval tasks, the networks were challenged to manage a wagering task that relied on building decision-making skills over time. The researchers structured the training so that the RNNs progressed through simple tasks and gradually advanced to more complex ones. This kindergarten curriculum learning model was then meticulously compared against existing RNN-training methodologies, shedding light on its potential to enhance AI learning capabilities.
The results were compelling. The RNNs trained via the kindergarten curriculum model demonstrated significantly faster learning rates than their counterparts subjected to conventional training techniques. This marked a notable advance in the field of artificial intelligence, suggesting not only the efficacy of systematic learning in neural networks but also a direction for future research aimed at improving AI systems. The findings support the premise that a structured approach—akin to early educational stages for children—could foster better learning outcomes as artificial intelligence continues to evolve.
The implications of this research extend beyond the confines of animal behavior and AI training. By understanding how basic skills can be layered to address complex challenges, researchers can pave the way for more sophisticated AI systems capable of mimicking human cognitive functions more closely. This approach also underscores the importance of considering prior knowledge and experiences when designing training frameworks for AI, potentially leading to tools that can learn and adapt in ways akin to human learning experiences.
As the researchers continue to analyze and refine their methods, their findings emphasize a shift in perspective regarding AI development. The incorporation of learning frameworks that reflect the natural learning progression seen in humans may signal a new era in artificial intelligence training, prompting other scientists to explore similar paths. The evidence supports a broader inquiry into how foundational training can affect not only the efficiency and speed of learning, but also the overall capability of AI systems to solve real-world problems.
Ultimately, this innovative research sets the stage for future explorations aimed at understanding the intricate interplay between learning, knowledge storage, and complex task performance in artificial intelligence. As advancements in AI continue to unfold, applying lessons learned from our understanding of cognitive development becomes increasingly vital. By embracing strategies such as kindergarten curriculum learning, researchers can enhance the cognitive capacities of AI systems, potentially leading to more intuitive and capable machines in the foreseeable future.
The quest to develop increasingly intelligent AI is one of the most pressing areas of contemporary research. As methods evolve and new strategies emerge, the NYU team’s work illustrates a promising path forward. By digging into the depths of learning methodologies, we can unlock the potential of AI systems to not only perform basic tasks but to also engage in more complex, humanlike behaviors. This realignment of AI training processes to correlate with cognitive learning in animals and humans may very well transform how we approach the future of artificial intelligence.
As researchers and developers forge ahead, the insights gleaned from this study may serve as foundational elements in the creation of robust, adaptable AI systems. With a clearer understanding of how basic skills can be taught and combined to achieve more significant outcomes, the sky is the limit for future applications. Interdisciplinary collaboration will be essential in this endeavor, as insights from cognitive science and neuroscience continue to inform the development and progression of artificial intelligence.
In conclusion, the NYU study marks a significant milestone in AI research, shedding light on effective training strategies that mirror human cognitive development. By fostering a deeper understanding of how foundational skills can facilitate complex behaviors, this research lays the groundwork for more sophisticated and capable AI systems, pushing the boundaries of what artificial intelligence can achieve and enhancing its integration into everyday life. As we continue to explore this intersection of technology and cognitive science, we may find solutions to some of the most challenging problems facing our society today, ultimately leading us to a future where AI can function not only as tools but as collaborative partners in human endeavors.
Subject of Research: Animals
Article Title: Compositional pretraining improves computational efficiency and matches animal behaviour on complex tasks
News Publication Date: 19-May-2025
Web References: http://dx.doi.org/10.1038/s42256-025-01029-3
References: N/A
Image Credits: N/A
Keywords
Tags: AI learning strategiesartificial intelligence developmentcognitive task executioncomplex task performanceearly childhood education in AIfoundational skills in AIhuman cognition parallelsinnovative AI training methodskindergarten curriculum learningNYU research on AIrecurrent neural networks trainingsequential learning stages
What's Your Reaction?
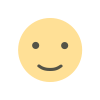
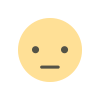
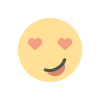
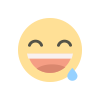
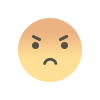
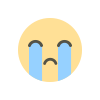
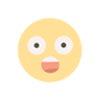