OpenAI Deep Research Tool Revolutionizes Literature Reviews
In an era defined by the relentless march of technological progress and the growing integration of artificial intelligence into every facet of scientific inquiry, the recent unveiling of OpenAI’s pay-for-access “deep research” tool marks a significant milestone in the evolution of academic research methodologies. Announced on 06 February 2025, this innovative tool is designed to […]

In an era defined by the relentless march of technological progress and the growing integration of artificial intelligence into every facet of scientific inquiry, the recent unveiling of OpenAI’s pay-for-access “deep research” tool marks a significant milestone in the evolution of academic research methodologies. Announced on 06 February 2025, this innovative tool is designed to synthesize information from a multitude of online sources into comprehensive, cited reports that span several pages—a function that promises to revolutionize the way literature reviews and full review papers are generated. By leveraging the improved reasoning capabilities of the o3 large language model (LLM) in conjunction with real-time internet search functionalities, the tool can accomplish in mere minutes what traditionally has taken researchers hours or even days to compile. Such an achievement is both striking and transformative, capturing the attention of scientists, data experts, and technology enthusiasts who are eager to explore its potential applications in advancing academic research and facilitating the rapid assimilation of knowledge.
The advent of this tool comes at a time when the pressure on researchers to stay abreast of the rapidly expanding body of scientific literature is greater than ever. Traditional methods of conducting literature reviews are increasingly seen as cumbersome and time-consuming, with the sheer volume of information available across countless databases and websites rendering manual synthesis a formidable challenge. OpenAI’s deep research tool, therefore, emerges as a timely solution to these challenges by offering the possibility of generating thorough, cited reports that not only summarize existing research but also identify potential gaps in knowledge. In doing so, it promises to act as a highly efficient personal research assistant—one capable of processing and organizing vast amounts of information with a level of speed and precision that is unprecedented in the academic sphere.
Early adopters of the tool have reported a mixture of enthusiasm and cautious optimism. Many scientists, including those who have extensive experience with both conventional research methodologies and cutting-edge AI technologies, have expressed admiration for the tool’s ability to produce coherent and well-cited literature reviews that could potentially streamline the research process. For instance, Derya Unutmaz, an immunologist at the Jackson Laboratory in Farmington, Connecticut, who has been granted complimentary access to ChatGPT Pro for her medical research, described the reports generated by OpenAI’s deep research tool as “extremely impressive” and “trustworthy.” Unutmaz went further to assert that the quality of these AI-generated documents is comparable to, if not superior to, that of published review papers, suggesting that the traditional practice of writing reviews may soon become obsolete. Such high praise is tempered, however, by a recognition of the tool’s limitations. Like all LLM-based systems, it is not immune to inaccuracies; the tool occasionally produces erroneous citations, hallucinates facts, and struggles to distinguish between authoritative sources and unverified information. OpenAI itself acknowledges that the tool is in its early stages and that its current shortcomings—such as the occasional misattribution of sources and the inability to precisely convey uncertainty—are expected to improve over time as the technology matures and accumulates usage data.
The tool’s launch is part of a broader trend in which major technology firms are investing heavily in the development of AI agents that can undertake complex, multi-step tasks traditionally performed by human experts. Google, for example, released its own Deep Research tool in December, which similarly integrates search capabilities with advanced reasoning to produce synthesized reports. While both tools share the common goal of accelerating the research process, differences in their underlying architectures have already begun to emerge. Google’s tool is currently based on the Gemini 1.5 Pro model, whereas OpenAI’s offering builds upon the enhanced reasoning prowess of its o3 LLM. Proponents of the OpenAI system argue that this enhanced reasoning capability lends the tool an added layer of sophistication, enabling it to not only aggregate data from numerous sources but also to perform a level of critical analysis that is more aligned with the cognitive processes of human researchers.
One of the most compelling aspects of OpenAI’s deep research tool is its performance on challenging benchmark tests that assess its reasoning and information synthesis capabilities. For example, when subjected to Humanity’s Last Exam (HLE)—a rigorous 3,000-question benchmark designed to evaluate expert-level knowledge across a range of disciplines—the tool achieved a score of 26.6% on text-only questions, positioning it at the top of the leaderboard for such evaluations. In addition, when measured against the GAIA benchmark—a test specifically developed to assess AI systems that utilize multi-step reasoning and real-time web browsing—the tool scored an impressive 58.03%, outperforming competing systems that rely on alternative models. These benchmark results are significant not only because they underscore the tool’s technical prowess, but also because they suggest that the integration of advanced reasoning with dynamic web search capabilities can yield outputs that are both comprehensive and contextually relevant.
Despite these achievements, the tool is not without its detractors. Some researchers have voiced concerns regarding the extent to which the tool’s outputs can be relied upon for academic research, given the inherent challenges associated with ensuring the accuracy of automatically generated citations and the potential for the tool to misrepresent complex scientific concepts. Kyle Kabasares, a data scientist at the Bay Area Environmental Research Institute in Moffett Field, California, expressed a measure of skepticism in an online video review, noting that if a human produced the same report, it would require substantial revision and refinement. Such critiques highlight a broader tension within the scientific community: the balance between embracing innovative AI solutions that promise to enhance productivity and maintaining rigorous standards of accuracy and reliability that are the hallmark of scholarly research.
The debate over the utility of AI-driven research tools also touches on the fundamental nature of what constitutes “research.” Mario Krenn, the leader of the Artificial Scientist Lab at the Max Planck Institute for the Science of Light in Erlangen, Germany, pointed out that while AI systems such as OpenAI’s deep research tool can generate literature reviews with remarkable speed, they do not engage in research in the traditional sense. Krenn argued that genuine scientific inquiry typically involves years of dedicated investigation and the evolution of new ideas—a process that AI, at least in its current form, has not yet demonstrated the capacity to replicate. Nonetheless, the potential of these tools to serve as valuable adjuncts to human research is undeniable. By rapidly aggregating and synthesizing vast quantities of information, they can provide researchers with a comprehensive starting point from which to identify novel hypotheses and explore unexplored areas of inquiry. This capability is particularly appealing in a landscape where the volume of scientific literature is growing exponentially, making it increasingly difficult for individual researchers to remain current with the latest developments in their fields.
The implications of such AI-driven research tools extend far beyond the realm of literature reviews. As the technology matures, there is considerable speculation that these systems could evolve into fully autonomous research assistants capable of generating original insights and even proposing new experimental methodologies. Andrew White, a chemist and AI expert at FutureHouse—a startup based in San Francisco—suggested that the next logical step in the evolution of these systems might be their integration into dynamic review processes, wherein AI-generated reports are periodically updated to reflect the latest advances in a given field. White noted that the traditional model of authoritative reviews, which are typically updated only every six months due to the labor-intensive nature of the process, may soon be supplanted by continuously updated, AI-driven documents that provide a real-time synthesis of the latest research findings. Such a transformation could have profound implications for academic publishing, research funding, and the overall pace of scientific progress.
It is also worth noting that the deep research tool, like its counterparts, faces technical limitations that may constrain its utility in certain contexts. A significant challenge is its inability to extract information from paywalled sources, which represent a substantial portion of the scientific literature. This limitation is particularly problematic in an era when access to comprehensive, high-quality information is more critical than ever. Researchers have proposed various workarounds, including the possibility of integrating institutional credentials or journal subscriptions into the tool’s framework, thereby enabling it to bypass paywalls. In response to such proposals, OpenAI’s CEO Sam Altman has acknowledged the need for a solution that reconciles the open-access ethos of scientific research with the proprietary nature of many academic publications. Altman’s comment underscores the broader challenge facing developers of AI research tools: balancing the imperatives of open science with the practical realities of information access in a commercially driven publishing landscape.
The interplay between the technical capabilities of the tool and its practical applications is further illuminated by its performance in real-world scenarios. Several scientists have reported that the deep research tool is particularly effective in generating literature reviews that are not only comprehensive but also well-organized and meticulously cited. In some cases, the tool has been used to identify gaps in existing research, thereby providing researchers with valuable insights into potential avenues for future investigation. Such applications are especially relevant in interdisciplinary fields, where the ability to quickly synthesize information from diverse domains can accelerate the pace of innovation and foster novel collaborations. By automating the more routine aspects of the research process, the deep research tool enables scientists to devote more of their time and intellectual resources to creative problem-solving and experimental design.
Critically, the adoption of AI tools such as OpenAI’s deep research tool raises important questions about the future of scientific work. As these systems become increasingly sophisticated, there is a growing debate about the role of human judgment in the research process. While some fear that an overreliance on AI-generated reports could lead to a diminution of critical thinking and independent inquiry, others argue that these tools will ultimately serve to augment human capabilities rather than replace them. In this view, AI systems are best understood as complementary instruments that can assist researchers by handling the labor-intensive aspects of data collection and synthesis, thereby freeing up human experts to focus on the more nuanced and interpretive aspects of scientific inquiry. Such a synergistic relationship between human researchers and AI tools has the potential to usher in a new era of accelerated scientific discovery, one in which the combined strengths of machine precision and human creativity are harnessed to tackle the most complex challenges of our time.
The broader implications of these developments extend well beyond the confines of academic research. As AI-driven tools become more integrated into various sectors, from healthcare and finance to education and public policy, their influence on decision-making processes and strategic planning is likely to grow. The deep research tool, with its capacity to rapidly aggregate and analyze vast amounts of information, exemplifies the transformative potential of AI to reshape not only the way research is conducted but also the manner in which knowledge is disseminated and applied in practical contexts. The promise of such tools lies in their ability to democratize access to information, enabling a wider range of stakeholders to engage with cutting-edge research and to participate in the collective endeavor of scientific advancement.
At the same time, the rapid evolution of AI research tools invites a reexamination of established norms and practices within the academic community. As the line between human-generated and machine-generated content becomes increasingly blurred, questions about authorship, intellectual property, and academic integrity are likely to come to the fore. It is incumbent upon researchers, publishers, and policymakers to develop robust frameworks that ensure the responsible and ethical use of AI in academic contexts, while also safeguarding the core values of transparency, rigor, and accountability that underpin the scientific enterprise. The ongoing dialogue about the merits and limitations of tools like OpenAI’s deep research system is thus not merely a technical discussion but also a broader reflection on the evolving nature of knowledge production in the 21st century.
In sum, the introduction of OpenAI’s deep research tool represents a watershed moment in the integration of artificial intelligence into the scientific research process. With its ability to synthesize information from a multitude of online sources into coherent, cited, and multi-page reports, the tool offers a powerful new means of generating literature reviews and full review papers in a fraction of the time traditionally required. While the tool is not without its limitations—such as occasional inaccuracies in citation and the challenge of accessing paywalled content—its potential to transform the way researchers engage with and synthesize information is undeniable. The enthusiastic responses from many in the scientific community, tempered by prudent caution from others, reflect a broader ambivalence about the role of AI in research—a tension between the promise of unprecedented efficiency and the imperative of maintaining rigorous scholarly standards.
As the tool continues to evolve and as further refinements are made to address its current shortcomings, it is likely that its impact on academic research will only grow more profound. The deep research tool stands as a testament to the power of AI to augment human capabilities and to catalyze new forms of intellectual inquiry. Whether it ultimately renders traditional literature review writing obsolete or merely serves as a valuable adjunct to human expertise remains to be seen; however, its emergence signals a clear shift in the landscape of academic research—a shift towards a future in which AI plays an increasingly central role in the generation, synthesis, and dissemination of knowledge.
In this context, it is essential to recognize that the evolution of AI research tools such as OpenAI’s deep research system is emblematic of a broader trend in which the boundaries of traditional scientific work are being redefined. As these tools become more sophisticated, they will undoubtedly spur further innovations in both the methodologies employed by researchers and the ways in which academic work is communicated and evaluated. The challenges associated with ensuring the accuracy, reliability, and ethical use of AI-generated content are significant, yet they are far outweighed by the promise of enhanced productivity, accelerated discovery, and the democratization of knowledge.
Ultimately, the deep research tool heralds a new era in which the convergence of advanced artificial intelligence and scholarly research creates opportunities for breakthroughs that were once the exclusive domain of human ingenuity. As researchers continue to explore the full potential of this technology, the scientific community will be tasked with navigating the complexities of this brave new world—a world in which the synthesis of human and machine intelligence offers the possibility of transformative progress across all fields of inquiry. The future of academic research, it seems, is poised to be both more efficient and more dynamic, driven by the relentless innovation of AI systems that are rapidly reshaping our understanding of what it means to “do research.”
Subject of Research: Artificial Intelligence, Academic Research, Literature Synthesis, Information Aggregation
Article Title : OpenAI’s ‘Deep Research’ Tool: Evaluating Its Utility for Scientific Inquiry
News Publication Date : 06 February 2025
Article Doi References : https://doi.org/10.1038/d41586-025-00377-9
Image Credits : Scienmag
Keywords : OpenAI, Deep Research, AI Tool, Literature Reviews, Academic Research, Large Language Models, Information Synthesis, Scientific Inquiry
Tags: academic research technologyadvancements in research methodologiesartificial intelligence in academic researchautomated literature synthesiscomprehensive cited reports in researchefficient literature review processeslarge language model for researchOpenAI deep research toolpressures on researchers for up-to-date knowledgereal-time internet search in researchrevolutionizing literature reviewstransforming scientific inquiry
What's Your Reaction?
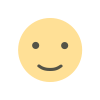
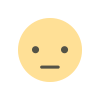
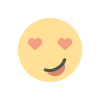
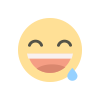
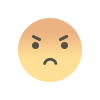
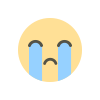
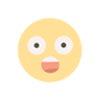