Machine Learning Predicts Short-Term Hub-Height Wind
In the relentless pursuit of sustainable energy, wind power has emerged as a cornerstone of the global shift toward cleaner, renewable sources. However, the inherent variability and unpredictability of wind continue to present formidable obstacles in optimizing power generation and ensuring grid stability. Addressing this challenge head-on, a groundbreaking study published in Nature Communications introduces […]

In the relentless pursuit of sustainable energy, wind power has emerged as a cornerstone of the global shift toward cleaner, renewable sources. However, the inherent variability and unpredictability of wind continue to present formidable obstacles in optimizing power generation and ensuring grid stability. Addressing this challenge head-on, a groundbreaking study published in Nature Communications introduces a cutting-edge machine learning model designed to enhance the accuracy of short-term wind speed predictions at hub heights—the critical elevation where wind turbines operate. This innovative approach not only promises to transform operational forecasting but also to revolutionize the integration of wind energy into power systems worldwide.
Understanding wind speed fluctuations, especially at turbine hub heights typically spanning 80 to 120 meters above ground level, is vital for several reasons. Accurate prediction enables better scheduling of wind power output, minimization of wear on turbine components, and informed maintenance strategies. Traditional forecasting methods, largely reliant on numerical weather prediction (NWP) models and physical simulations, often grapple with resolution limitations and computational demands that hinder reliable short-term forecasts. The new model developed by Zhang, Lin, Gao, and their collaborators employs advanced machine learning techniques to bridge these gaps, allowing for real-time, high-resolution wind speed forecasts that surpass the constraints of classical approaches.
At the heart of this innovation lies the integration of vast datasets encompassing meteorological variables, historical wind speed records, and turbine operational parameters. The researchers harnessed state-of-the-art neural network architectures capable of capturing complex, nonlinear patterns in the data, reflecting the chaotic nature of atmospheric dynamics. By training these models on extensive time-series data across various climatic zones and terrain types, the team ensured robust generalization and adaptability to diverse wind regimes. This level of adaptability is paramount for deploying the model across different wind farms, each with unique geographic and meteorological characteristics.
One of the pivotal advancements of this research is the incorporation of temporal and spatial attention mechanisms within the machine learning framework. These mechanisms enable the model to weigh the relative importance of input features dynamically, effectively discerning which data points are most relevant for accurate prediction at any given moment. Consequently, the model can adjust to sudden shifts in wind patterns, such as gusts or lulls, that traditional statistical models often fail to anticipate. This adaptability enhances both the precision and reliability of forecasts, which is crucial for operators who must make split-second decisions to stabilize the power grid.
Furthermore, the researchers confronted the notorious challenge of data sparsity and noise inherent in atmospheric measurements. To mitigate these issues, the model incorporates sophisticated regularization techniques and data augmentation strategies during training, thereby enhancing its robustness against imperfect or incomplete input data. This resilience ensures that the forecast remains consistent despite occasional sensor malfunctions or gaps in data collection, a common occurrence in remote or harsh environments where wind farms are frequently located.
From an operational perspective, the model’s capacity to predict wind speed with unprecedented granularity over short horizons—ranging from minutes up to several hours ahead—translates into tangible benefits. Grid operators can optimize power dispatch schedules, reducing reliance on fossil fuel-based peaking plants traditionally used to compensate for wind volatility. Grid stability improves, lowering the risks of blackouts or frequency fluctuations. Additionally, improved load forecasting enables more efficient energy trading, helping to stabilize energy markets and potentially driving down costs for consumers.
Crucially, this research aligns with the growing impetus to integrate artificial intelligence (AI) into energy systems not simply as a novelty but as a practical tool with measurable impact. By demonstrating that machine learning models can outperform classical meteorological models in short-term forecasting tasks, the study establishes a new paradigm. It changes the narrative from reactive to proactive energy management, where predictive analytics anticipate and mitigate volatility long before it affects power delivery. This is a leap forward in operational intelligence for wind energy systems.
The study also addresses the scalability of the approach. Given the proliferation of wind farms globally, solutions must be both scalable and adaptable. The model’s architecture, designed with modularity and transfer learning capabilities, allows for swift adaptation to local datasets or evolving meteorological conditions without necessitating complete retraining. This feature significantly reduces computational expenses and accelerates deployment timelines—both critical factors in real-world applications.
Moreover, the environmental implications cannot be overstated. By enhancing the predictability of wind energy generation, the model supports greater penetration of renewables into the energy mix. This progression is consistent with international decarbonization goals and energy security commitments. As intermittent renewable generation becomes more manageable and less disruptive, infrastructure investments in grid storage and backup fossil-fuel capacity may be reevaluated, leading to more sustainable and cost-effective energy systems.
Beyond technical prowess, the study underscores the importance of interdisciplinary collaboration. The fusion of atmospheric science, machine learning, and electrical engineering expertise exemplifies the multidisciplinary efforts required to solve complex real-world problems in energy systems. This integrative approach also facilitates the translation of academic research into commercial products and services, accelerating the path from innovation to industry impact.
Challenges, however, remain. The researchers note that while the model excels in short-term prediction, longer-term forecasts still rely heavily on established meteorological models. Integrating these methodologies presents an avenue for future exploration, potentially creating hybrid models that leverage the strengths of both physical simulations and data-driven AI approaches. Additionally, continuous monitoring and model retraining are necessary to account for changes in climate patterns and turbine technology upgrades, ensuring sustained accuracy over time.
The extensive validation efforts reported in the study—spanning multiple continents and varied climatic regimens—demonstrate the model’s versatility. This broad-based validation builds confidence among industry stakeholders regarding the feasibility of integrating such models into operational workflows. Pilot projects are already underway, exploring real-time deployment at commercial wind farms, with preliminary results indicating significant performance improvements and cost savings.
Looking ahead, the incorporation of complementary data sources such as satellite observations, lidar scans, and real-time turbine feedback promises to further refine forecast granularity and accuracy. The use of edge computing devices to process data onsite in wind farms could reduce latency and enhance responsiveness, opening new possibilities for adaptive turbine control and energy storage management.
In conclusion, the development of this advanced machine learning model represents a monumental stride in renewable energy forecasting, demonstrating how AI can be harnessed not just for innovation’s sake but to solve pressing challenges in sustainable power generation. As climate imperatives intensify, such technological breakthroughs will be indispensable in weaving renewables seamlessly into the global energy fabric. Zhang, Lin, Gao, and their team’s contribution sets a high bar and provides a compelling blueprint for future research and commercial application in wind energy prediction.
Subject of Research: Short-term wind speed prediction at turbine hub heights using machine learning models
Article Title: A machine learning model for hub-height short-term wind speed prediction
Article References:
Zhang, Z., Lin, L., Gao, S. et al. A machine learning model for hub-height short-term wind speed prediction. Nat Commun 16, 3195 (2025). https://doi.org/10.1038/s41467-025-58456-4
Image Credits: AI Generated
Tags: accurate wind speed modelsadvanced forecasting techniques for windenhancing grid stability with wind powerhub-height wind energy optimizationmachine learning for wind speed predictionMachine Learning in Renewable Energyovercoming wind variability challengesreal-time wind energy forecastingrenewable energy integrationshort-term wind forecastingsustainable wind power generationturbine maintenance strategies
What's Your Reaction?
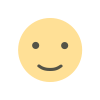
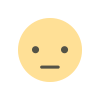
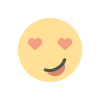
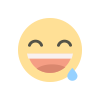
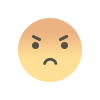
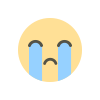
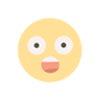