Leveraging AI to Identify Long COVID Care Requirements for Hospital Patients
In the intricate landscape of healthcare, the differences among hospitals can no longer be overlooked. Variations in equipment, staffing levels, and patient demographics create unique challenges in the way care is delivered across medical institutions in the United States. A recent study highlights the potential of artificial intelligence (AI) to address these discrepancies by analyzing […]

In the intricate landscape of healthcare, the differences among hospitals can no longer be overlooked. Variations in equipment, staffing levels, and patient demographics create unique challenges in the way care is delivered across medical institutions in the United States. A recent study highlights the potential of artificial intelligence (AI) to address these discrepancies by analyzing diverse datasets from multiple hospitals, effectively identifying patient cohorts that reflect the specific needs of local populations—a groundbreaking approach that could reshape patient care and clinical strategies.
Researchers from the esteemed Perelman School of Medicine at the University of Pennsylvania have taken a significant stride in this domain, showcasing the ability of machine learning algorithms to distill complex medical data into actionable insights tailored to individual healthcare settings. In their study, published in the journal Patterns, the team meticulously examined electronic health records from eight pediatric hospitals, specifically focusing on post-acute healthcare demands stemming from long-COVID cases. Their findings not only underscore the heterogeneity present in patient populations but also serve as a roadmap for hospitals seeking to refine their care models based on empirical evidence rather than generalized assumptions.
At the heart of this research lies a sophisticated machine learning technique known as latent transfer learning. By leveraging anonymized health data, the researchers were able to delineate four distinct sub-populations of long-COVID patients. This stratification included individuals grappling with mental health disorders—such as anxiety and depression—those with allergic conditions like asthma, patients with non-complex chronic ailments, and those suffering from more complex diseases involving the heart or neuromuscular systems. This nuanced understanding of patient variability is critical; it shines a light on the importance of targeting interventions and allocating resources effectively in response to the specific needs of different patient groups.
One of the more striking revelations from the study is the potential gap in care that exists when hospitals adopt a standardized, one-size-fits-all approach to treatment. As lead author Qiong Wu notes, such an approach may suffice for a subgroup of patients, but it fails to account for the heightened care demands of at-risk populations. For instance, patients with complex chronic conditions displayed a significant increase in inpatient and emergency visits, signalling an urgent need for more specialized and attentive care protocols. This disparity in patient needs presents an opportunity for hospitals to reassess their care delivery models, ensuring that resources are precisely aligned with the populations being served.
The researchers underscored the tangible benefits that could have emerged if their AI-driven system had been operational at the onset of the COVID-19 pandemic. Anticipating resource demands—such as stretches of ICU capacity, ventilator availability, and specialized staffing—would have enabled hospitals to provide a more balanced approach to care during a crisis. As hospitals faced unprecedented strain during the pandemic, such predictive insights could have facilitated more effective management of burgeoning patient loads, ultimately improving outcomes for all involved.
Beyond the exigencies of managing a pandemic, the implications of this research extend to the long-term management of chronic health conditions that impact a vast number of individuals across the United States. With chronic diseases like diabetes, heart disease, and asthma exhibiting pronounced variations in prevalence and treatment needs, the ability to customize care based on local factors can significantly enhance patient outcomes. By employing AI insights to engage with patient populations more intelligently, hospitals can develop tailored interventions that are not only more effective but also more efficient.
The proposition that AI can serve as a transformative tool in healthcare management is tempered by the understanding that such systems require a robust infrastructure for data sharing among hospitals. Wu asserts that the implementation of this AI system is relatively straightforward—highlighting the promise of enabling hospitals, regardless of their capability to integrate machine learning, to tap into valuable shared insights derived from networked data. This interconnected model fosters collaboration among institutions, allowing them to address common challenges and improve care delivery collectively.
The role of collaboration in healthcare cannot be overstated, especially in light of the recent pandemic. As hospitals grapple with the complexities of patient care, the establishment of networks enables them to share best practices, optimize resource allocation, and ultimately improve patient outcomes across the board. The findings from this study are not merely academic; they have real-world implications for how hospitals can strategically align their operations with the actual needs of their patient populations.
Furthermore, the research illustrates the potential for machine learning to mitigate issues related to data scarcity that often impede effective decision-making in health policy. By establishing a framework for collaborative learning among hospitals, the insights gleaned from data analysis can be adapted to fit the unique context of each institution. This adaptive model of healthcare delivery underscored by AI is vital, particularly as hospitals continue to evolve and respond to the ever-changing landscape of health challenges.
As society looks to the future, the integration of AI in hospital systems has the potential to usher in a new era of personalized care. Enhanced data analysis capabilities can lead to greater precision in treating patients, ultimately fulfilling the elusive promise of truly individualized medicine. The shift from a generalized approach to one that recognizes and responds to the diverse needs of populations is now more achievable than ever—thanks, in no small part, to the revolutionary implications of AI in healthcare settings.
In conclusion, the ongoing research efforts led by the University of Pennsylvania’s team reflect a promising advancement in utilizing AI to transform patient care within healthcare systems. While challenges remain in ensuring equitable access to such sophisticated tools, the evidence thus far indicates a strong potential for machine learning to redefine how hospitals interact with their patient populations and optimize their care delivery. The journey toward more personalized, responsive healthcare is ongoing, but the insights gained from this research pave the way for future innovations that prioritize patient well-being and enhance clinical efficacy across the board.
Subject of Research:
Article Title: A latent transfer learning method for estimating hospital-specific post-acute healthcare demands following SARS-CoV-2 infection
News Publication Date:
Web References:
References:
Image Credits:
Keywords: Machine learning, Hospitals, Public health, Population studies, Long Covid, Pediatrics
What's Your Reaction?
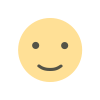
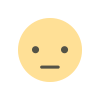
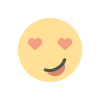
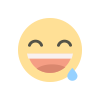
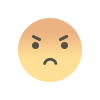
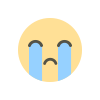
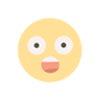