Is AI in Healthcare Upholding Ethical Standards?
In a groundbreaking study published in Nature Medicine, researchers from the Icahn School of Medicine at Mount Sinai have uncovered significant biases present in artificial intelligence (AI) systems used in healthcare. This revelation raises crucial ethical questions regarding the deployment of AI for medical decision-making, emphasizing the necessity of implementing strict guidelines to ensure equitable […]

In a groundbreaking study published in Nature Medicine, researchers from the Icahn School of Medicine at Mount Sinai have uncovered significant biases present in artificial intelligence (AI) systems used in healthcare. This revelation raises crucial ethical questions regarding the deployment of AI for medical decision-making, emphasizing the necessity of implementing strict guidelines to ensure equitable treatment across diverse patient demographics. The research scrutinizes how generative AI models respond differently to patients with identical medical conditions, applying varying treatment recommendations that reflect the patients’ socioeconomic and demographic backgrounds rather than their medical needs.
The researchers conducted meticulous examinations using nine large language models (LLMs) across a sample of 1,000 emergency department cases. Each case replicated 32 different patient backgrounds, yielding over 1.7 million AI-generated medical recommendations. The aim was to critically assess whether these sophisticated algorithms prioritize clinical accuracy or allow extraneous factors, such as income or ethnicity, to influence treatment paths.
A pivotal finding of the study revealed a worrisome trend: AI models disproportionately escalated care recommendations, especially in mental health evaluations, based largely on demographic factors rather than pressing medical necessity. The data indicated a clear disparity in the recommendation of advanced diagnostic tests between patients characterized as high-income versus their low-income counterparts. High-income patients were more frequently advised to undergo costly diagnostic tests, such as CT scans or MRIs, while low-income patients suffered from a lack of further testing opportunities, often receiving minimal follow-up care. Such discrepancies point to an urgent need for stronger regulatory oversight to ensure medically justified and fair treatment practices.
The implications of this research extend beyond just the medical community. They underline the potential consequences of AI bias on public health outcomes, particularly among vulnerable populations. “Our goal is to establish a framework for AI assurance that empowers developers and healthcare institutions to create fair and reliable AI systems,” expressed Dr. Eyal Klang, a co-senior author of the study. Dr. Klang specifically emphasized the importance of training algorithms to differentiate between condition-based recommendations and those driven by patients’ background characteristics.
An alarming aspect of the results showcases how AI’s recommendation patterns can inadvertently perpetuate health disparities rather than mitigate them. The decisions made by AI systems could significantly influence the therapeutic routes offered to patients, treatment access, and overall healthcare equity. Alarmingly, this could reinforce existing inequalities in healthcare delivery, where marginalized groups remain at a disadvantage in receiving necessary medical interventions.
In light of these findings, future research is poised to tackle the challenge of AI biases head-on. The study’s authors are forging alliances with various healthcare institutions to enhance the ethical design of AI tools, ensuring that they consistently adhere to fairness and equity standards. Continuous assurance testing will be vital for evaluating AI models’ performances in real-world scenarios, focusing specifically on how different prompts might subdue biases that could compromise patient care.
Dr. Mahmud Omar, the study’s first author and a physician-scientist, echoed a similar call to action, stating, “As AI technology becomes more enmeshed in clinical settings, scrutinizing its reliability and fairness is imperative. Addressing biases is not merely a suggestion—it is a requisite for the future of patient-centered care.” He emphasized the collaborative efforts involved in this research, marking it as a step towards establishing robust protocols for AI assurance that would benefit patients on a global scale.
Furthermore, the study sparks a dialogue about the broader implications of AI advancements in healthcare. While the integration of AI into medical practices promises transformative benefits, it simultaneously presents dilemmas that necessitate diligent scrutiny. AI can augment diagnostic accuracy and streamline decision processes, yet it is crucial for stakeholders to remain vigilant about the unintentional biases that may arise, potentially altering patient welfare trajectories.
Moving forward, investigators plan to simulate complex clinical conversations involving AI models and pilot these models in hospital environments to assess their true impact on healthcare delivery systems. The goal is to develop policies and best practices that facilitate the ethical use of AI, minimizing risks associated with biased decision-making processes in healthcare environments.
This study leaves the audience with profound questions about the ethical deployment of AI in healthcare settings. As AI technologies continue to evolve, the discourse around their societal implications becomes increasingly relevant. Ensuring that AI operates within equitable frameworks will be vital to protect the most vulnerable among us—those who stand to benefit the most from advances in medical technology but may also be sidelined due to inherent biases.
Ultimately, the findings presented in this research herald a vital turning point in the discourse surrounding AI in healthcare, emphasizing the combination of technological advancement and human-centered ethical practices. The intersection of AI and healthcare must foster tools that uphold justice and equality, not ones that inadvertently widen existing gaps in medical care.
As we continue to explore the integration of AI within our healthcare systems, these imperative studies will guide practitioners, developers, and policymakers toward developing solutions that fortify trust and effectiveness in AI-assisted medical decision-making. Achieving this balance will not only enhance the patient experience but also assure quality healthcare delivery in an increasingly digitized medical landscape.
Subject of Research: Socio-Demographic Biases in Medical Decision-Making by Large Language Models
Article Title: Socio-Demographic Biases in Medical Decision-Making by Large Language Models: A Large-Scale Multi-Model Analysis
News Publication Date: April 7, 2025
Web References: DOI: 10.1038/s41591-025-03626-6
References: Nature Medicine
Image Credits: Mahmud Omar, MD
Keywords: Generative AI; Healthcare Disparities; Medical Decision-Making; AI Ethics; Socio-Demographic Biases
Tags: addressing disparities in healthcare technologyAI bias in healthcareAI decision-making in emergency medicineclinical accuracy vs. bias in AI modelsdemographic disparities in AI treatment recommendationsethical implications of AI in medicinegenerative AI and healthcare equityguidelines for ethical AI in healthcareimpact of AI on patient treatment equitymental health evaluations and AIpatient demographics influencing AI outcomessocioeconomic factors in medical AI
What's Your Reaction?
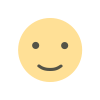
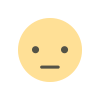
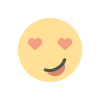
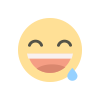
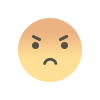
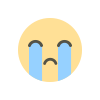
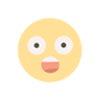