AI Technology Revolutionizes Monitoring of Multiple Sclerosis Treatment Efficacy
A groundbreaking development in the realm of medical imaging and artificial intelligence has emerged from researchers at University College London (UCL). This innovative tool, known as MindGlide, has been designed to revolutionize the assessment of treatment effectiveness for patients diagnosed with multiple sclerosis (MS). By harnessing advanced machine learning techniques, MindGlide aims to provide crucial […]

A groundbreaking development in the realm of medical imaging and artificial intelligence has emerged from researchers at University College London (UCL). This innovative tool, known as MindGlide, has been designed to revolutionize the assessment of treatment effectiveness for patients diagnosed with multiple sclerosis (MS). By harnessing advanced machine learning techniques, MindGlide aims to provide crucial insights into the nuances of the disease’s progression through detailed analysis of MRI scans.
AI entails leveraging mathematical models and algorithms to process vast datasets, allowing computers to replicate complex human-like cognitive tasks. Its applications range from predictive analytics to image recognition, showcasing the capability of machines to perform tasks traditionally requiring human expertise. In the case of MS, this technology offers the promise of rapid, accurate assessments that could enhance patient care and treatment outcomes.
MindGlide stands out in its ability to extract and analyze significant data from MRI scans of the brain. This includes identifying areas of damage, measuring brain shrinkage, and highlighting the presence of plaques, which are indicative of the disease’s progression. Given that MS is characterized by an autoimmune response that attacks the central nervous system, often leading to debilitating physical and cognitive impairments, the need for such precise imaging tools is paramount in managing and understanding the condition.
Statistically, MS affects around 130,000 individuals in the UK alone, imposing a considerable financial burden on the National Health Service, with costs exceeding £2.9 billion annually. To adequately study MS and test potential treatments, MRI markers serve as essential diagnostic tools. However, the effectiveness of standard hospital scans is often compromised by inconsistencies in the types of MRI scans utilized, which can limit the analysis of these crucial markers.
In their recent study published in Nature Communications, UCL researchers explored the capabilities of MindGlide, testing it against an extensive dataset comprising over 14,000 MRIs from more than 1,000 MS patients. The traditional process of analyzing these MRI scans typically demands the expertise of neuro-radiologists and can take weeks due to the healthcare system’s inherent workload. MindGlide, in contrast, is capable of delivering results in mere seconds—between five to ten seconds per image—demonstrating a significant leap forward in efficiency.
MindGlide’s performance has proven superior when benchmarked against existing AI tools, such as SAMSEG and WMH-SynthSeg. SAMSEG is utilized primarily for delineating various brain structures within MRI images, while WMH-SynthSeg detects and quantifies bright spots associated with conditions like MS. Remarkably, MindGlide surpassed these tools by being 60% more effective than SAMSEG and 20% more capable than WMH-SynthSeg at identifying and monitoring critical brain abnormalities like lesions.
Dr. Philipp Goebl, the first author of the research originating from UCL, expressed optimism regarding MindGlide’s potential to unlock valuable insights from existing medical archives. By integrating this AI system into routine clinical practice, researchers are hopeful that MindGlide will enhance understanding of MS and improve personalized treatment strategies for patients within the next five to ten years.
The findings indicate that MindGlide can accurately identify and measure vital brain tissues, even when utilizing limited or low-quality MRI data. This includes analyzing single-scan types that have not previously been leveraged for such evaluations, like T2-weighted MRIs without FLAIR sequences, notorious for complicating plaque visibility due to bright signals. Besides effectively tracking changes in the outer cortical regions of the brain, MindGlide has also successfully evaluated deeper structures.
Notably, the validation of MindGlide’s accuracy and reliability spans both cross-sectional and longitudinal analyses, confirming its effectiveness across annual scans by patients. The researchers faced substantial limitations in the past due to the quality of available clinical images, but the integration of AI presents an opportunity to tap into the wealth of information held within existing data reservoirs.
Dr. Arman Eshaghi, the principal investigator and head of the MS-PINPOINT group, highlighted the transformational potential of MindGlide. By utilizing previously underanalysed clinical images, the AI tool unlocks unprecedented opportunities for gaining insights into MS progression and treatment efficacy. The research team aims to adapt MindGlide for practical evaluation of MS therapies beyond the confines of clinical trials—to encompass diverse patient populations, thereby addressing the limitations faced in traditional research settings.
However, despite MindGlide’s advanced capabilities, it currently focuses solely on brain imaging and does not accommodate spinal cord assessments, which are crucial for evaluating disability levels in MS patients. As such, the researchers recognize the necessity for continued advancements and future explorations to create a more comprehensive approach that evaluates the entirety of the central nervous system.
The development of MindGlide is not merely a technical achievement; it reflects a broader trend where AI is reshaping medical diagnostics and treatment regimes. By effectively training on a substantial base of data—in this instance, an initial dataset comprising 4,247 MRI scans from nearly 3,000 patients—this deep learning model has demonstrated a profound understanding of disease markers. As the researchers utilized three separate databases comprising nearly 15,000 images for validation, the potential of MindGlide to influence both research and clinical practice becomes even clearer.
As researchers anticipate deploying the MindGlide tool in real-world healthcare settings, they remain committed to overcoming historical constraints imposed by inadequate imaging quality. The broader implications of successful implementation may well extend beyond MS, providing foundational methodologies for AI applications in other neurological disorders and enhancing global health outcomes.
In conclusion, the advent of MindGlide highlights a significant milestone in neurological research and patient care—bridging the gap between technology and medicine. The pursuit of improved diagnostic tools through AI paves the way for enhanced understanding and management of MS, offering hope and promising new avenues for patients grappling with the complexities of this chronic condition.
Subject of Research: People
Article Title: Repurposing Clinical MRI Archives for Multiple Sclerosis Research with a Flexible, Single-Contrast Approach: New Insights from Old Scans
News Publication Date: 7-Apr-2025
Web References: 10.1038/s41467-025-58274-8
References: [Not available]
Image Credits: [Not available]
Keywords: Multiple sclerosis, Human brain, Magnetic resonance imaging, Medical treatments, Tools, Research and development, Neurological data, Neuroimaging, Hospitals, Image analysis.
Tags: advanced imaging techniques for neurological disordersAI in medical imagingAI-driven healthcare solutionsassessing disease progression in MSautoimmune diseases and imagingcognitive impairments in multiple sclerosisEnhancing patient care with AImachine learning in healthcareMindGlide technology for MS treatmentMRI scan analysis for MSmultiple sclerosis treatment efficacyrevolutionary tools for monitoring MS
What's Your Reaction?
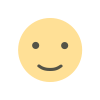
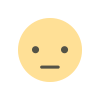
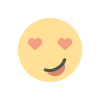
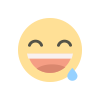
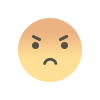
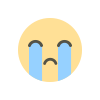
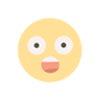