Imminent Breakthroughs in Fully Autonomous AI Technology
Researchers are on the verge of a significant breakthrough in artificial intelligence with the development of a novel algorithm named Torque Clustering. This innovative approach represents a substantial leap toward achieving a form of intelligence reminiscent of natural learning processes found in animals. Unlike traditional AI systems, which often require extensive human intervention to label […]

Researchers are on the verge of a significant breakthrough in artificial intelligence with the development of a novel algorithm named Torque Clustering. This innovative approach represents a substantial leap toward achieving a form of intelligence reminiscent of natural learning processes found in animals. Unlike traditional AI systems, which often require extensive human intervention to label data, Torque Clustering operates independently, allowing for a level of autonomy that could fundamentally change the landscape of AI.
While most existing AI technologies are rooted in supervised learning, which necessitates vast quantities of labeled data, Torque Clustering shifts the paradigm toward unsupervised learning. This method allows AI to uncover hidden structures and patterns within datasets without prior instruction or categorization from humans. By doing so, it alleviates some of the significant bottlenecks associated with data preparation and label generation, which are often cumbersome and resource-intensive.
The implications of Torque Clustering are profound. Researchers have tested this algorithm on an impressive array of 1,000 diverse datasets, achieving an astounding average adjusted mutual information (AMI) score of 97.7%. This score is indicative of the algorithm’s robustness and efficiency, far surpassing contemporary state-of-the-art methods, which typically hover in the 80% range. Such exceptional performance suggests that Torque Clustering may be poised to revolutionize how machines interpret complex data, offering unprecedented insights and accelerating discoveries across various fields.
One of the most intriguing aspects of Torque Clustering is its foundational basis in physics, particularly the concept of torque. This algorithm draws inspiration from the natural forces at play in the universe, exemplified by the gravitational interactions occurring during galaxy mergers. By leveraging properties such as mass and distance, Torque Clustering elegantly adapts to the varying characteristics of different datasets, overcoming challenges posed by differences in shapes, densities, and noise levels. This fundamental link to real-world physics not only enhances its operational capability but also infuses it with a layer of scientific significance that could reshape computational methodologies.
The research team emphasizes that Torque Clustering represents not just a technical advancement but a pivotal moment for the field of AI. Distinguished Professor CT Lin from the University of Technology Sydney elaborates on the innovative nature of the approach, noting that many current AI systems are limited by their reliance on predefined categories for data labeling. The opportunity to free AI from these constraints opens the door to a more intuitive learning process, akin to how living organisms learn from their environment by observation and interaction.
Dr. Jie Yang, the paper’s first author, highlights that the design of this algorithm could serve as a transformative force in the quest for general artificial intelligence. As robotics and autonomous systems evolve, incorporating Torque Clustering could dramatically enhance their ability to optimize movement, control, and decision-making processes. The implications of such advancements span a myriad of applications, from improving healthcare outcomes through better disease pattern detection to reducing financial fraud through sophisticated data analysis.
Moreover, Torque Clustering’s efficiency in data processing is noteworthy. In an era inundated with vast amounts of information, the ability to autonomously analyze and extract meaningful patterns presents a significant advantage for researchers and practitioners alike. By minimizing the need for extensive human intervention, this method allows for quicker responses and a more agile approach to research questions, potentially leading to the rapid generation of new hypotheses and innovations.
The publication of the research in IEEE Transactions on Pattern Analysis and Machine Intelligence marks a significant milestone in disseminating this groundbreaking work. As the algorithm’s open-source code has been made available, it invites other researchers to explore and expand upon its capabilities, fostering a collaborative environment for further advancements in unsupervised learning.
In conclusion, Torque Clustering stands not only as a testament to the potential of unsupervised learning but also as a pivotal chapter in the ongoing story of artificial intelligence. Its ability to operate independent of human-labeled data, combined with a robust performance demonstrated through rigorous testing, positions it as a key player in future AI developments. As researchers continue to refine and explore the vast applications of this method, the horizon of AI capabilities grows ever broader, hinting at a future where machines may learn and adapt in ways previously thought to be the exclusive domain of living beings.
As the academic community and industry alike begin to harness the potential of Torque Clustering, it is evident that this technology could redefine how we understand intelligence itself. The accompanying advancements in related fields may eventually pave the way for breakthroughs that echo across the scientific spectrum, enriching our understanding of both artificial intelligence and the world around us.
Subject of Research: Not applicable
Article Title: Autonomous clustering by fast find of mass and distance peaks
News Publication Date: 10-Feb-2025
Web References: DOI link
References: N/A
Image Credits: N/A
Keywords
Artificial intelligence, Cognitive robotics, Machine learning, Cognitive simulation, Autonomous knowledge acquisition, Robotic imitation, Computational simulation/modeling
Tags: AI performance metricsautonomy in artificial intelligencebreakthroughs in artificial intelligencedata preparation and label generationfully autonomous AI technologyinnovative AI approachesmachine learning advancementsnatural learning processes in AIrobust AI algorithmsTorque Clustering algorithmtransformative AI technologiesunsupervised learning methods
What's Your Reaction?
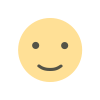
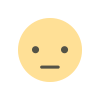
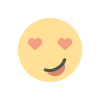
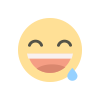
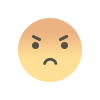
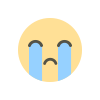
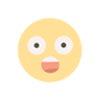