Advanced Machine Learning Model Enhances Detection of ‘Woody Breast’ in Chickens
In the world of poultry production, one particular issue is gaining unexpected attention due to its substantial economic impact: “woody breast” meat. This condition, characterized by an unusual hardness and chewiness in chicken breast, is distressing not only for poultry processors but also for consumers who are dissatisfied with the texture of their chicken sandwiches. […]

In the world of poultry production, one particular issue is gaining unexpected attention due to its substantial economic impact: “woody breast” meat. This condition, characterized by an unusual hardness and chewiness in chicken breast, is distressing not only for poultry processors but also for consumers who are dissatisfied with the texture of their chicken sandwiches. Recent research from the Arkansas Agricultural Experiment Station, affiliated with the University of Arkansas System Division of Agriculture, unveils a robust solution—a machine learning model supplemented by advanced hyperspectral imaging technology that accurately detects this defect with up to 95 percent accuracy.
Problematic woody breast meat accounts for significant yield losses, nearing $200 million annually for producers in Arkansas alone, but the implications stretch far wider into the United States poultry industry, with losses exceeding $1 billion annually when factoring in indirect costs. The woody breast defect, resulting from muscle development issues, is more prevalent in larger birds. Up to 20 percent of chicken breast meat from these larger fowl can exhibit this defect, posing a challenge for industry stakeholders aiming for quality assurance in meat products.
The advent of hyperspectral imaging technology, combined with sophisticated machine learning algorithms, marks a pivotal advancement in detecting woody breast. Through the analysis of various energy wavelengths, researchers can glean detailed insights into the composition of chicken meat. This innovative approach succeeds where traditional detection methods struggle, providing a non-invasive, real-time solution to deficiency identification. Researchers have implemented a process where hyperspectral images are not merely captured but also meticulously analyzed using the power of machine learning.
Key to the success of this research is the development of a new machine learning model known as NAS-WD, conceived and executed by Chaitanya Pallerla, a food science graduate student, under the guidance of Assistant Professor Dongyi Wang. The innovative architecture of NAS-WD allows for a more nuanced analysis of hyperspectral imaging data, effectively correlating multiple variables, something traditional models struggled to achieve. This capability to auscultate more than one variable simultaneously presents a significant advantage in the classification of woody breast meat.
The model leverages previous data about “woodiness” in chicken, extensively trained on existing datasets comprising known examples of both healthy and defected meat. The result is a pairing of accuracy and speed, with NAS-WD outperforming existing traditional models—support vector machines, and multi-layer perceptrons—across various metrics. While previous algorithms had been able to offer only around 80% or 73% accuracy respectively, the NAS-WD model sets a new benchmark, representing a paradigm shift in the detection of meat quality defects.
As technology continually evolves, researchers aim to integrate this hyperspectral imaging system into online applications, effectively placing this tool in the hands of poultry processors. This integration promises not only to enhance quality assurance practices but also to improve customer confidence in poultry products—an essential consideration in a competitive marketplace where consumer trust is vital.
Furthermore, hyperspectral imaging emerges as a non-invasive method to extract intricate data about food products, addressing various quality parameters promptly. Such innovation owes its success to the robust collaboration between machine learning and imaging technology, underscoring the necessity of interdisciplinary approaches in modern agricultural research.
The findings of this exciting research were thoroughly documented and presented in the journal Artificial Intelligence in Agriculture. The publication not only offers insights into the technical processes—such as the push broom methodology used for imaging—but also highlights the significance of hyperspectral imaging as a promising avenue for solving agricultural challenges. In effect, this study captures how algorithms and machine learning can refine traditional practices, bringing data-driven decision-making to the forefront of agricultural science.
As their methodologies become refined and implemented wider in the industry pipeline, researchers in Arkansas are optimistic about the future of poultry processing, seeing the potential to curtail the annual losses caused by woody breast. They envisage a landscape where technology enables processors to swiftly detect defects, thereby preserving the quality of poultry products that reach consumers’ plates. The implications are profound—not just for processing efficiency, but for enhancing end-user satisfaction in a market that increasingly values quality alongside ethical rearing practices.
In closing, this transformative approach offers a glimpse into the future of food quality assurance, inspiring further research and the development of related technologies. By harnessing the combined powers of hyperspectral imaging and machine learning, the poultry industry is poised to enhance its quality control measures significantly, benefiting producers and consumers alike. The research not only addresses a critical issue in the meat production industry but also exemplifies how agricultural methodologies must evolve to meet new consumer demands and standards.
Subject of Research: Detection of woody breast defect in chicken meat using hyperspectral imaging and machine learning
Article Title: Neural network architecture search enabled wide-deep learning (NAS-WD) for spatially heterogeneous property aware chicken woody breast classification and hardness regression
News Publication Date: 10-Dec-2024
Web References: Arkansas Agricultural Experiment Station
References: Artificial Intelligence in Agriculture
Image Credits: U of A System Division of Agriculture photo
Keywords: Machine learning, Artificial neural networks, Imaging analysis, Food quality detection, Hyperspectral imaging, Poultry processing.
Tags: advanced machine learning in poultry productionArkansas poultry industry advancementsconsumer satisfaction in poultry productsdetection of woody breast meateconomic impact of poultry defectshyperspectral imaging technology in agricultureimproving chicken meat textureinnovative solutions for meat quality issuesmachine learning applications in food safetypoultry processing challengesquality assurance in chicken meatreducing yield losses in poultry industry
What's Your Reaction?
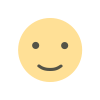
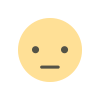
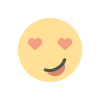
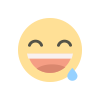
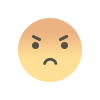
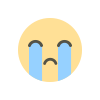
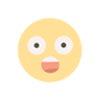