High-Dimensional Imaging via Combinatorial Multiplexing, AI
In the rapidly evolving domain of biomedical imaging, the quest to visualize and quantify numerous proteins within tissues while maintaining their spatial context has become a cornerstone for understanding cellular complexity and disease pathology. Traditional imaging methods, however, impose inherent limitations by dedicating a distinct imaging channel to each protein target. This approach severely restricts […]

In the rapidly evolving domain of biomedical imaging, the quest to visualize and quantify numerous proteins within tissues while maintaining their spatial context has become a cornerstone for understanding cellular complexity and disease pathology. Traditional imaging methods, however, impose inherent limitations by dedicating a distinct imaging channel to each protein target. This approach severely restricts the number of proteins that can be simultaneously visualized, owing to spectral overlap and available fluorophore channels, thereby capping throughput and scalability. Addressing this critical bottleneck, a pioneering study introduces an innovative technology named combinatorial multiplexing, or CombPlex, which harnesses the power of combinatorial staining strategies and advanced deep learning algorithms to transcend these limitations and exponentially amplify multiplexing capacity.
At its essence, CombPlex reconceptualizes the way proteins are encoded and decoded in imaging data. Rather than assigning each protein its own exclusive channel, multiple proteins are stained such that each imaging channel captures a composite image reflecting a unique combination of these proteins. This clever combinatorial compression reduces the number of needed imaging channels drastically. Following this data acquisition phase, a sophisticated deep learning framework processes the compressed images, computationally disentangling them to recreate individual, high-fidelity protein images. This algorithmic unmixing facilitates accurate and reliable recovery of the original protein-specific spatial expression patterns, a feat previously unattainable when using spectral multiplexing alone.
The development team demonstrated the potency of CombPlex by successfully compressing the staining of 22 distinct proteins into only five imaging channels, representing a remarkable reduction in channel usage by over fourfold. This compression level was shown to retain near-pristine proteomic spatial detail upon reconstruction, underscoring the robustness of the combined staining strategy and the AI-driven decompression algorithms. The dual utility of the method was validated not only in fluorescence microscopy—a widely used optical approach—but also extended seamlessly to mass-based imaging techniques. This cross-platform compatibility underscores CombPlex’s versatility and promise as a broadly applicable tool across diverse imaging modalities without necessitating specialized instrumentation or hardware upgrades.
Central to the CombPlex innovation is the intelligent design of staining panels, where proteins are assigned combinatorial barcodes reflecting unique presence or absence patterns across the compressed channels. Achieving effective encoding demands careful selection to minimize ambiguity and maximize the orthogonality of combinations, directly influencing the decompression model’s ability to accurately resolve individual protein images. The deep learning model, trained on vast datasets containing known staining combinations and imaging outputs, leverages convolutional neural networks to analyze spatial correlations and intensity patterns, enabling precise protein localization despite channel overlap.
Underpinning this strategy is a sophisticated computational pipeline that integrates image preprocessing, noise reduction, and artifact correction to optimize input quality for the neural network. This critical step ensures that the downstream decompression maintains accuracy, particularly in complex tissue environments where autofluorescence, nonspecific staining, or signal bleed-through might otherwise confound interpretation. The team reports that this approach not only facilitates accurate protein recovery but also enhances robustness against common imaging challenges, marking a significant leap forward in quantitative multiplexed imaging.
The impact of CombPlex extends far beyond mere channel reduction. By dramatically increasing the number of proteins that can be resolved within single cells and preserving their spatial organization, researchers gain unprecedented insight into tissue heterogeneity and cellular microenvironments. This enhanced resolution is particularly transformative for cancer biology, where tumor microenvironments embody complex cellular ecosystems with diverse phenotypes and signaling networks. The capacity to multiplex broadly paves the way for detailed phenotypic mapping, signaling pathway interrogation, and biomarker discovery at scales previously prohibited by previous imaging constraints.
Demonstrations within various cancer types and normal tissue specimens substantiate CombPlex’s practical relevance. The method consistently produced reconstructions that maintained biologically meaningful spatial distributions, enabling nuanced interpretation of both cellular and subcellular protein localization patterns. By validating these results across multiple tissue types, the study showcases the generalizability of the approach, reinforcing its potential for implementation across diverse research questions and clinical applications, including precise immunophenotyping and spatial proteomics.
Moreover, the authors highlight the advantage that CombPlex does not rely on specialized imaging hardware. Conventional fluorescence microscopes and existing mass-based imaging systems can adopt this technique without modification, leveraging computational upgrades to unlock vastly improved multiplexing capabilities. This accessibility democratizes high-dimensional imaging, providing laboratories worldwide with a cost-effective and scalable method to interrogate proteomic landscapes in situ without investing in complex and expensive instrumentation.
Looking ahead, the integration of combinatorial staining and AI-driven image decompression exemplifies a forward leap in the fusion of wet lab techniques with computational innovation. This synthesis not only tackles a foundational challenge in bioimaging but also charts a blueprint for future advances where experimental design and machine learning co-evolve. The study’s success establishes a new paradigm for multiplex imaging, and the underlying principles are expected to inspire extensions into other biomolecular targets, such as RNA and metabolites, further expanding the frontiers of spatial omics.
The significance of CombPlex is further accentuated in light of the growing emphasis on single-cell multi-omics. Efforts worldwide focus on unraveling the intricate molecular interplays that define cell states and functions within tissues. By enabling high-throughput spatial profiling of dozens of proteins within the native tissue context, CombPlex contributes an indispensable tool that complements transcriptomic and genomic analyses. Such integrative approaches are paramount for deciphering disease mechanisms, identifying therapeutic targets, and personalizing treatment strategies.
A pivotal feature of CombPlex is its scalability. Unlike linear multiplexing approaches, where each incremental protein necessitates additional channels or rounds of staining, combing protein signals into compressed channel sets creates an exponential multiplexing capacity. This scalability could support the detection of hundreds of proteins in a single tissue section in the future, simply by expanding the combinatorial staining schemas and fine-tuning the neural network models. Such high-parameter imaging would break current barriers in cell atlas projects and accelerate discovery in systems biology.
The study’s authors also acknowledge the challenges that remain. Precise control over staining protocols, cross-reactivity of antibodies, and computational complexity of the decompression models require continuous refinement. Ensuring reproducibility across different labs and preparing user-friendly software tools for broad dissemination will be essential next steps. However, the initial successes demonstrated here provide a solid foundation and clear pathway for iterative improvements.
In parallel, the ethical implications of deep learning-driven image reconstruction warrant careful consideration. Confidence measures and error estimation in decompressed images must be rigorously validated to prevent misinterpretation. Transparency about the algorithm’s decision-making process and incorporation of explainability features would foster trust among end-users, particularly in clinical settings where imaging-based diagnoses might be influenced by computationally reconstructed data.
In summary, CombPlex represents a transformative advancement at the interface of imaging technology and machine learning, redefining multiplexed protein detection and spatial biology. By exploiting combinatorial staining and deep learning reconstruction, this method circumvents the physical limitations of spectral imaging and enables a dramatic increase in multiplexing throughput. Its broad applicability, cost-effectiveness, and ability to maintain detailed spatial proteomic information render it a game-changer for biologists and clinicians alike, poised to illuminate the complexities of tissue architecture and disease with unprecedented clarity.
As spatial biology continues its meteoric rise, innovations like CombPlex will be central to unlocking the cellular mysteries embedded within tissues. The integration of computational and experimental strategies heralds a new era where complexity no longer imposes limits but instead fuels scientific discovery. This groundbreaking approach opens the door to comprehensive, high-dimensional mapping of proteins in situ, fostering a deeper understanding of biology’s fundamental units and offering new vistas for biomedical innovation.
Subject of Research: High-dimensional multiplexed protein imaging preserving spatial resolution in tissues
Article Title: High-dimensional imaging using combinatorial channel multiplexing and deep learning
Article References:
Ben-Uri, R., Ben Shabat, L., Shainshein, D. et al. High-dimensional imaging using combinatorial channel multiplexing and deep learning. Nat Biotechnol (2025). https://doi.org/10.1038/s41587-025-02585-0
Image Credits: AI Generated
Tags: advanced deep learning algorithmsalgorithmic unmixing methodsbiomedical imaging innovationscellular complexity analysiscombinatorial multiplexing technologydisease pathology understandinghigh-dimensional imagingimaging data encoding and decodingmultiplexing capacity enhancementprotein visualization techniquesspatial context in tissue imagingspectral overlap challenges
What's Your Reaction?
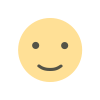
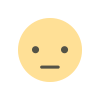
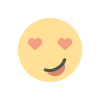
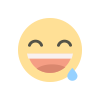
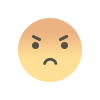
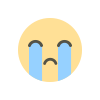
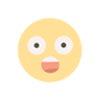