Groundbreaking Approach Enhances Accuracy of Radiologists’ Diagnostic Reports
In the intricate realm of medical diagnostics, the nuances of language can significantly impact clinical decision-making. Radiologists, tasked with interpreting complex medical images such as X-rays, often grapple with the ambiguity inherent in these visual representations. Their use of qualifiers like “may,” “likely,” or “possibly” when signaling the presence of pathologies has become a critical […]

In the intricate realm of medical diagnostics, the nuances of language can significantly impact clinical decision-making. Radiologists, tasked with interpreting complex medical images such as X-rays, often grapple with the ambiguity inherent in these visual representations. Their use of qualifiers like “may,” “likely,” or “possibly” when signaling the presence of pathologies has become a critical focal point in ensuring accuracy in medical communications. Trained to navigate this delicate balance, radiologists must convey their confidence levels in a way that not only reflects their expertise but also informs crucial clinical interventions.
Recent research emerging from MIT sheds light on a pressing question: Do the linguistic expressions employed by radiologists accurately mirror the likelihood of the conditions they describe? Conventional wisdom might suggest a straightforward correlation between the terms used and their corresponding probabilities. However, a newly conducted study illuminates a different reality, revealing that radiologists often lean towards overconfidence when using terms like “very likely,” and display a tendency for underconfidence when describing conditions as “possibly.” This disparity presents critical implications for patient diagnosis and treatment strategies, with the potential effects rippling throughout the healthcare system.
Collaborating with researchers and clinicians affiliated with prestigious institutions such as Harvard Medical School, a multidisciplinary team from MIT has developed a novel framework designed to quantify the reliability of radiologists’ confidence expressions. This innovative approach not only specifies how well certain terms correlate with diagnostic accuracy but also proposes more reliable alternatives that can enhance clinical reporting. The research holds promise for revolutionizing how radiologists communicate uncertainty, thereby improving patient outcomes through better-informed clinical decisions.
The intricacies of medical language often lead to confusion and misinterpretation. For instance, a radiologist may indicate a “possible” pneumonia in a report, prompting a physician to order further imaging tests to verify the diagnosis. In contrast, a declaration of “likely” pneumonia may trigger immediate treatment interventions, potentially impacting patient care swiftly and decisively. It is essential to recognize that each word carries distinct connotations that can lead to markedly different clinical actions; consequently, the accuracy of these linguistic indicators becomes paramount in the healthcare environment.
Analyzing the calibration of these terms presents complexities that traditional calibration methods have struggled to address. Typically, models derive predictions based on numerical confidence scores indicating the perceived likelihood of an event. An example could be a weather forecasting model that assigns an 83 percent chance of rain, which is considered well-calibrated if it rains 83 percent of the time in similar predictive instances. Yet, the human application of language diverges from this numerical precision, making it challenging to apply similar assessment methods to radiological language.
In response to these challenges, the MIT researchers propose a paradigm shift by treating certainty phrases not as singular probabilities but as probability distributions. A distribution allows for a broader representation of beliefs about an event, capturing the spectrum of potential outcomes tied to a particular word. This nuanced perspective enables a more accurate reflection of the inherent uncertainty that accompanies medical diagnostics, which is often not conducive to binary evaluations.
To develop their calibration framework, the research team tapped into prior studies that collected probabilistic assessments from radiologists about various diagnostic phrases. By mapping these phrases to their corresponding probability distributions, the researchers illuminated how terms like “consistent with” correlate with higher probabilities—often clustering above the 90 percent mark—while phrases like “may represent” exhibited wider distributions centered around 50 percent, effectively reflecting their ambiguous nature.
Through their optimization approach, the researchers introduced a calibration map designed to enhance the use of certain phrases based on specific pathologies. This enables radiologists to evaluate and reconsider their language choices, potentially replacing instances of overly confident or uncertain language with more calibrated phrasing. For example, it may be more appropriate to use “likely present” rather than simply “present” for certain diagnoses, ultimately aligning language with medical reality and improving communication.
Initial evaluations of clinical reports revealed notable patterns in the confidence radiologists exhibited across different diagnoses. For frequent conditions like atelectasis, they were generally underconfident, which could lead to missed or delayed treatments. Conversely, in more ambiguous circumstances, such as infections, radiologists tended to be overconfident, presenting a risk of unnecessary treatments or interventions that could complicate patient management.
The researchers further extended their methodology to assess the reliability of large language models, uncovering insights into how these systems express certainty in their predictions. Classical approaches, which often rely solely on confidence scores, may instill a false sense of security in correctness, discouraging critical inquiry. Therefore, this work stands to influence not only human practitioners but also the ongoing development of artificial intelligence applications in medical diagnostics.
Future endeavors aim to harness this framework beyond the realm of X-rays, with plans to integrate abdominal CT scan data, expanding the potential impact even further. Additionally, the researchers are keen on exploring how receptive radiologists are to adopting coaching-based suggestions for phrase calibration and whether adjustments in their communication strategies will translate into improved diagnostic practices.
The implications of this research could ripple across the medical community, reinforcing the critical nature of language in clinical settings. As the collaboration between technology and healthcare continues to deepen, the hope is that enhanced communication strategies, grounded in the evolving understanding of how language shapes decision-making, will ultimately foster better patient care outcomes. The transition towards more precise and reliable expression of confidence may empower both radiologists and treating physicians, driving a collective commitment to advancing diagnostic accuracy and embracing a future rich with improved medical practices.
In a landscape characterized by rapid technological advancement, this study offers a foundational perspective on the interplay between human language and artificial intelligence in medicine. As researchers pursue further investigations and bolster collaborations with medical practitioners, there is an overarching objective: to enrich the healthcare experience for patients by ensuring that the words used to frame diagnoses resonate with the realities of clinical evidence.
Such interdisciplinary efforts have the potential to redefine not only how we perceive medical communication but also how we approach the complexities of uncertainty in the face of evolving healthcare landscapes. As we strive to unpack the meanings behind words and ensure that they align with the probabilities they seek to represent, both the academic and medical communities may find common ground in their commitment to improving patient outcomes through precise communication.
In summary, the fusion of language calibration research with modern medical practice indicates a promising horizon for enhancing diagnostic accuracy and empowering healthcare professionals. With ongoing efforts to refine the relationship between communication and clinical efficacy, our medical institutions may well be on the precipice of a transformative era, one delineated by clearer perceptions of certainty and a shared understanding of the intricate dance between diagnosis and treatment.
Subject of Research: Calibration of Radiologists’ Language in Medical Reporting
Article Title: Bridging Ambiguity: Enhancing Communication in Radiology
News Publication Date: October 2023
Web References: MIT Press Release
References: ArXiv Preprint, MIT Research Collaborations
Image Credits: MIT Media Relations
Keywords
Radiology, Medical Imaging, Artificial Intelligence, Diagnostic Accuracy, Natural Language Processing, Uncertainty Quantification
Tags: accuracy in clinical decision-makingambiguity in medical imagingcommunication in healthcareimpact of language on patient diagnosislinguistic expressions in radiologymedical diagnosticsMIT research on radiologymultidisciplinary collaboration in medicineoverconfidence in medical languagepatient treatment strategiesradiologists’ diagnostic reportsunderconfidence in diagnostic terms
What's Your Reaction?
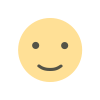
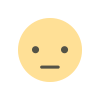
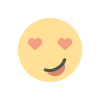
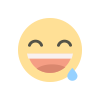
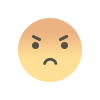
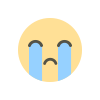
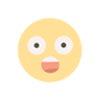