Built to Thrive: How Nurture Outweighs Nature in Robotic Hand Development
Researchers at the ValeroLab in the Viterbi School of Engineering have taken groundbreaking steps to unravel the complexities of how robotic arms and prosthetic hands learn to grasp and manipulate objects, specifically focusing on how they can learn to handle tasks like grasping and rotating a ball without relying solely on tactile sensation. Traditionally, the […]

Researchers at the ValeroLab in the Viterbi School of Engineering have taken groundbreaking steps to unravel the complexities of how robotic arms and prosthetic hands learn to grasp and manipulate objects, specifically focusing on how they can learn to handle tasks like grasping and rotating a ball without relying solely on tactile sensation. Traditionally, the ability of our hands to grasp objects has been attributed to the sensitive skin and intricate network of nerve endings that convey feedback to the brain, enabling fine motor control and adaptation during manipulation. This innate form of sensory feedback has been viewed as essential not just for human hands but also for artificial counterparts designed to mimic our dexterity.
However, the research team, composed of Romina Mir, Ali Marjaninejad, Andrew Erwin, and Professor Francisco Valero-Cuevas, pondered an important question: is tactile sensation absolutely necessary for the learning of complex manipulative tasks? They sought to discriminate between the influence of a hand’s inherent sensory capabilities—referred to as nature—and the training methods employed during the learning process, termed nurturing. This delicate interplay forms the crux of the research that intricately examines the foundations of dexterous manipulation.
In their study published in the prestigious journal Science Advances, the researchers aimed to resolve the long-standing “nature versus nurture” debate within the context of machine learning and robotics. Using advanced computational modeling and machine learning techniques, their paper titled “Curriculum Is More Influential Than Haptic Information During Reinforcement Learning of Object Manipulation Against Gravity” proposes that the structured sequence of learning experiences—essentially a tailored curriculum—is far more consequential than haptic feedback when it comes to enabling a robotic hand to learn how to interact with and manipulate objects effectively.
Their findings challenge the conventional wisdom that emphasizes the necessity of sensory feedback. Instead, the study demonstrates that by implementing a well-designed curriculum that prompts the robotic hand to learn through reinforcement learning, it can develop the capability to manipulate objects even in the absence of complete tactile inputs. This discovery could have wide-reaching implications, potentially revolutionizing the way robotic systems are programmed for dexterous tasks in various applications, from industrial automation to assistive technology for individuals with limb loss.
The researchers employed sophisticated simulation software to create a three-fingered robotic hand capable of interacting with virtual objects in a simulated environment. This engaging set-up allowed them to observe how different sequences of learning tasks influenced the robotic system’s ability to develop manipulation skills without fully relying on haptic sensation. Through this simulated environment, the study showcased how a carefully structured learning curriculum leads to substantial improvements in the system’s performance, thereby supporting the researchers’ hypothesis that the method of training plays a pivotal role.
Moreover, Francisco Valero-Cuevas, the corresponding author and Professor in the Division of Biokinesiology and Physical Therapy at USC, articulated the broader implications of this study, emphasizing that biological systems, like human hands, are also the products of their experiences and learning trajectories. By drawing parallels between machine learning processes and biological adaptation mechanisms, the research seeks to pave the way for advancements in artificial intelligence systems. These systems would not only be capable of learning and adapting based on their interactions with the physical world but would also become more effective in tasks that require nuanced motor control.
The collaboration between the Viterbi School of Engineering and the University of California, Santa Cruz, has brought together a diverse group of researchers, including doctoral students and faculty from both institutions. Notably, co-leading this innovative project, Parmita Ojaghi from UCSC and Romina Mir from USC highlighted how their collective contributions emphasize the significance of educational frameworks in both human and artificial learning. Their interdisciplinary efforts represent a leap forward in understanding the nuances of learning across different domains.
As this research unfolds, it sparks deeper inquiry into not only the mechanics of robotic movement but also the philosophical questions surrounding intelligence and the nature of learning itself. By questioning long-held assumptions about the necessity of tactile feedback, the study opens new avenues for exploration regarding how artificial systems can be designed to learn more efficiently. This has profound implications for the burgeoning field of robotics, particularly in the realm of rehabilitation engineering where prosthetics and robotic limbs are increasingly necessary.
The implications of this study resonate within several industries. In healthcare, for example, it may allow for the development of prosthetic limbs that can learn to operate with less sensory feedback, reducing costs and improving accessibility for individuals who require them. Meanwhile, industries reliant on automation and robotics could integrate these findings to create machines that can learn complex tasks more readily, enhancing productivity and efficiency.
In conclusion, the work done by the ValeroLab team adds significantly to our understanding of how both robots and prosthetics can evolve in their capability to manipulate the environment. It challenges established norms around the learning processes in robotics and offers insights that could lead to smarter, more adaptable automated systems. This research reinforces the belief that learning and adaptation remain core processes not just inherent to biological organisms, but also applicable and advantageous within artificial constructs.
As researchers continue to investigate these dynamics, the integration of curriculum-based learning models could reshape our future interactions with robotic systems. Allowing robots to learn more naturally could foster a new relationship between humans and machines, one built on a deeper understanding of both the physical and learning aspects of manipulation.
Potential future studies may focus on refining these computational models to incorporate more complex dynamics, ensuring that the resulting systems are robust and capable of handling real-world challenges. By understanding the essential components that contribute to successful learning in both humans and machines, we can pave the way for unprecedented advancements in robotic technology and artificial intelligence.
Ultimately, the journey of discovery in the intersection of robotics, biology, and artificial intelligence is just beginning. As we continue to unravel the threads connecting these fields, the potential outcome could redefine the contours of innovation, enhancing our ability to create machines that not only mimic human dexterity but also learn from their interactions in increasingly intelligent ways.
—
Subject of Research: The interplay of tactile sensation and training methods in teaching robotic arms and prosthetic hands to grasp and manipulate objects.
Article Title: Curriculum Is More Influential Than Haptic Feedback When Learning Object Manipulation
News Publication Date: 2-Apr-2025
Web References: http://dx.doi.org/10.1126/sciadv.adp8407
References: Not applicable
Image Credits: Not applicable
Keywords
Robotics, Artificial Intelligence, Haptic Feedback, Machine Learning, Object Manipulation.
Tags: advancements in robotic dexteritydexterous manipulation techniquesgrasping and manipulation tasksinfluence of training on robotic skillslearning methods in robotic systemsnature versus nurture in roboticsprosthetic hand technologyrobotic hand developmentsensory feedback in robotic armstactile sensation in roboticsValeroLab research findingsViterbi School of Engineering
What's Your Reaction?
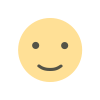
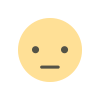
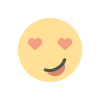
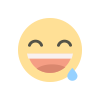
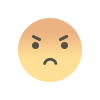
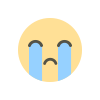
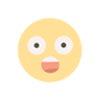