Generative AI Demonstrates Diagnostic Skills on Par with General Practitioners
In recent years, the burgeoning field of generative artificial intelligence (AI) has sparked considerable excitement across numerous sectors, none more so than in medicine. Generative AI, known primarily for its ability to produce human-like text, images, and other data, holds particular promise in diagnostic medicine. The potential for AI systems to analyze symptoms, interpret medical […]

In recent years, the burgeoning field of generative artificial intelligence (AI) has sparked considerable excitement across numerous sectors, none more so than in medicine. Generative AI, known primarily for its ability to produce human-like text, images, and other data, holds particular promise in diagnostic medicine. The potential for AI systems to analyze symptoms, interpret medical images, and suggest diagnoses offers the tantalizing prospect of augmenting or even transforming healthcare delivery. Despite the vast literature emerging in this arena, the variability in evaluation standards has posed significant challenges in assessing exactly how well generative AI performs compared to human clinicians. Addressing this gap, a landmark meta-analysis led by Dr. Hirotaka Takita and Associate Professor Daiju Ueda at Osaka Metropolitan University has systematically synthesized evidence from the last six years to benchmark generative AI’s diagnostic accuracy against that of physicians.
The research team undertook a comprehensive review of 83 peer-reviewed studies published between June 2018 and June 2024, encompassing a diverse array of medical specialties including internal medicine, radiology, dermatology, and pathology among others. Central to the analysis were large language models (LLMs) such as OpenAI’s ChatGPT, which emerged as the most frequently investigated generative AI framework within these studies. The meta-analysis aimed to cut through the methodological heterogeneity that has characterized previous research, applying rigorous statistical techniques to unify diagnostic performance metrics and enable direct comparison between AI-driven and human-generated diagnoses.
Their findings revealed that while generative AI systems have made impressive strides, there remains a notable gap when juxtaposed with expert clinicians. On average, human medical specialists outperformed generative AI models by approximately 15.8% in diagnostic accuracy. Specifically, AI achieved an average diagnostic accuracy rate of 52.1%, a figure that at first glance may seem modest but is highly nuanced upon deeper inspection. Intriguingly, the most advanced and recent generative AI architectures demonstrated diagnostic performance that rivals that of non-specialist physicians, illuminating a critical potential niche where AI can serve as an effective diagnostic ally, particularly in settings with limited access to medical expertise.
This meta-analysis underscores key distinctions in proficiency between specialist doctors—who undergo years of intensive training within focused disciplines—and current AI models operating primarily as generalized problem solvers. While specialists integrate complex clinical reasoning, experience-based heuristics, and contextual knowledge in their diagnostic processes, generative AI primarily relies on pattern recognition across vast datasets. As such, AI’s proficiency remains exceptional for routine or less complicated cases but diminishes in the face of rare diseases or nuanced clinical presentations requiring deep expert insight.
Dr. Takita highlighted the pragmatic implications of these findings, emphasizing the transformative role generative AI could play in medical education and healthcare delivery. “Our research shows that AI’s diagnostic capabilities are comparable to those of non-specialist doctors,” he explained. “This positions generative AI as a valuable tool for supporting clinicians who may not have specialized training, thereby potentially improving diagnostic accuracy in resource-poor environments or during initial patient assessments.” The integration of AI diagnostic support systems could democratize healthcare by extending high-quality diagnostic assistance beyond traditional academic medical centers and urban hospitals.
However, the researchers caution that considerable work remains before generative AI can be fully trusted as a diagnostic partner. Future investigations must rigorously evaluate AI performance in more complex, real-world clinical scenarios, including multifaceted patient histories and comorbidities that challenge straightforward diagnostic algorithms. Moreover, ongoing studies will need to incorporate actual medical records, rather than hypothetical or simulated cases, to better approximate clinical realities. Enhancing the interpretability and transparency of AI decision-making processes also remains paramount to fostering clinician trust and ensuring accountability.
Notably, ethical and equity concerns must inform the development and deployment of diagnostic AI. It is incumbent upon the scientific community to verify that AI models are rigorously validated across diverse patient populations, including underrepresented groups that historically suffer from healthcare disparities. Ensuring fairness and minimizing biases embedded within training data will be critical in preventing AI from inadvertently perpetuating inequities in medical diagnosis and treatment.
The Osaka Metropolitan University group’s work has been published in npj Digital Medicine, a reputable open-access journal dedicated to digital health innovations. Their comprehensive meta-analysis not only consolidates the current state of generative AI in diagnostics but also provides a valuable roadmap for future research agendas. As generative AI models continue to evolve at an unprecedented pace, with expanding capabilities in natural language processing and multimodal data integration, their diagnostic accuracy is expected to improve, potentially narrowing the gap with human specialists.
In the meantime, the responsible application of generative AI as a supplementary tool rather than a standalone diagnostician represents the most viable pathway for clinical integration. Such an approach leverages the strengths of both human expertise and AI efficiency, optimizing patient care outcomes while mitigating risks associated with overreliance on artificial systems.
The implications extend beyond individual patient encounters; widespread adoption of generative AI diagnostic assistants could help alleviate workforce shortages, reduce clinical burnout, and streamline healthcare workflows amidst increasing demand. Furthermore, AI could accelerate knowledge dissemination and continuing education among healthcare providers, offering instant access to the latest evidence-based guidelines and diagnostic frameworks.
This meta-analysis serves as both a milestone and a clarion call, inviting the global medical and AI research communities to collaborate extensively. Harmonizing data standards, developing robust evaluation frameworks, and fostering transparent reporting practices will catalyze innovation and ensure that AI diagnostic tools are rigorously vetted and equitably implemented across healthcare systems.
In summary, while generative AI today does not yet surpass human medical specialists in diagnostic accuracy, its current capabilities approximate those of non-specialist doctors, highlighting a significant opportunity to transform medical practice. Through continued research, technological refinement, and ethical stewardship, generative AI stands poised to become an invaluable partner in the quest for more accessible, accurate, and efficient medical diagnostics worldwide.
—
Subject of Research: People
Article Title: A systematic review and meta-analysis of diagnostic performance comparison between generative AI and physicians
News Publication Date: 22-Mar-2025
Web References: http://dx.doi.org/10.1038/s41746-025-01543-z
References: Published in npj Digital Medicine
Keywords: Generative AI, diagnostic accuracy, large language models, medical diagnostics, ChatGPT, meta-analysis, AI in healthcare, medical education, AI ethics, clinical decision support
Tags: AI diagnostic accuracy compared to physiciansapplications of generative AI in medicinechallenges in assessing AI healthcare performancedermatology and AI integrationevaluation standards in AI diagnosticsgenerative artificial intelligence in healthcarehealthcare delivery transformation with AIinternal medicine AI diagnosticslarge language models in medicinemeta-analysis of AI diagnostic performancepeer-reviewed studies on AI in healthcareradiology AI tools
What's Your Reaction?
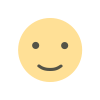
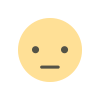
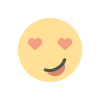
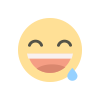
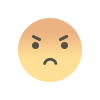
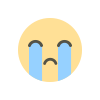
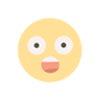