Enhancing Time Series Data: Exploring Intra-Class Similarity Mixing for Improved Augmentation Techniques
In an era defined by the meteoric rise of artificial intelligence and machine learning, the intersection of advanced computational methods and time series analysis is presenting unprecedented opportunities and challenges. A pressing issue in the field of time series classification is the scarcity of training data, particularly in environments where anomalous events occur infrequently. This […]

In an era defined by the meteoric rise of artificial intelligence and machine learning, the intersection of advanced computational methods and time series analysis is presenting unprecedented opportunities and challenges. A pressing issue in the field of time series classification is the scarcity of training data, particularly in environments where anomalous events occur infrequently. This gap presents a significant hurdle for researchers and practitioners aiming to develop robust models capable of identifying rare events amidst noise—and it is in this context that innovative strategies are emerging to enhance model effectiveness.
A recent study conducted by a research team led by eminent scholars Pin Liu, Rui Wang, Yongqiang He, and Yuzhu Wang provides a comprehensive approach to addressing this challenge through a novel time series augmentation technique known as Intra-class Similarity Mixing (ISM). By focusing on the interplay between intra-class feature similarity and augmented data diversity, this method not only demonstrates a pathway to expanding data sets but also enhances the overall classification performance of deep learning models.
The ISM method involves a meticulous process of matching similar local segments within intra-class time series before executing a mixing operation that generates new augmented samples. By leveraging region-specific features rather than mixing global attributes, the researchers aim to retain the essence of the original data while introducing new samples that are indiscernibly similar to the existing data points. This localized approach marks a significant departure from traditional augmentation techniques that often indiscriminately blend larger data segments, which can inadvertently introduce irrelevant features and confound classification tasks.
One of the most significant advantages of this research lies in its experimental validation. The team conducted extensive experiments across ten representative data sets sourced from the UCR2018, a benchmark in time series classification. Through careful evaluation, they recorded critical metrics such as classification performance, processing time, and the effects of varying batch sizes on model training. The results underscore the effectiveness of the ISM method, which not only outperformed existing augmentation strategies but did so with significantly reduced computational overhead.
A crucial finding of the study is the resilience of the ISM technique against fluctuations in batch size. Regardless of how large the batch size was during training, the ISM method maintained its effectiveness, suggesting that it may provide a reliable augmentation strategy adaptable to various training regimes. This outcome is particularly beneficial in real-world applications where computational resources and time are often limited.
As companies and organizations increasingly rely on deep learning models for automated anomaly detection—especially in critical sectors such as industrial monitoring, healthcare, and finance—the implication of developing reliable and efficient time series classification systems cannot be overstated. The ability to accurately detect rare events in vast streams of data can drive significant operational efficiencies, minimize risks, and contribute to better decision-making processes. Therefore, advances such as ISM have substantial practical value.
Moreover, the study highlights the potential for ISM to contribute to broader applications within the field of data science, possibly transforming how researchers and practitioners approach data scarcity and class imbalance in various scenarios beyond time series classification. For instance, industries facing challenges in obtaining sufficient labeled data could leverage ISM to artificially bolster their training datasets, thereby enhancing the robustness of their predictive models.
This ground-breaking research was published on December 15, 2024, in the journal Frontiers of Computer Science, an esteemed publication co-published by Higher Education Press and Springer Nature. The paper not only provides vital insights for researchers in the field of machine learning but also acts as a guide for future exploration into the realm of data augmentation strategies. The application of ISM could pave the way for further innovations and refinements in model training that prioritize feature integrity while expanding data diversity—an essential balance.
As we move forward into a future increasingly dominated by digital narratives driven by AI and data-driven insights, the significance of such research cannot be overlooked. The strategies and methodologies underpinning ISM serve as critical cornerstones in understanding how we can better navigate the complexities of time series data and enhance the performance of computational models tasked with this intricate and often unpredictable type of analysis.
Continuous exploration and innovation are essential to adapting and refining techniques like ISM in the face of evolving challenges in time series classification. As researchers and practitioners delve deeper into this domain, ongoing collaboration and knowledge sharing will be paramount to advance methodologies that are effective, efficient, and adaptable. Exploring nuances in data and improving augmentation processes are vital steps toward building smarter, more capable systems that can thrive in a data-rich future.
In conclusion, the research spearheaded by Liu and his colleagues not only contributes to the academic landscape of machine learning and statistics but also serves as an important reminder of the transformative potential that lies within well-conceived data augmentation methodologies. By moving beyond conventional approaches and venturing into the realm of intra-class similarity, the implications for improving anomaly detection and time series analyses could indeed reshape the operational capabilities of numerous industries, fostering an era of informed decision-making and smarter technologies.
Subject of Research: Time series augmentation techniques
Article Title: ISM: Intra-class Similarity Mixing for Time Series Augmentation
News Publication Date: 15-Dec-2024
Web References: Link to Article
References: Liu, P., Wang, R., He, Y., & Wang, Y. (2024). ISM: Intra-class Similarity Mixing for Time Series Augmentation. Frontiers of Computer Science. DOI: 10.1007/s11704-024-40110-9
Image Credits: Pin Liu, Rui Wang, Yongqiang He, Yuzhu Wang
Keywords
Applied sciences and engineering, Computer science
What's Your Reaction?
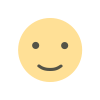
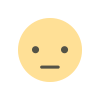
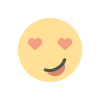
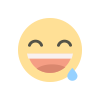
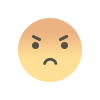
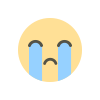
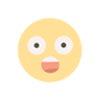