AI Uncovers Early Signs of Cognitive Decline During Menopause Transition
CLEVELAND, Ohio (Jan 15, 2025)—In an extraordinary leap forward in the intersection of artificial intelligence and healthcare, researchers have harnessed the power of machine learning to shed light on a critical aspect of women’s health: subjective cognitive decline during the menopause transition. As this pivotal period of life often marks significant physiological changes, it is […]

CLEVELAND, Ohio (Jan 15, 2025)—In an extraordinary leap forward in the intersection of artificial intelligence and healthcare, researchers have harnessed the power of machine learning to shed light on a critical aspect of women’s health: subjective cognitive decline during the menopause transition. As this pivotal period of life often marks significant physiological changes, it is crucial to evaluate how these changes impact cognitive function. Emerging evidence suggests that machine learning algorithms can provide quicker and more cost-effective identifications of women experiencing severe cognitive decline, potentially enabling better management strategies for cognitive health.
Subjective cognitive decline, characterized by an individual’s perception of declining memory or cognitive abilities, is one of the more prevalent concerns related to menopause. This issue is not merely a transient experience; rather, it indicates a pressing need for attention, as it could signal an increased risk for serious neurodegenerative diseases, including Alzheimer’s disease. The psychological impacts of cognitive decline can dramatically diminish quality of life, making effective identification and intervention vital. Current research indicates that cognitive decline during menopause is a symptom that should not be overlooked, as it could lead to long-term health complications.
Various factors have been identified in previous studies as contributing to the risk of cognitive decline. These factors include traditional aspects such as aging and hypertension, as well as lifestyle factors like obesity and depression. However, the extant models predominantly focus on dementia, an irreversible condition where opportunities for intervention are limited. Recognizing that subjective cognitive decline might not always be indicative of long-term cognitive deterioration, researchers propose creating a predictive model that can facilitate early interventions essential for preserving cognitive health. Such foresight could lead to significant strides in clinical practices.
Most conventional cognitive performance assessments rely heavily on complex models that often incorporate a range of laboratory indicators, including blood glucose levels, lipid profiles, and brain imaging results. While these sophisticated approaches have their place in the field, they are frequently cost-prohibitive and overly complex, hindering widespread application in clinical environments. Alternative models, especially those based on questionnaires, are poised to revolutionize the landscape. They prioritize a straightforward yet comprehensive understanding of individual variables, drawing on sociodemographic, lifestyle, work-related, and psychological factors, thus enhancing both accessibility and feasibility.
The advent of machine learning offers a transformative opportunity in the domain of cognitive health, particularly with the growing availability of large datasets. The ability of machine learning algorithms to identify patterns and trends among vast amounts of information enables the creation of reliable models that can rapidly process complex interrelationships among variables. In a pioneering study involving over 1,200 women navigating the menopause transition, researchers successfully developed and validated a machine learning model capable of detecting women experiencing severe subjective cognitive decline along with correlated risk factors.
The implications of these findings are far-reaching. They represent a fresh paradigm for designing targeted interventions that could effectively safeguard cognitive health among women during an often-challenging life phase. The researchers emphasize the necessity for further investigations to validate the current results, allowing for the exploration of additional factors that may influence cognitive health during menopause. The potential for machine learning as a supportive tool in clinical practices is becoming increasingly evident, showcasing its role in identifying individuals at higher risk and guiding interventions.
Dr. Stephanie Faubion, the Medical Director for The Menopause Society and a leading voice in the discussion, underscores the significance of these findings. The study not only highlights the efficacy of employing machine learning in identifying severe subjective cognitive decline, but it also lays the groundwork for future efforts that can facilitate early identification of at-risk individuals. Such foresight may lead to timely interventions that protect cognitive health—a vital aspect of women’s overall well-being.
Looking ahead, the research community acknowledges the importance of longitudinal studies that incorporate objective measures of cognitive performance. Such endeavors are crucial to thoroughly understanding the associations uncovered in this study. By delving deeper into the emerging relationships between menopause and cognitive health, researchers can enhance the understanding of these issues. This could, in turn, lead to the development of strategic protocols for interventions designed to optimize cognitive health during the menopause transition.
As more women experience the challenges of menopause, the urgency surrounding research in this domain intensifies. Engaging with machine learning not only supports an objective understanding of cognitive decline but also promotes a proactive stance against long-term health concerns. The implications extend beyond academic inquiry; they speak directly to improving the quality of life for countless individuals.
The increasing prevalence of subjective cognitive decline necessitates a multi-faceted approach. Effective interventions could hinge on a better understanding of how various lifestyle choices, mental health considerations, and even work-related stressors contribute to these cognitive shifts. Investigating these areas presents an exciting opportunity for future research and clinical practice, indicating a shift towards a more personalized, data-driven approach to women’s health care.
With an evolving understanding of the menopause transition and its ramifications on cognitive health, society stands at a crossroads where technology-driven insights could reshape clinical practices. Harnessing AI and machine learning may yield transformative strategies that prioritize women’s cognitive health in a manner that is both efficient and deeply considerate of their unique experiences. As researchers continue to explore and refine these methodologies, the possibility of improved cognitive health outcomes for women becomes increasingly within reach.
In conclusion, the integration of machine learning into the assessment of cognitive decline presents an innovative frontier in women’s health care. As the conversation surrounding menopause and cognitive health intensifies, ongoing research, coupled with advanced machine learning techniques, offers the potential to revolutionize how we understand and address these critical issues.
Subject of Research: People
Article Title: Using machine learning models to identify severe subjective cognitive decline and related factors in nurses during the menopause transition: a pilot study
News Publication Date: 15-Jan-2025
Web References: https://menopause.org/wp-content/uploads/press-release/MENO-D-24-00284.pdf
References: http://dx.doi.org/10.1097/GME.00000000000002500
Image Credits: Not Applicable
Keywords: Menopause, Machine Learning, Cognitive Health, Subjective Cognitive Decline, Neurodegenerative Diseases
What's Your Reaction?
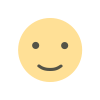
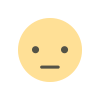
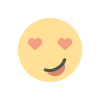
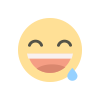
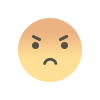
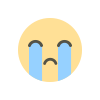
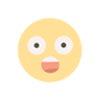