Breast Cancer Subtype Prediction via Ultrasound
In a groundbreaking advancement for breast cancer diagnostics, researchers have unveiled predictive models capable of distinguishing breast cancer molecular subtypes by integrating multimodal ultrasound imaging with clinical features. This innovative approach leverages the synergy of conventional ultrasound, shear wave elastography, and contrast-enhanced ultrasound to decode the complex biological signatures that differentiate luminal A, luminal B, […]

In a groundbreaking advancement for breast cancer diagnostics, researchers have unveiled predictive models capable of distinguishing breast cancer molecular subtypes by integrating multimodal ultrasound imaging with clinical features. This innovative approach leverages the synergy of conventional ultrasound, shear wave elastography, and contrast-enhanced ultrasound to decode the complex biological signatures that differentiate luminal A, luminal B, HER2-overexpressing, and triple-negative breast cancers. With breast cancer remaining one of the most prevalent and heterogeneous malignancies worldwide, these models promise to revolutionize personalized treatment strategies by providing more accurate, non-invasive molecular profiling.
Breast cancer classification traditionally hinges on immunohistochemical assessments of tissue biopsies to determine molecular subtypes. These subtypes—luminal A, luminal B, HER2-overexpressing (HER2), and triple-negative breast cancer (TNBC)—have distinct prognostic and therapeutic implications. However, biopsy procedures can be invasive, time-consuming, and sometimes limited by tumor heterogeneity or sampling errors. Therefore, developing reliable imaging-based prediction tools could significantly enhance early diagnosis and individualized treatment planning.
Multimodal ultrasound imaging has emerged as a powerful, radiation-free diagnostic modality capable of capturing diverse tissue characteristics. Conventional ultrasound (CUS) provides morphological information such as lesion size, shape, and echogenicity. Shear wave elastography (SWE) quantifies tissue stiffness, reflecting biomechanical changes associated with malignancy. Contrast-enhanced ultrasound (CEUS) assesses tumor vascularity and perfusion patterns, offering insights into angiogenic activity. The integration of these imaging techniques captures a holistic view of tumor biology, potentially correlating imaging phenotypes with molecular subtypes.
In this comprehensive study, breast cancer patients who underwent CUS, SWE, and CEUS imaging from January 2023 to June 2024 were meticulously analyzed. Researchers selected pertinent clinical and imaging parameters that revealed statistically significant variations among breast cancer molecular subtypes. Ten critical features emerged, including BI-RADS categorization, presence of palpable mass, tumor aspect ratio, maximum diameter, calcification status, heterogeneous echogenicity, irregular lesion shape, the standard deviation of the elastic modulus within lesions, and CEUS parameters such as arrival time and peak intensity.
Building on these findings, the research team developed multiple binary prediction models targeting each molecular subtype independently. The models were constructed from several feature sets: CUS features alone, SWE features alone, CEUS features alone, and a comprehensive full-parameter feature set that amalgamated data across all imaging modalities alongside clinical information. This stratified modeling approach allowed for a nuanced comparison of the predictive power contributed by each modality.
The results underscored the superior performance of models utilizing full multimodal parameter integration. Each prediction model demonstrated higher accuracy and robustness when all imaging and clinical variables were considered collectively, compared to models limited to single-modal features. Specifically, the area under the receiver operating characteristic curves (AUCs) for the full parameter models were 0.81 for luminal A, 0.74 for luminal B, 0.89 for HER2-overexpressing, and 0.78 for triple-negative breast cancer. These metrics reflect strong discriminative ability, essential for clinical decision-making.
Importantly, these findings affirm that molecular heterogeneity in breast cancer manifests as distinct imaging phenotypes detectable via advanced ultrasound techniques. Features such as tissue stiffness variability and contrast enhancement patterns appear intimately linked to underlying tumor biology, including cellular proliferation rates, hormone receptor expression, and vascular architecture. This concordance between imaging biomarkers and molecular subtypes opens new avenues for non-invasive tumor characterization.
From a clinical perspective, these prediction models have notable implications. Accurate preoperative identification of molecular subtype can guide therapeutic choices—ranging from endocrine therapy suitability for luminal cancers to targeted HER2-directed therapies or chemotherapy regimens tailored for triple-negative tumors. Moreover, non-invasive imaging could facilitate serial monitoring of tumor evolution or response to therapy without repeated biopsies.
The adoption of multimodal ultrasound in standard clinical workflows also offers logistical and economic benefits. Ultrasound devices are widely accessible, cost-effective, and do not expose patients to ionizing radiation, making them particularly suitable for frequent monitoring and application in resource-constrained settings. These advantages bolster the feasibility of personalized management strategies informed by imaging-based molecular classification.
Technically, the study employed rigorous statistical analyses to identify discriminative features, incorporating machine learning algorithms to optimize prediction model performance. Binary classifiers for each subtype were carefully validated using test data sets to ensure generalizability and minimize overfitting. Evaluation metrics extended beyond AUCs to include accuracy, precision, recall, and F1 scores, providing a comprehensive assessment of model reliability.
Notably, the integration of SWE parameters—such as the standard deviation of the lesion’s elastic modulus—highlighted the importance of tumor biomechanical heterogeneity in differentiating subtypes. Tumors exhibiting increased stiffness variability tend to correlate with aggressive phenotypes like HER2-overexpressing and triple-negative cancers. Similarly, CEUS-derived parameters reflecting microvascular flow dynamics enriched the predictive capacity by correlating with angiogenic profiles associated with specific molecular subtypes.
While these findings are promising, the researchers acknowledge the need for further validation in larger, multi-center cohorts to consolidate the clinical utility of the proposed models. Expanding the feature set to include emerging ultrasound modalities and advanced image analysis techniques, such as radiomics and deep learning, may further enhance predictive accuracy. Additionally, integration with other non-invasive biomarkers like circulating tumor DNA could create synergistic diagnostic frameworks.
In conclusion, this pioneering study marks a significant leap toward non-invasive, precision-guided management of breast cancer. By harnessing the complementary strengths of multimodal ultrasound and clinical features, clinicians are now closer to accurately predicting molecular subtypes preoperatively, facilitating tailored therapeutic interventions. This approach has the potential to improve patient outcomes, reduce unnecessary treatments, and optimize healthcare resources.
As breast cancer heterogeneity continues to challenge oncologists worldwide, such technological innovations exemplify how advanced imaging and data science converge to transform cancer care. The capability to decode molecular signatures through ultrasound imaging underscores a new frontier in personalized medicine—one where treatment strategies are as dynamic and multifaceted as the tumors themselves.
The promising results from this research herald a future where ultrasound-guided precision oncology becomes routine, empowering clinicians with rapid, reliable, and non-invasive tools to unravel the complex biological landscape of breast cancer at the patient’s bedside.
Subject of Research: Prediction of breast cancer molecular subtypes using multimodal ultrasound imaging and clinical features.
Article Title: Prediction models of breast cancer molecular subtypes based on multimodal ultrasound and clinical features.
Article References:
Li, H., Zhang, Ct., Shao, Hg. et al. Prediction models of breast cancer molecular subtypes based on multimodal ultrasound and clinical features. BMC Cancer 25, 886 (2025). https://doi.org/10.1186/s12885-025-14233-6
Image Credits: Scienmag.com
DOI: https://doi.org/10.1186/s12885-025-14233-6
Tags: breast cancer subtype predictioncontrast-enhanced ultrasound benefitsearly breast cancer detectionHER2-overexpressing breast cancerimaging-based diagnostic toolsluminal A breast cancerluminal B breast cancermultimodal ultrasound imagingnon-invasive molecular profilingpersonalized breast cancer treatmentshear wave elastography applicationstriple-negative breast cancer diagnosis
What's Your Reaction?
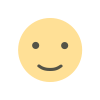
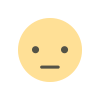
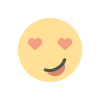
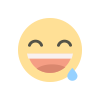
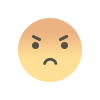
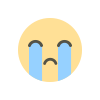
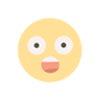