Big Data Analytics Transforming Food Industry Insights
In recent years, the intersection of big data analytics and food safety has emerged as a groundbreaking frontier with the potential to revolutionize how the industry detects spoilage and pathogens. The complexities inherent in food supply chains, combined with the imperative to ensure consumer safety, have driven researchers and industry players to explore innovative solutions. […]

In recent years, the intersection of big data analytics and food safety has emerged as a groundbreaking frontier with the potential to revolutionize how the industry detects spoilage and pathogens. The complexities inherent in food supply chains, combined with the imperative to ensure consumer safety, have driven researchers and industry players to explore innovative solutions. Central to these advancements is the integration of Big Data Analytics (BDA) with the Internet of Things (IoT), creating an interconnected network capable of gathering granular real-time data along the entirety of the food production process. This technological synergy promises unprecedented insights into spoilage patterns and contamination risks, setting the stage for a transformative shift in food safety monitoring.
The deployment of IoT devices throughout the supply chain introduces a new paradigm for data acquisition. Sensors embedded at various nodes, ranging from farm fields to processing plants and retail shelves, continuously collect a plethora of variables such as temperature fluctuations, humidity levels, microbial presence, and chemical indicators. This continuous data stream enables traceability with a granularity previously unattainable, empowering stakeholders to identify contamination events promptly. The richness and immediacy of this data enable a proactive response mechanism, fundamentally shifting the paradigm from reactive to preventive food safety management.
Despite the promising capabilities of IoT-enhanced big data systems, the practicality of widescale implementation demands careful attention to cost-effectiveness. Historically, the sophistication of detection equipment and the intricacies involved in data preprocessing have posed significant barriers, particularly for small and medium-sized enterprises (SMEs) within the food industry. Reducing the economic burden through innovative sensor designs and streamlined preprocessing workflows is not just an operational consideration but a strategic imperative to democratize access to these transformative technologies. More accessible systems can foster widespread adoption, thereby amplifying the impact of big data-driven safety protocols across diverse industry segments.
Beyond hardware integration, software advancements, particularly in machine learning, play a crucial role in translating raw data into actionable intelligence. Machine learning models tasked with recognizing spoilage or pathogen signals must contend with the inherent variability of biological systems and environmental influences. To maintain their efficacy over time, these models require continuous refinement and retraining with incoming data. This dynamic updating process ensures adaptability and resilience, allowing the predictive frameworks to evolve alongside changing conditions and emerging challenges in food safety.
Integral to this evolution is the standardized protocol for data collection, processing, and analysis. Without harmonized methodologies, the comparability and reliability of data across different studies and industrial applications remain compromised. Establishing standardized processes for capturing and interpreting data ensures data quality and fosters interoperability among disparate systems. Such standardization is foundational to building trust in data-driven decision-making tools, enabling regulators and industry leaders alike to rely on these systems for critical safety assessments.
The convergence of these technological and methodological advances holds promise for addressing longstanding challenges in the food industry, including waste reduction and enhanced public health protection. By facilitating early detection of spoilage and contamination, big data analytics can minimize the distribution of compromised products and reduce unnecessary food wastage. Moreover, the enhanced precision in pathogen detection contributes directly to consumer safety, potentially averting foodborne illness outbreaks that pose significant health and economic burdens.
However, translating laboratory-scale innovations into commercially viable solutions remains fraught with challenges. Rapid detection methods that show promise in controlled research environments often struggle to scale due to technical limitations, cost, and integration complexities within existing supply chain infrastructures. Overcoming these hurdles necessitates a concerted effort encompassing cross-disciplinary collaboration, investment in scalable technologies, and robust validation frameworks.
The architecture of big data applications in this domain extends beyond mere data aggregation. Sophisticated analytics platforms harness advanced algorithms to sift through vast datasets, extracting subtle patterns that may herald the onset of spoilage or contamination. These insights facilitate not only detection but also predictive modeling, enabling the anticipation of risks before visible signs manifest. Such foresight equips stakeholders to implement timely interventions, thus safeguarding product integrity and consumer health.
Furthermore, the role of environmental parameters must not be underestimated. Variability arising from differences in geographic conditions, climate, and seasonal changes imposes additional complexity on detection systems. Data-driven models must account for these factors to reduce false positives and negatives. Incorporating environmental context into analytical frameworks improves the robustness and accuracy of predictions, tailoring detection strategies to the nuanced realities of diverse food production ecosystems.
The emerging landscape also highlights the importance of interoperability between IoT devices and analytics platforms. Seamless data flow from sensor nodes through cloud-based processing units to end-user interfaces is critical for real-time monitoring and decision-making. Standardized communication protocols and secure data transmission channels underpin the integrity and usability of the system, protecting sensitive information while facilitating rapid responsiveness.
From a regulatory perspective, the integration of big data analytics into food safety regimes introduces new considerations. Regulatory bodies must grapple with establishing guidelines for data governance, validation of machine learning models, and the acceptable thresholds for detection alerts. Harmonizing regulatory standards across jurisdictions will be integral to supporting innovation without compromising public safety, enabling global adoption of advanced big data solutions.
In parallel, ethical considerations surrounding data privacy and ownership cannot be overlooked. The proliferation of IoT devices and extensive data collection raise questions about proprietary rights and the protection of sensitive commercial information. Transparent policies and ethical frameworks must accompany technological advancements to ensure stakeholder confidence and compliance.
Educational initiatives targeting industry stakeholders are equally vital. Empowering producers, processors, and retailers with the knowledge to interpret analytics outputs and integrate findings into operational decisions will determine the practical efficacy of these technologies. Training programs and user-friendly interfaces foster engagement and encourage the transition from traditional to data-driven approaches.
Looking forward, ongoing innovation and cross-sector collaboration will drive the maturation of big data analytics in the food safety landscape. The synthesis of evolving sensor technologies, adaptive machine learning algorithms, and robust data infrastructure promises to unlock new dimensions in food quality assurance. As these systems mature, they will not only mitigate risks but also catalyze efficiencies and sustainability within the global food ecosystem.
Ultimately, harnessing the full potential of big data in food spoilage and pathogen detection stands as a pivotal endeavor requiring sustained commitment. Addressing current limitations, such as cost barriers, data variability, and model refinement processes, will be instrumental in translating research breakthroughs into real-world impact. By embracing best practices and leveraging cutting-edge technologies, the food industry can transition toward a future marked by enhanced safety, reduced waste, and greater consumer trust.
Subject of Research: Big data analytics applications in food spoilage and pathogen detection
Article Title: Big data analytics in food industry: a state-of-the-art literature review
Article References:
Siddique, A., Gupta, A., Sawyer, J.T. et al. Big data analytics in food industry: a state-of-the-art literature review. npj Sci Food 9, 36 (2025). https://doi.org/10.1038/s41538-025-00394-y
Image Credits: AI Generated
Tags: agriculture and technology integrationbig data analytics in food safetydata-driven insights in food safetyfood contamination risk managementfood industry transformation through technologyinnovative solutions for food safetyinterconnected food production networksIoT in food supply chainspreventive food safety strategiesreal-time data collection in food industrysensors in food monitoringspoilage detection technologies
What's Your Reaction?
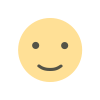
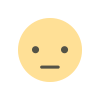
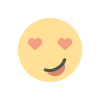
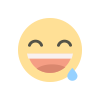
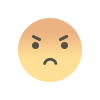
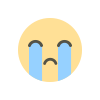
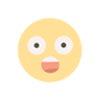