AI-Driven ECG Algorithm Excels in Early Heart Failure Detection in Kenya
In a groundbreaking study presented at the Heart Failure 2025 Congress, researchers unveiled a novel approach utilizing artificial intelligence (AI) to detect early signs of heart failure in Kenyan populations through routine electrocardiograms (ECGs). This development heralds a potentially transformative shift in cardiovascular diagnostics, especially in settings where traditional imaging modalities like echocardiography remain sparse […]

In a groundbreaking study presented at the Heart Failure 2025 Congress, researchers unveiled a novel approach utilizing artificial intelligence (AI) to detect early signs of heart failure in Kenyan populations through routine electrocardiograms (ECGs). This development heralds a potentially transformative shift in cardiovascular diagnostics, especially in settings where traditional imaging modalities like echocardiography remain sparse and costly. The study’s findings offer a promising vision for scalable, low-cost screening interventions in resource-limited regions struggling with high cardiovascular disease burdens.
Heart failure remains a critical health challenge globally, with a particularly acute impact in Sub-Saharan Africa. Unlike many high-income countries where heart failure predominantly affects the elderly, in regions like Kenya, patients are often younger yet experience disproportionately poorer outcomes. The availability and accessibility of diagnostic tools play a pivotal role in these outcomes. While echocardiography remains the gold standard for assessing left ventricular systolic dysfunction (LVSD)—a key marker and precursor of heart failure—it requires expensive equipment and specialized expertise, both scarce commodities in many African healthcare settings.
The current study, spearheaded by Dr. Ambarish Pandey from the University of Texas Southwestern Medical Center, explores an innovative solution: leveraging an AI-enabled, ECG-based algorithm to detect LVSD early and accurately. Traditional ECGs offer a wealth of cardiac electrical information, but interpreting subtle patterns indicative of LVSD demands advanced analytical frameworks. The team employed validated AI software — AiTiALVSD — developed by Medical AI Co in Seoul, South Korea. This deep learning algorithm was trained to estimate the likelihood of LVSD based on 12-lead ECG data, establishing a probability threshold (>0.097) to flag high-risk individuals.
Conducted as a prospective, cross-sectional multicentre screening involving nearly 6,000 adult patients across eight Kenyan healthcare facilities, the study meticulously gauged cardiovascular risk profiles. Participants’ risk was stratified using the well-established Framingham Risk Score (FRS) and existing histories of cardiovascular disease (CVD), enabling researchers to contextualize the AI findings within traditional clinical risk frameworks. Intriguingly, two-thirds of the study cohort were female, and the average age hovered at 55 years, underscoring the relative youth of the affected demographic.
The AI-ECG algorithm revealed a substantial prevalence of LVSD—18.3% across all participants—with significantly higher rates among those with elevated cardiovascular risk profiles. Specifically, individuals with a high FRS exhibited a 22.9% prevalence, while those with established CVD demonstrated a striking 32% occurrence. Conversely, participants classified as low-risk had a prevalence below 10%, reinforcing the algorithm’s sensitivity to clinically meaningful distinctions in cardiac function.
To rigorously evaluate the AI tool’s diagnostic accuracy, a subset of over 1,400 participants underwent concomitant echocardiographic evaluation. Echocardiography-confirmed LVSD appeared in 14.1% of this subgroup, serving as the benchmark for validation. Performance metrics for the AI algorithm were notably impressive: sensitivity reached 95.6%, highlighting its ability to correctly identify nearly all true positive cases, while specificity was 79.4%, reflecting a robust capacity to exclude false positives. Most striking was the negative predictive value of 99.1%, suggesting that a negative AI-ECG result virtually ruled out significant LVSD.
These findings not only validate the algorithm’s clinical utility but also underscore the transformative potential of AI in democratizing access to cardiovascular diagnostics. In regions like Sub-Saharan Africa, where echocardiography is frequently unavailable outside major urban centers, scalable contactless diagnostics through routine ECG examinations could revolutionize early detection pathways and subsequently improve patient prognoses.
Dr. Bernard Samia, President of the Kenya Cardiac Society and senior author of the study, emphasized the real-world implications: “Our results demonstrate AI-enabled ECG screening as a cost-effective and scalable approach to identify individuals at risk of left ventricular dysfunction, a critical antecedent of heart failure. Such tools can bridge the gap created by limited healthcare infrastructure while facilitating timely interventions.”
The research team envisions expanding this pioneering work across multiple African countries, aiming to leverage AI-enhanced ECG screening as a foundational step in comprehensive heart failure prevention programs. Future investigations will focus on whether early identification of LVSD via AI leads to increased initiation of evidence-based therapies, which could significantly alter disease trajectories and reduce cardiovascular mortality rates.
Technically, the AI algorithm utilizes deep convolutional neural networks trained on vast datasets to detect nuanced ECG features invisible to the human eye yet predictive of impaired myocardial function. This methodology reflects a broader trend in precision cardiology, where machine learning models supplement clinical acumen to enhance diagnostic accuracy, stratify risk, and tailor interventions.
However, the deployment of AI-ECG in resource-limited settings poses pragmatic challenges beyond algorithmic performance. Ensuring widespread availability of quality ECG devices, training healthcare personnel in AI interpretation, establishing referral pathways for confirmatory testing and treatment, and addressing potential ethical and data privacy concerns are critical next steps.
This study marks a compelling example of collaborative, technology-driven solutions addressing global health inequalities in cardiovascular disease. By combining cutting-edge AI techniques with accessible diagnostic modalities, the research opens new frontiers for early disease detection in populations historically underserved by conventional healthcare models.
In summary, the AI-based ECG screening approach demonstrated in Kenya embodies a scalable, cost-efficient, and high-performance strategy to detect left ventricular systolic dysfunction in at-risk individuals. It presents a vital opportunity to reshape heart failure screening and prophylaxis in Sub-Saharan Africa, paving the way for broader regional and global adoption.
Subject of Research: Left ventricular systolic dysfunction detection using AI-enabled electrocardiography
Article Title: Implementing an AI-ECG Based Algorithm to Screen for Left Ventricular Dysfunction in Kenya: A Prospective Cohort Study
News Publication Date: 17 May 2025
Web References:
https://esc365.escardio.org/Heart-Failure/sessions/13792-late-breaking-science-diagnostic-methods-and-assessment
References:
1. ‘Implementing an AI-ECG based algorithm to screen for left ventricular dysfunction in Kenya: a prospective cohort study’ (Heart Failure 2025)
2. Siddikatou D, Linwa EMM, Ndobo V, et al. Heart failure outcomes in Sub-Saharan Africa: a scoping review. BMC Cardiovasc Disord. 2025;25:302.
3. Kwon JM, Kim KH, Jeon KH, et al. Development and validation of deep-learning algorithm for electrocardiography-based heart failure identification. Korean Circ J. 2019;49:629–639.
Keywords:
AI-ECG, left ventricular systolic dysfunction, heart failure, Sub-Saharan Africa, Kenya, cardiovascular risk, Framingham Risk Score, echocardiography, deep learning, machine learning, cardiovascular diagnostics, resource-limited settings
Tags: accessibility of diagnostic toolsAI algorithm for LVSD detectionAI in cardiovascular diagnosticsAI-driven healthcare solutionsearly heart failure detection in Kenyaelectrocardiogram (ECG) technologyhealthcare innovation in Sub-Saharan AfricaHeart Failure 2025 Congress findingslow-cost heart disease screeningresource-limited medical interventionstransformative heart failure diagnosisyouth heart failure outcomes
What's Your Reaction?
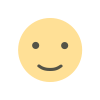
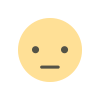
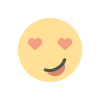
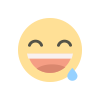
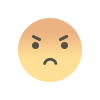
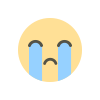
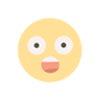