AI Tool Analyzes Facial Images to Estimate Biological Age and Forecast Cancer Prognosis
In a groundbreaking advancement at the intersection of artificial intelligence and medicine, researchers at Mass General Brigham have developed an innovative deep learning system named FaceAge that can predict biological age from facial photographs. This development goes beyond mere chronological age, offering a nuanced and clinically significant metric that correlates with patient health status and […]

In a groundbreaking advancement at the intersection of artificial intelligence and medicine, researchers at Mass General Brigham have developed an innovative deep learning system named FaceAge that can predict biological age from facial photographs. This development goes beyond mere chronological age, offering a nuanced and clinically significant metric that correlates with patient health status and survival prospects—especially for those battling cancer. The study, recently published in The Lancet Digital Health, demonstrates how facial features captured in an image reveal deep biological signals that relate to an individual’s aging process and can serve as predictive markers for clinical outcomes in oncological care.
FaceAge employs sophisticated deep learning algorithms, a subset of artificial intelligence that excels at recognizing complex patterns within images, to analyze subtle features within a patient’s face. The model was trained on an extensive dataset comprising nearly 59,000 photographs of presumed healthy individuals, sourced from publicly available datasets, to learn normative aging patterns. This foundational training makes the model sensitive to variances beyond chronological time, identifying aging markers that may signal underlying physiological or pathological changes invisible to the naked eye.
Following initial training, FaceAge was rigorously tested on a cohort of over 6,000 cancer patients from two distinct medical centers, utilizing photographs routinely taken at the outset of radiotherapy treatment. The results revealed a striking trend: cancer patients consistently exhibited a biological age—an inferred FaceAge—that was roughly five years older than their actual chronological age. This disparity suggests that their physical appearance encodes the toll that cancer and perhaps its treatments impose on the body’s biological systems.
Importantly, the researchers discovered that an elevated FaceAge correlated strongly with worse overall survival outcomes across multiple cancer types. The predictive power of FaceAge remained robust even after adjusting for traditional prognostic factors including chronological age, sex, and cancer classification, underscoring its value as an independent biomarker. Notably, patients with FaceAge estimates indicating they appeared older than 85 years faced particularly poor prognoses, making FaceAge a potentially critical tool in patient stratification and personalized treatment planning.
Predicting survival time, particularly in terminal conditions, remains a profound challenge in clinical oncology due to the complex interplay of patient variables. The Mass General Brigham team engaged ten clinicians and researchers to retrospectively evaluate short-term life expectancy from 100 patient photos undergoing palliative radiotherapy. Despite their expertise and access to clinical data, clinician predictions were only marginally better than chance. However, when clinicians were augmented with FaceAge metrics, their prognostic accuracy improved significantly, demonstrating how AI-derived biological age could complement clinical intuition and reduce subjectivity inherent in traditional assessments.
The implications of FaceAge extend beyond a single disease or even oncology itself. Facial morphology and appearance can serve as visible readouts of an individual’s complex biological aging process, which is influenced by myriad factors including genetics, environment, and disease burden. The ability to decode this information through a simple photograph opens avenues for biomarker discovery that leverage noninvasive, ubiquitous data sources. This approach holds promise not only for predicting cancer outcomes but also for early detection of chronic illnesses and monitoring general health trajectories over time.
While FaceAge’s performance is compelling, the researchers emphasize that further validation across diverse populations, healthcare settings, and disease stages is essential before clinical deployment. Ongoing studies aim to evaluate the system’s robustness in different demographic and geographic contexts, track longitudinal changes in FaceAge during disease progression or recovery, and compare its reliability against confounders such as cosmetic interventions like plastic surgery or makeup.
Technical innovation also includes the integration of FaceAge into clinical workflows in a manner that respects ethical considerations and patient privacy. The research team advocates for incorporating regulatory frameworks and transparency about algorithm limitations, to ensure that this emerging technology serves as a tool to support, rather than replace, physician judgment. Ultimately, FaceAge could revolutionize how clinicians assess biological aging and tailor individualized care pathways, making treatment more precise by integrating objective physiological metrics derived from facial imaging.
Co-senior and corresponding author Hugo Aerts, PhD, highlights the unique power of this approach: “A simple selfie contains layers of biological information that have been traditionally overlooked. This method transforms everyday data into crucial clinical insights that could refine prognostication and patient management.” Meanwhile, co-senior author Ray Mak, MD, envisions that FaceAge and similar tools could become cornerstones for early disease detection across aging-related conditions, provided their development proceeds with rigorous scientific standards and ethical oversight.
The potential applications of FaceAge also intersect with population health, as aging faces are a universal human attribute. By capturing and quantifying aging trajectories at the individual level, this technology could contribute to a broader understanding of how chronic diseases accelerate biological aging, potentially guiding public health interventions and resource allocation. Moving forward, FaceAge’s developers seek to integrate multi-modal data sources, incorporating genomic, metabolic, and lifestyle information alongside facial imaging to create comprehensive, personalized health profiles.
This research underscores a transformative moment in medicine, where artificial intelligence translates visual data into meaningful biological markers. The capacity to decode aging and prognosis from facial photographs may redefine patient evaluation, prognostication, and care personalization. As digital health technologies continue to evolve, FaceAge exemplifies the power of combining computational modeling with clinical insight, paving the way for more sophisticated, accessible, and objective health assessments in the near future.
Subject of Research: People
Article Title: FaceAge, a deep learning system to estimate biological age from face photographs to improve prognostication: a model development and validation study
News Publication Date: 8-May-2025
Web References:
Mass General Brigham
The Lancet Digital Health Article
DOI Link
References:
Bontempi, et al. “Decoding biological age from face photographs using deep learning.” The Lancet Digital Health, DOI: 10.1016/j.landig.2025.03.002
Image Credits: Mass General Brigham
Keywords: Artificial intelligence, Life expectancy, Cancer, Aging populations
Tags: aging process analysis through AIAI facial recognition technologybiological age estimationcancer prognosis predictionclinical outcomes forecastingdeep learning in healthcarefacial image analysis for healthinnovative healthcare solutionsmachine learning in medicineMass General Brigham researchoncological care advancementspredictive markers in cancer treatment
What's Your Reaction?
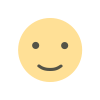
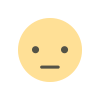
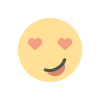
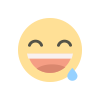
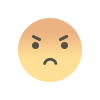
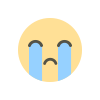
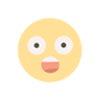