Unsupervised Learning Reveals Liver Cancer Immune Profiles
In the relentless pursuit to unravel the complexities of liver cancer, a recent study harnesses the power of unsupervised machine learning to redefine how hepatocellular carcinoma (HCC) is understood and classified. HCC remains the most prevalent form of liver cancer worldwide, posing formidable challenges due to its intricate tumour microenvironment (TME) and heterogeneous nature. This […]

In the relentless pursuit to unravel the complexities of liver cancer, a recent study harnesses the power of unsupervised machine learning to redefine how hepatocellular carcinoma (HCC) is understood and classified. HCC remains the most prevalent form of liver cancer worldwide, posing formidable challenges due to its intricate tumour microenvironment (TME) and heterogeneous nature. This groundbreaking research offers a fresh perspective, employing advanced computational methods to dissect HCC’s molecular landscape and immune milieu, potentially paving the way for more precise prognostic markers and therapeutic avenues.
At the heart of this study lies the application of unsupervised machine learning techniques, a class of algorithms designed to identify hidden patterns in data without predefined labels. The researchers utilized three distinct methodologies: agglomerative hierarchical clustering, Multi-Omics Factor Analysis coupled with the K-means++ algorithm, and an autoencoder integrated with K-means++. Together, these approaches enabled the stratification of HCC patient samples based on their gene expression profiles gleaned from microarray data, marking a significant stride toward personalized medicine.
Agglomerative hierarchical clustering, a bottom-up approach, iteratively merges similar data points, creating a dendrogram that represents the nested grouping of samples. This method excels at revealing intrinsic structure without requiring a preset number of clusters. Meanwhile, Multi-Omics Factor Analysis extends beyond traditional single-data-type analyses by integrating multiple layers of omics information to capture latent factors affecting tumor biology, which, when combined with the refined K-means++, further enhances clustering accuracy. The use of autoencoders, a form of neural network tailored for unsupervised feature learning, allows the compression of complex gene expression data, facilitating discrimination of subtle but biologically meaningful differences among tumour samples.
Upon the derivation of patient clusters, the research team delved deeper into the tumour microenvironment by implementing immune deconvolution algorithms. These computational techniques estimate the proportions of various infiltrating immune cell populations within tumours, providing vital insights into immune landscape heterogeneity. Understanding which immune components prevail in distinct HCC subtypes can illuminate mechanisms of immune evasion and response, potentially informing immunotherapeutic strategies.
Strikingly, the analysis uncovered a set of thirteen genes consistently influential in defining HCC subtypes across both primary and validation cohorts. Among these, three genes—TOP2A, DCN, and MT1E—emerged as significant prognosticators associated with patient survival and cancer recurrence. TOP2A, long implicated in cellular proliferation and DNA replication, corroborates previous findings relating its overexpression to aggressive tumour behavior. MT1E, part of the metallothionein family, is known for its role in metal ion binding and oxidative stress modulation, suggesting nuanced involvement in tumour progression.
Most noteworthy is the identification of DCN (Decorin), a well-characterized tumour suppressor gene. Its expression correlated consistently with improved patient survival, highlighting its potential as a key modulator within the HCC microenvironment. Decorin’s biological functions extend to influencing extracellular matrix composition and interacting with growth factor signaling pathways, which may contribute to its anti-tumour capabilities by orchestrating a microenvironment hostile to cancer proliferation and facilitating anti-tumour immune responses.
The study’s findings reinforce the concept that HCC heterogeneity is underpinned not only by genetic variability but also by the complex interplay within the tumour microenvironment. By successfully stratifying patient populations using conserved gene signatures, the research offers a robust framework for future clinical applications. Such stratification can refine risk assessment, guide treatment decisions, and identify candidates who may benefit from emerging immunotherapies.
While gene expression profiling provides invaluable insights, the authors highlight the necessity to explore additional factors influencing the TME. Elements such as the tumour-associated microbiome and stromal cell dynamics remain largely enigmatic but are believed to substantially affect tumour behavior and therapeutic response. Future investigations incorporating these dimensions could unveil novel biomarkers and therapeutic targets, addressing the current gaps in understanding HCC progression.
From a translational perspective, the integration of unsupervised machine learning in cancer genomics exemplifies the paradigm shift toward data-driven oncology. This approach circumvents the limitations of supervised learning, which relies on existing clinical labels that may not capture underlying biological complexities. By uncovering new molecular subtypes, researchers can better comprehend the disease’s multifaceted nature and tailor interventions accordingly.
Moreover, the immune deconvolution component underscores the growing recognition of the immune system’s pivotal role in cancer control. HCC, often arising in chronic inflammatory contexts like cirrhosis or viral hepatitis, presents a particularly challenging immune landscape. Detailed immune cell profiling embedded within molecular subtypes offers a compelling route to identify immune evasion patterns and opportunities for immunomodulation.
The robustness of the study is amplified by its validation across independent datasets, ensuring that the identified gene signatures and clustering strategies are reproducible and generalizable. This reproducibility is critical for any proposed biomarker or stratification schema to transition into clinical practice, where variability across patient populations can dilute efficacy.
In essence, this research represents a significant leap in leveraging computational biology and immunology to decode the intricate heterogeneity of hepatocellular carcinoma. It converges cutting-edge machine learning techniques with molecular oncology to unravel the complex biology of liver cancer, offering hope for more personalized and effective management strategies.
As the global burden of liver cancer continues to rise, innovations such as these provide a beacon of hope. They exemplify how integrating technology, biology, and clinical insight can transcend traditional research boundaries. Ultimately, understanding HCC at such a granular level is crucial to surmounting its therapeutic challenges and improving patient outcomes in the years ahead.
Subject of Research: Hepatocellular carcinoma stratification and tumour microenvironment analysis using unsupervised machine learning and immune deconvolution techniques.
Article Title: Unsupervised machine learning-based stratification and immune deconvolution of liver hepatocellular carcinoma
Article References:
Reierson, M.M., Acharjee, A. Unsupervised machine learning-based stratification and immune deconvolution of liver hepatocellular carcinoma.
BMC Cancer 25, 853 (2025). https://doi.org/10.1186/s12885-025-14242-5
Image Credits: Scienmag.com
DOI: https://doi.org/10.1186/s12885-025-14242-5
Keywords: Hepatocellular carcinoma, unsupervised machine learning, tumour microenvironment, immune deconvolution, gene expression profiling, tumour heterogeneity, Decorin, biomarker discovery
Tags: advanced computational methods in oncologyautoencoder applications in bioinformaticsgene expression profiling in HCChepatocellular carcinoma classificationhierarchical clustering in cancer researchimmune profiles in liver cancermolecular landscape of HCCMulti-Omics Factor Analysis techniquespersonalized medicine for liver cancertherapeutic avenues for liver cancertumor microenvironment analysisunsupervised machine learning in liver cancer
What's Your Reaction?
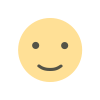
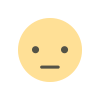
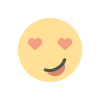
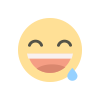
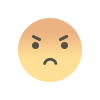
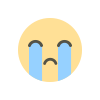
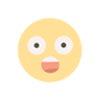