Unlocking membrane-based He/H₂ separation with AI
Technological advancement and data proliferation have deemed artificial intelligence (AI)-driven innovation as growth opportunity to the development of breakthrough materials for special applications, especially in the field of gas separation. One of the main challenges associated with this process is the extremely close kinetic diameters of the two gas molecules, resulting in low membrane selectivity. […]
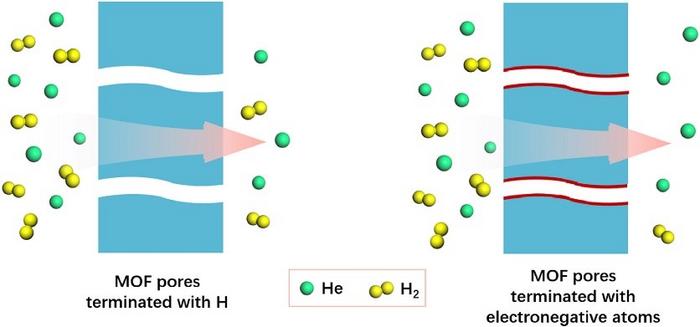
Technological advancement and data proliferation have deemed artificial intelligence (AI)-driven innovation as growth opportunity to the development of breakthrough materials for special applications, especially in the field of gas separation. One of the main challenges associated with this process is the extremely close kinetic diameters of the two gas molecules, resulting in low membrane selectivity.
Credit: Zhang. S. et al.,
Technological advancement and data proliferation have deemed artificial intelligence (AI)-driven innovation as growth opportunity to the development of breakthrough materials for special applications, especially in the field of gas separation. One of the main challenges associated with this process is the extremely close kinetic diameters of the two gas molecules, resulting in low membrane selectivity.
In a study published in the KeAi Green Chemical Engineering, a group of researchers from China came up with a novel approach to explore materials with enhanced helium extraction efficiency—with the aid of AI. In particular, the researchers investigated structure-performance relationships, elucidated separation mechanisms and identified crucial factors influencing the separation performance to design metal-organic framework (MOF)-based membranes. The pore limiting diameter (PLD) and void fraction (φ) were revealed as the most important physical features for determining the membrane selectivity and He permeability, respectively.
“Traditional material development faces constraints, but AI is revolutionizing the field,” says Zhengqing Zhang, lead investigator of the study “Our approach not only reveals hidden mechanisms but also uncovers new insights.”
The team hopes that their results would encourage scientists to continue investigating the intersection of AI and material science, opening doors to unprecedented technological advancements.
###
Contact the author: Zhang Zhengqing, State Key Laboratory of Separation Membranes and Membrane Processes&School of Chemical Engineering and Technology, Tiangong University, Tianjin 300387, P. R. China, zhangzhengqing@tiangong.edu.cn
The publisher KeAi was established by Elsevier and China Science Publishing & Media Ltd to unfold quality research globally. In 2013, our focus shifted to open access publishing. We now proudly publish more than 100 world-class, open access, English language journals, spanning all scientific disciplines. Many of these are titles we publish in partnership with prestigious societies and academic institutions, such as the National Natural Science Foundation of China (NSFC).
Journal
Green Chemical Engineering
DOI
10.1016/j.gce.2024.01.005
Article Title
Machine learning aided investigation on the structure-performance correlation of MOF for membrane-based He/H₂ separation
COI Statement
The authors declare that they have no known competing financial interests or personal relationships that could have appeared to influence the work reported in this paper.
What's Your Reaction?
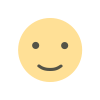
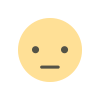
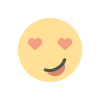
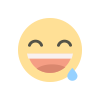
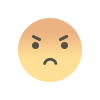
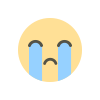
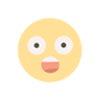