Unequal Testing Skews Vaccine Effectiveness Estimates
In the ever-evolving landscape of infectious diseases, particularly under the shadow of a global pandemic, accurate estimation of vaccine effectiveness remains pivotal for shaping public health policies and guiding individual behaviors. A recent groundbreaking simulation study, published in Nature Communications, delves into an often-overlooked factor that can drastically skew such estimations—the unequal rates of viral […]

In the ever-evolving landscape of infectious diseases, particularly under the shadow of a global pandemic, accurate estimation of vaccine effectiveness remains pivotal for shaping public health policies and guiding individual behaviors. A recent groundbreaking simulation study, published in Nature Communications, delves into an often-overlooked factor that can drastically skew such estimations—the unequal rates of viral testing among different population subgroups. This research provides fresh insights into the biases introduced in vaccine effectiveness metrics when testing behaviors and access vary across vaccinated and unvaccinated cohorts, as well as across different study designs used to monitor vaccine performance.
The crux of the study is an exploration of how discrepancies in testing rates systematically influence the calculated effectiveness of vaccines in real-world settings. Vaccines, inherently designed to reduce the risk of infection and severe disease, are commonly evaluated through observational studies that rely heavily on detected infections confirmed by diagnostic tests. However, when the likelihood of seeking or undergoing testing is not uniform—either due to behavioral differences, healthcare access disparities, or policy-driven testing mandates—these observational estimates can become misleading.
To interrogate this problem, the authors employed advanced simulation models replicating two commonly used epidemiological study designs in vaccine effectiveness research: the cohort study and the test-negative case-control study. Each design carries unique assumptions and sampling frameworks that can be vulnerable to varying degrees of bias under unequal testing conditions. By simulating how infection probabilities, vaccination status, and testing propensities interact, the research offers a nuanced quantification of how vaccine effectiveness estimates deviate from true values.
Cohort studies, following large groups of individuals differing by vaccination status over time, tend to count observed infections as outcomes. They require precise knowledge or consistent testing to correctly classify cases. The simulation showed that if vaccinated individuals are less likely to seek testing due to perceived lower risk or milder symptoms, infections among the vaccinated may be underreported, artificially inflating the apparent effectiveness of the vaccine. Conversely, if unvaccinated individuals face barriers to testing, their infection rates may be underestimated, leading to an undervalued effectiveness estimate.
On the other side, the test-negative design, which enrolls individuals presenting for testing and compares vaccination rates among positives versus negatives, is often presumed to mitigate bias from healthcare-seeking behavior variations. Yet, the simulation revealed that even these designs are not immune. When testing behaviors differ between vaccinated and unvaccinated people, the selection of who gets tested skews the representation of cases and controls, embedding bias into vaccine effectiveness calculations. For instance, if vaccinated persons with mild symptoms are less likely to get tested compared to unvaccinated individuals, the test-negative approach may overestimate vaccine protection.
An expansion of the study’s findings shows intricate interactions between severity of symptoms, test availability, and behavioral factors affecting testing patterns. The authors simulated scenarios where testing disparities were driven not only by vaccination status but also by symptom severity, sociodemographic variables, and temporal shifts in public health policy. Such complexity mirrors the dynamic characteristics observed during real-world infectious disease outbreaks.
Importantly, the study underscores that discernible biases may persist or even amplify as vaccination coverage increases and epidemic curves flatten, challenging the simplistic assumption that more data automatically equate to greater accuracy in effectiveness estimates. Under these conditions, unequal testing can create artifacts in surveillance data, giving rise to false narratives about waning immunity or vaccine failure that may influence public perception and vaccination uptake detrimentally.
To mitigate these biases, the authors suggest methodological adaptations including enhanced data collection on testing motives and timing, stratification by testing propensity, and incorporation of sensitivity analyses that model plausible testing behavior scenarios. Advanced statistical techniques that adjust for differential misclassification and selection bias are also highlighted as promising avenues to refine observational estimates.
Beyond methodological reforms, the research calls for cohesive public health strategies to promote equitable access and utilization of diagnostic testing. Ensuring that people across vaccination statuses and demographic groups have similar incentives and opportunities to test when symptomatic can reduce disparities in case detection, lending greater validity to vaccine effectiveness assessments.
This study, by integrating detailed statistical simulations with practical epidemiological concerns, illuminates a blind spot in current vaccine research, particularly relevant in the context of emerging variants and booster vaccination campaigns. The insights gathered have profound implications for interpreting ongoing data streams and for the design of future vaccine effectiveness studies in the face of evolving testing landscapes.
Moreover, the findings serve as a cautionary tale against overconfidence in observational data devoid of contextual understanding of testing behaviors. As vaccines continue to be our frontline defense against infectious threats, precision in evaluating their real-world impact constitutes a crucial foundation for maintaining public trust and optimizing vaccination policies.
Ultimately, this work advances the epidemiological discourse by foregrounding testing equity as an integral component in measuring and understanding vaccine performance. Its implications ripple across research methodology, health communication, and policy-making domains, advocating for a more holistic approach to pandemic evaluation beyond the vaccine alone.
As infectious disease epidemiology increasingly intersects with data science and behavioral insights, studies like this pave the way for more robust, accurate, and actionable public health intelligence. The challenges posed by unequal testing are emblematic of broader issues in surveillance and measurement, highlighting the need for interdisciplinary collaboration to disentangle complex real-world phenomena impacting the fight against infectious diseases.
In conclusion, the simulation conducted by Bodner, Wang, Kustra, and colleagues is a landmark contribution to the field, providing a compelling framework for understanding and correcting biases in vaccine effectiveness estimates due to unequal testing. Their research not only refines the methodological toolkit available to scientists but also elevates the discourse on how best to interpret and act upon real-world vaccine data, ensuring that scientific conclusions are grounded firmly in the realities faced by populations worldwide.
Subject of Research: Impact of Unequal Testing on Vaccine Effectiveness Estimates Across Two Study Designs
Article Title: Impact of unequal testing on vaccine effectiveness estimates across two study designs: a simulation study
Article References:
Bodner, K., Wang, L., Kustra, R. et al. Impact of unequal testing on vaccine effectiveness estimates across two study designs: a simulation study. Nat Commun 16, 4849 (2025). https://doi.org/10.1038/s41467-025-59768-1
Image Credits: AI Generated
Tags: COVID-19 vaccine effectiveness metricshealthcare access disparitiesinfectious disease vaccination strategiesobservational study biasespandemic response and vaccine evaluationpopulation subgroup analysispublic health policy implicationssimulation modeling in epidemiologytesting behavior variationsunequal testing rates impactsvaccine effectiveness researchvaccine performance monitoring
What's Your Reaction?
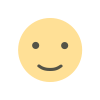
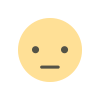
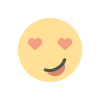
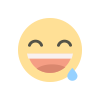
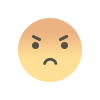
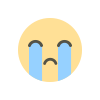
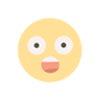