Transforming Visual Speech Representation: The Power of Audio-Guided Self-Supervised Learning
In a groundbreaking advancement in the field of machine learning and computer vision, researchers have made significant strides in understanding and modeling the complex interplay between visual communication and auditory signals in spoken language. The research spearheaded by a team led by Shuang Yang proposes a novel approach to disentangling visual speech representations from video […]

In a groundbreaking advancement in the field of machine learning and computer vision, researchers have made significant strides in understanding and modeling the complex interplay between visual communication and auditory signals in spoken language. The research spearheaded by a team led by Shuang Yang proposes a novel approach to disentangling visual speech representations from video data. Capturing the nuances of lip movements, head gestures, and facial expressions in synchrony with speech has significant implications for various applications ranging from lip reading to synthetic speech generation.
The primary challenge in this domain lies in isolating speech-relevant features from noise and distraction inherent in real-world video recordings. Factors such as varied lighting conditions, different camera angles, and background movements can introduce confounding variables that make it difficult for traditional models to effectively parse out the essential elements of visual speech. This difficulty can impede the progress of numerous speech-related tasks, including audio-visual speech separation and the production of realistic synthesized talking faces.
In their recent study published in the journal “Frontiers of Computer Science,” Yang and his team propose a two-branch self-supervised learning model. This model is adept at distinguishing between speech-relevant and speech-irrelevant components of visual speech data, thereby enhancing the effectiveness of learning algorithms in this domain. The model leverages a self-supervised approach, enabling it to learn from the intrinsic properties of the data rather than relying solely on external annotations, which often are scarce and expensive to obtain.
A distinguishing characteristic of the research is the observation that speech-relevant facial movements—those that align closely with the phonetic and prosodic elements of spoken language—occur with a higher frequency compared to speech-irrelevant movements. This insight allows the researchers to strategically guide the learning process, focusing on those moments when facial changes are most informative of speech content. The alignment of head motions and lip movements with audio signals presents an opportunity to create a model that effectively discerns the subtleties of visual speech production.
The proposed two-branch network architecture stands as a testament to the innovative thinking driving this research. The first branch of the network is dedicated to capturing high-frequency audio signals, which serve as a guiding force in the identification of speech-relevant visual cues. By funneling this auditory information into the learning process, the model can enhance its competency in recognizing when specific facial movements correspond with spoken words. Conversely, the second branch of the model is tempered with an information bottleneck to filter out lower-frequency movements that do not contribute significantly to the understanding of speech.
Over the course of the research, the team demonstrated the practical efficacy of their approach through rigorous testing on recognized visual speech datasets, such as LRW (Lip Reading in the Wild) and LRS2-BBC. The results yielded compelling qualitative and quantitative metrics that confirmed the advantages of their model over existing methodologies. The implication of their findings is substantial, paving the way for improved strategies in lip reading, facilitating communication for those with hearing impairments, and enhancing the realism of animated conversations in virtual environments.
Moreover, this research embodies an essential step towards understanding multimodal communication. As speech is inherently a multimodal phenomenon, relying solely on auditory or visual cues to interpret meaning often proves insufficient. The integration of visual speech representation learning with audio signals provides a more comprehensive understanding of how humans communicate.
Looking forward, the implications of this research extend beyond mere improvements in lip reading and visual speech models. Future explorations could delve into the intersection of this technology with broader aspects of artificial intelligence, exploring partnerships with psychological studies to examine how humans naturally discern speech from visual stimuli. The potential applications are far-reaching, including advancements in human-computer interaction, virtual reality experiences, and assistive technologies for communication-impaired individuals.
Furthermore, potential future works can focus on the exploration of explicit auxiliary tasks and constraints that go beyond reconstruction tasks to further enrich the model’s capacity to capture the intricacies of speech. As researchers continue to investigate the nature of visual speech signals, collaborations across disciplines could yield new insights into cognitive processes and communication theories.
The realm of disentangled visual speech representation learning is certainly an exciting area of exploration, promising innovative solutions in audiovisual communication technologies. Given the anticipated advancements, ongoing research in this space holds the potential to redefine the capabilities of machines in understanding and generating human language.
As we stand on the brink of this new frontier, it is crucial to consider the ethical ramifications of such technologies. Ensuring that advancements do not inadvertently perpetuate biases inherent in the training data is imperative. Continuous refinement of methodologies, alongside a commitment to ethical research practices, will help in fostering responsible advancements in the field.
In conclusion, the work led by Yang and his team stands as a significant contribution to the understanding of visual speech processing through a self-supervised learning framework. Their approach not only innovatively addresses existing challenges but also lays the groundwork for future research that could redefine how machines interpret visual language, ultimately bringing us closer to natural interactions between humans and artificial intelligences.
Subject of Research: Visual Speech Representation Learning
Article Title: Audio-guided self-supervised learning for disentangled visual speech representations
News Publication Date: 15-Dec-2024
Web References: Frontiers of Computer Science
References: Pursuant journal articles on related visual speech technologies
Image Credits: Dalu FENG, Shuang YANG, Shiguang SHAN, Xilin CHEN
Keywords
Disentangled Learning, Visual Speech Processing, Self-supervised Learning, Lip Reading, Machine Learning, Audio-visual Integration, Communication Technology.
What's Your Reaction?
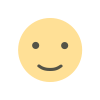
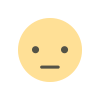
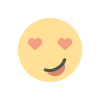
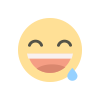
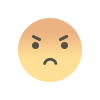
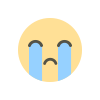
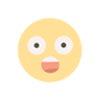