Transforming Ionic Thermoelectrics: How Machine Learning is Paving the Way
A revolutionary approach in the realm of ionic thermoelectric (i-TE) materials has emerged from a dedicated team of researchers who have harnessed the power of machine learning (ML) to significantly accelerate the discovery process. Traditional methodologies in this field have often relied on extensive trial-and-error experiments, which proved to be both time-consuming and complex. This […]

A revolutionary approach in the realm of ionic thermoelectric (i-TE) materials has emerged from a dedicated team of researchers who have harnessed the power of machine learning (ML) to significantly accelerate the discovery process. Traditional methodologies in this field have often relied on extensive trial-and-error experiments, which proved to be both time-consuming and complex. This new ML framework offers a fresh perspective, streamlining the quest for high-performance i-TE materials by providing rapid and accurate predictions of their essential properties, notably the Seebeck coefficient.
The Seebeck coefficient is a pivotal measure in the evaluation of thermoelectric materials, reflecting their ability to convert temperature differences into electrical energy. With ionic thermoelectric materials boasting Seebeck coefficients that frequently exceed 10 mV/K—far surpassing those of traditional electronic thermoelectric materials—there exists a significant opportunity for innovation in energy conversion technologies. Nonetheless, the path toward harnessing this potential has been obstructed by a multitude of challenges, including the diverse chemical makeup of ionic materials and the lack of comprehensive theoretical guidance to navigate this intricate landscape.
In response to these challenges, the research team adopted the use of the Simplified Molecular Input Line Entry System (SMILES) for encoding various molecular structures. This strategic choice allows their machine learning model to effectively process and analyze a broad array of i-TE material types. The model is built upon a carefully curated dataset comprising 51 distinct i-TE material samples and has achieved an astounding coefficient of determination (R²) of 0.98 during its testing phase. Such high accuracy not only enhances the reliability of predictions but also inspires confidence in the model’s applicability for future explorations in ionic thermoelectric materials.
Among the promising materials identified through this novel framework is a waterborne polyurethane-potassium iodide (WPU/KI) ionogel, which has shown exceptional qualities in terms of its Seebeck coefficient. Following rigorous experimental validation, this ionogel was confirmed to attain a Seebeck coefficient of an impressive 41.39 mV/K, underscoring the model’s efficacy in revealing materials with exceptional thermoelectric properties. This development underscores the potential for machine learning not just to predict, but to transform research methodologies in materials science.
The researchers carried out an important facet of their inquiry into the molecular mechanisms that contribute to the efficient thermoelectric performance of these materials. Through interpretable analyses of the ML model, they were able to pinpoint critical molecular descriptors that play a defining role in influencing the Seebeck coefficient. Two notable features emerged as significant: the number of rotatable bonds in the material and the octanol-water partition coefficient of ion donors. Molecular dynamics simulations corroborated these findings, highlighting how these properties dictate ion diffusion rates and interactions within the polymer matrix. It became evident that while a higher number of rotatable bonds could hinder ion diffusion efficiency, lower partition coefficients were associated with stronger Coulomb interactions that bolster thermoelectric outcomes.
Furthermore, this research underlines the synergistic potential of machine learning models in addressing complex materials science challenges. While gradient-boosted decision trees (GBDT) demonstrated their strength in making highly accurate predictions within known parameter ranges, alternative symbolic regression models such as those based on genetic programming showcased their value in identifying novel material combinations beyond the confines of the original dataset. This dual approach amplifies the reach of their findings, opening new avenues for innovative material design.
Practical validation of the research’s predictive power was achieved through the fabrication and testing of the top-ranked materials, with the WPU/KI ionogel emerging as a significant case study. Under various environmental conditions, this ionogel exhibited robust functionality, demonstrating a temperature-induced voltage increase of 250 mV within a thermal gradient of 5.5 K. Insights from molecular dynamics simulations illuminated the intricacies of the chemical interactions that underpin such performance, emphasizing the pivotal role of electrostatic attractions between potassium ions and the polymer matrix.
The implications of this innovative machine learning framework extend far beyond this singular study. By effectively bridging the gap between theoretical models and experimental implementations, it promises to revolutionize the landscape of ionic thermoelectrics. The high precision and scalability of the model will foster an era of material development characterized by accelerated discovery processes and heightened innovation, propelling forward the frontiers of sustainable energy solutions.
As the researchers continue to explore the uncharted territories of materials science using this machine learning framework, the groundwork is being laid for transformative advancements that could significantly impact energy technology. The potential applications of the resulting materials are vast, encompassing areas such as next-generation thermal sensors and miniaturized waste-heat recovery devices, which are crucial in the quest for sustainable energy systems.
The continued progress in this field symbolizes a shift towards more integrated and less resource-intensive methodologies in materials discovery, ultimately contributing to a more sustainable and energy-efficient future. As the intersection of machine learning and materials science continues to evolve, the research community can anticipate the unveiling of even more groundbreaking discoveries, pushing the boundaries of what is possible in the field of ionic thermoelectrics.
This transformative study thus marks an important milestone in the quest to harness the full potential of ionic thermoelectric materials, heralding a future where innovation is driven not just by experimentation, but also by advanced computational techniques that can predict, optimize, and drive material discovery forward with unprecedented speed and accuracy. The journey has only just begun, but the paths illuminated by this pioneering work are bound to lead to significant advancements in energy technology.
—
Subject of Research: Ionic Thermoelectric Materials
Article Title: Breakthrough Machine Learning Approach Revolutionizes Ionic Thermoelectric Material Discovery
News Publication Date: [Insert Date]
Web References: [Insert Relevant Links]
References: [Insert Relevant Citations]
Image Credits: Science China Press
Keywords
Model, Materials Science, Ionic Thermoelectrics, Machine Learning, Seebeck Coefficient, Energy Conversion, Sustainable Technology
Discover more from Science
Subscribe to get the latest posts sent to your email.
What's Your Reaction?
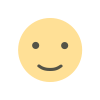
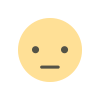
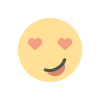
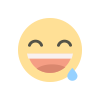
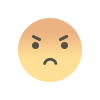
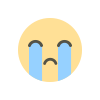
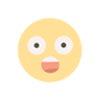