Surfing the AI Wave: Accelerating Accurate Ocean Simulations
Researchers at Osaka Metropolitan University are pioneering a remarkable advancement in fluid dynamics, leveraging the power of artificial intelligence to significantly enhance fluid simulation processes. This breakthrough focuses on machine learning methodologies that bridge the gap between computational efficiency and simulation accuracy. The team has introduced a novel AI-driven model that dramatically reduces computational time—down […]

Researchers at Osaka Metropolitan University are pioneering a remarkable advancement in fluid dynamics, leveraging the power of artificial intelligence to significantly enhance fluid simulation processes. This breakthrough focuses on machine learning methodologies that bridge the gap between computational efficiency and simulation accuracy. The team has introduced a novel AI-driven model that dramatically reduces computational time—down to just one-fifteenth of what traditional particle methods require—without sacrificing the precision that is so critical in various applications, including maritime engineering and oceanography.
Fluid behavior prediction plays a vital role in many industries, particularly those engaged in harnessing wave and tidal energy, as well as in the design of ocean-going vessels and offshore structures. Conventional particle methods have long been the standard for simulating these complex fluid dynamics, yet they demand extensive resources in both time and processing power. This has presented a significant barrier for industries seeking to innovate quickly and efficiently. The Osaka researchers sought to overcome these challenges by developing an AI-driven surrogate model, which they believe possesses the capacity to revolutionize fluid simulation practices.
Using cutting-edge graph neural networks, the Osaka Metropolitan University team has crafted a deep learning-based surrogate model that excels at fast and accurate fluid simulations. This model was designed to be adaptable, demonstrating strong generalization capabilities across a range of fluid dynamic situations. The researchers meticulously explored various training conditions, uncovering essential factors that contribute to the precision of their simulations. By fine-tuning their model, they ensured it could effectively accommodate different time step sizes—a key element in fluid dynamics simulations—alongside diverse fluid movements.
What makes this development particularly groundbreaking is its efficiency; traditional particle-based simulations could take around 45 minutes to compute, while the AI surrogate model completes the same tasks in approximately three minutes. This staggering reduction in computational time enables industries to iterate rapidly through design processes, drastically accelerating research and development timelines. Such efficiency not only has immediate implications for the shipbuilding and renewable energy sectors but also sets the stage for real-time analysis of fluid behavior in various scenarios, offering unprecedented insights into system dynamics.
While the prospects raised by such advancements are captivating, the research team acknowledges the limitations of artificial intelligence. Although AI can yield exceptional results for specific tasks, it can falter when applied to unfamiliar conditions. Lead author Takefumi Higaki, an assistant professor at the Graduate School of Engineering at Osaka Metropolitan University, highlighted the importance of consistency in producing reliable outcomes across a spectrum of scenarios. The development of this model aimed to address those concerns, setting the groundwork for more robust AI applications in fluid dynamics.
As industries increasingly turn their attention toward sustainable practices, the development of this faster and more precise fluid simulation tool is timely. With the rise of offshore power generation and an urgent need to optimize the design of maritime vessels and structures, the demand for enhanced simulation capabilities has never been greater. This research represents a critical step forward in making such advancements accessible and practical, ensuring that areas like ocean energy harnessing can progress with newfound speed and accuracy.
Moreover, the implications of these enhancements extend beyond purely academic interests. Real-time fluid analysis, powered by this AI technology, could empower engineers and designers to make informed decisions swiftly. It could also facilitate adaptive control systems in marine environments, maximizing the efficiency of energy systems that rely on fluctuating ocean conditions. The versatility of the AI model thus presents a dual opportunity: not only does it enhance traditional design processes, but it also affords real-time responses to environmental changes.
The findings of this promising research have been documented in the journal Applied Ocean Research, contributing to the growing body of knowledge that integrates machine learning with environmental engineering practices. As the paper outlines the methodology, results, and implications of the study, it serves as a reference point for other researchers and industry professionals excited about the potential of AI in computational sciences.
In an era where rapid technological innovation is paramount, this research provides a glimpse into a future where computational fluid dynamics is not bound by traditional limitations. Instead, it opens doors to new methodologies where machine learning frameworks could significantly shorten development times across numerous sectors, including energy, transportation, and environmental science.
As Osaka Metropolitan University advances its strong commitment to research and societal impact, this breakthrough in fluid simulation stands as a testament to the institution’s dedication to pioneering new frontiers of knowledge. The university is poised to continue leading in high-performance research and application, fostering developments that ultimately benefit both industry and society at large.
In conclusion, the AI-powered surrogate model put forth by researchers at Osaka Metropolitan University encapsulates the revolutionary possibilities that arise when artificial intelligence meets traditional computational methods. By optimizing fluid simulations, this breakthrough lays the groundwork for more sustainable and efficient practices within industries dependent on fluid behaviors. As this and similar research progresses, society may find itself at the cusp of a new era in which technology continuously evolves, offering substantial enhancements to our understanding and management of natural resources.
Subject of Research: Fluid Simulation using AI-Based Surrogate Models
Article Title: Breakthrough AI Model Revolutionizes Fluid Dynamics Simulation
News Publication Date: [Date not specified]
Web References: [Links not provided]
References: [Not specified in content]
Image Credits: Credit: Osaka Metropolitan University
Keywords
Artificial Intelligence, Fluid Dynamics, Machine Learning, Computational Modeling, Ocean Engineering, Surrogate Models, Graph Neural Networks, Real-time Analysis, Marine Energy, Offshore Design, Efficiency, Fluid Simulation.
Tags: accurate fluid simulation methodsadvancements in maritime engineeringAI-driven fluid dynamicscomputational efficiency in fluid dynamicsdeep learning for fluid behavior predictiongraph neural networks in simulationsmachine learning in ocean simulationsoffshore structures design innovationsOsaka Metropolitan University researchovercoming computational challenges in oceanographyrevolutionary AI simulation modelswave and tidal energy simulation
What's Your Reaction?
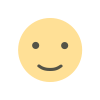
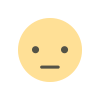
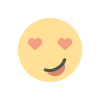
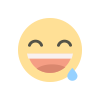
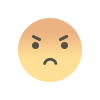
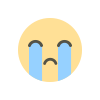
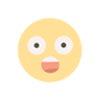