Scientists Create Innovative Metallic Materials Through Data-Driven Frameworks and Explainable AI
In a groundbreaking development poised to revolutionize materials science, researchers led by Sanket Deshmukh, an associate professor of chemical engineering at Virginia Tech, have harnessed the power of explainable artificial intelligence (AI) to design new multiple principal element alloys (MPEAs) with enhanced mechanical properties. These innovative alloys boast exceptional strength and resilience, opening fresh avenues […]

In a groundbreaking development poised to revolutionize materials science, researchers led by Sanket Deshmukh, an associate professor of chemical engineering at Virginia Tech, have harnessed the power of explainable artificial intelligence (AI) to design new multiple principal element alloys (MPEAs) with enhanced mechanical properties. These innovative alloys boast exceptional strength and resilience, opening fresh avenues for applications spanning from medical implants to aerospace components. The findings, funded by the National Science Foundation and published in npj Computational Materials, signal a paradigm shift in how advanced metallic materials are discovered and optimized.
MPEAs, also known as high-entropy alloys, differentiate themselves by being composed of three or more principal metallic elements, a composition strategy that delivers formidable strength, corrosion resistance, and thermal stability. These attributes make MPEAs vital in critical technologies such as knee replacements, bone plates, catalytic converters, and high-performance aircraft parts. Despite their immense potential, the traditional approach to crafting such alloys has been hindered by extensive trial-and-error experimentation, often resulting in protracted and costly development cycles.
Deshmukh and his multidisciplinary team sought to overcome these limitations by integrating a data-driven methodology with state-of-the-art explainable AI techniques. Explainable AI diverges from conventional “black box” models by illuminating the rationale behind its predictions, providing tangible insights into the interplay of elemental compositions and their influence on alloy characteristics. Employing SHAP (SHapley Additive exPlanations) analysis, the researchers unpacked the AI’s decision-making process, revealing elemental synergies and local atomic environments critical to achieving superior mechanical performance.
This innovative AI framework enabled rapid screening and optimization of countless hypothetical MPEA formulations, narrowing down options that would otherwise take years of experimental work to evaluate. By leveraging expansive datasets incorporating both experimental results and high-fidelity simulations, the model could predict with remarkable accuracy how various combinations and concentrations of elements affect key properties such as yield strength, ductility, and fatigue resistance. This predictive capability transforms materials discovery from a largely empirical endeavor into an informed, highly focused exploration.
The team went beyond pure computational exploration by integrating evolutionary algorithms into the design pipeline. These algorithms mimic natural selection mechanisms, iteratively refining alloy compositions toward optimal performance. Through this synergistic pairing of explainable AI and evolutionary computation, the researchers accelerated the identification of promising MPEAs that not only outperform traditional alloys but also offer greater resistance to wear and corrosion under extreme conditions.
A pivotal aspect of this research is the tangible interpretability that explainable AI provides, enabling scientists to extract fundamental materials science insights rather than merely relying on opaque predictions. Understanding which elements and atomic-scale factors drive mechanical behavior allows for more rational, hypothesis-driven alloy engineering. Such insights pave the way for expanding this methodology to design other complex materials beyond metals, including polymeric glycomaterials, which hold promise for applications in personal care products, food additives, and biomedical devices.
Fangxi “Toby” Wang, a postdoctoral associate on the project, emphasized that this approach is not just about faster discovery but creating versatile, transferable design tools. The workflow’s interpretability and adaptability provide a blueprint for the broader materials community to tackle various complex systems where traditional trial-and-error design approaches fall short. It embodies a future where materials scientists can systematically tailor material properties with unprecedented precision and understanding.
Collaboration across disciplines and institutions was instrumental in reaching these milestones. Partnerships with experts like Tyrel McQueen, a professor of materials science at Johns Hopkins University, and Maren Roman, a sustainable biomaterials professor at Virginia Tech and director of the NSF-supported GlycoMIP platform, enriched the research’s depth and scope. This interdisciplinary synergy bridged computational modeling, experimental synthesis, and characterization, enabling a closed-loop process that validated novel MPEA predictions and accelerated the transition from design to application.
Graduate researchers, including Allana Iwanicki at Johns Hopkins, contributed by synthesizing and testing the new alloy compositions in laboratory settings. Their experimental work confirmed the enhanced mechanical attributes predicted by the AI model, validating the novel computational methodologies. This comprehensive approach from theoretical design to empirical validation underscores the robustness and real-world relevance of the new materials discovery framework.
While initial efforts concentrated on solvent-free metallic systems, Deshmukh’s team has expanded their AI-driven computational design to more intricate biomaterials like glycomaterials—polymers containing carbohydrate structures. These materials possess broad applicability in biotechnology and consumer sectors, highlighting the translational potential of combining explainable AI with materials innovation platforms. The ability to rationally engineer such complex systems could catalyze breakthroughs in health, sustainability, and manufacturing technologies.
Deshmukh remarked that this research exemplifies the transformative power of integrating AI-driven predictive modeling with experimental science. It not only accelerates the discovery of superior metallic alloys for industrial applications but also establishes a versatile discoverability framework that can cross traditional disciplinary boundaries. The union of machine learning, evolutionary computation, and interpretability marks a significant stride towards a new era of scientific materials design, where predictive understanding replaces empirical guessing.
As AI continues to evolve, its impact on materials science is expected to deepen, shifting paradigms of how alloys and advanced materials are conceptualized, optimized, and commercialized. This research highlights the profound value of transparency in AI-driven decisions—ensuring that scientific innovation remains explainable, trustworthy, and actionable. With such advancements, industries will more swiftly develop materials that meet bespoke performance specifications while reducing costs and environmental impact.
The synthesis of explainable AI with materials engineering heralds a new frontier wherein human insight and computational power operate in concert, unraveling the complexities of atomic interactions at unprecedented speed and clarity. This not only accelerates innovation but empowers researchers to innovate with a purpose, creating next-generation materials that advance technology and improve lives on a global scale.
Subject of Research: Development of advanced multiple principal element alloys (MPEAs) using explainable artificial intelligence for superior mechanical properties.
Article Title: Not explicitly provided.
News Publication Date: 15-May-2025
Web References:
Sanket Deshmukh faculty profile: https://che.vt.edu/People/faculty/Deshmukh.html
Chemical engineering department at Virginia Tech: http://che.vt.edu/
GlycoMIP NSF Materials Innovation Platform: https://glycomip.org/
Article DOI: http://dx.doi.org/10.1038/s41524-025-01600-x
References: Published in npj Computational Materials (Nature), DOI: 10.1038/s41524-025-01600-x
Image Credits: Photo by Hailey Wade for Virginia Tech
Keywords
Chemical engineering, Engineering, Materials science, Metals, Alloys, Artificial intelligence, Computer science, Machine learning, Biomaterials
Tags: advanced metallic materials for aerospaceAI-driven optimization in materials engineeringapplications of MPEAs in medical technologycorrosion resistance in advanced alloysdata-driven materials scienceexplainable artificial intelligence in materialsinnovative alloy design techniquesmechanical properties of high-entropy alloysmultiple principal element alloysovercoming traditional alloy development challengesparadigm shift in material discovery methodsstrength and resilience in metallic materials
What's Your Reaction?
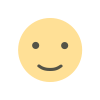
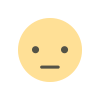
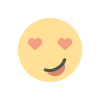
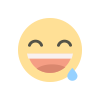
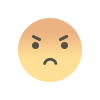
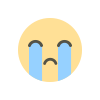
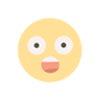