Scalable Spatial Transcriptomics via Computational Array Reconstruction
In recent years, spatial transcriptomics has revolutionized our understanding of gene expression by enabling scientists to observe where genes are activated within the complex architecture of tissues. Traditionally, this technology relies heavily on high-resolution imaging techniques to locate spatial barcodes that correspond to specific gene expression patterns across a tissue section. However, these imaging modalities […]

In recent years, spatial transcriptomics has revolutionized our understanding of gene expression by enabling scientists to observe where genes are activated within the complex architecture of tissues. Traditionally, this technology relies heavily on high-resolution imaging techniques to locate spatial barcodes that correspond to specific gene expression patterns across a tissue section. However, these imaging modalities come with significant limitations, including expensive equipment, time-consuming data acquisition, and challenges in scaling up to larger tissue samples or whole organs. That bottleneck has long constrained the throughput and accessibility of spatial transcriptomics, hampering its widespread adoption in both basic research and clinical settings.
Addressing these challenges, a groundbreaking study led by Hu, Borji, Marrero, and their collaborators has introduced an entirely new paradigm for spatial transcriptomics that bypasses the need for imaging. Published in Nature Biotechnology, their work describes a computational framework that reconstructs the spatial locations of transcriptomic barcodes through an innovative approach leveraging molecular diffusion patterns paired with dimensionality reduction algorithms. This development is a stunning leap forward, as it enables scalable, imaging-free spatial transcriptomics capable of mapping centimeter-scale tissue sections with remarkable fidelity, opening doors to expansive biological explorations previously hamstrung by the physical constraints of microscopy.
The core insight underpinning this method is the utilization of molecular diffusion—a naturally occurring physical process—as an informational conduit to infer spatial barcode positions. When spatial barcodes infused in a tissue diffuse outward, their overlaps and gradients form unique molecular signatures that encode spatial proximity information. By mathematically modeling the diffusion process and capturing the gradient patterns it produces, the researchers designed algorithms capable of reconstructing two-dimensional spatial maps without directly capturing images. This fusion of biology and computational science transcends prior limitations by exploiting the inherent physics of molecular behavior rather than depending on hardware-based imaging.
A pivotal component of their framework involves the application of dimensionality reduction techniques, such as manifold learning and graph-based embeddings, which parse through the complex diffusion-generated data to reveal spatial relationships between barcodes. These powerful mathematical tools reduce high-dimensional diffusion signals into manageable spatial maps that accurately recapitulate tissue architecture. This computational sophistication enables the extraction of meaningful spatial coordinates from what would otherwise appear as tangled, noisy molecular patterns, and it is this capability that differentiates the approach from previous methodologies dependent on direct fluorescent or optical readouts.
The robustness of their imaging-free system was meticulously validated through comparison with traditional ground-truth imaging datasets. By applying their computational reconstruction to tissues with known spatial configurations, the team demonstrated a high degree of concordance between their inferred maps and those obtained through microscopy. This fidelity substantiates the method’s accuracy, making it viable for routine biological investigations where imaging might be infeasible due to resource limitations or tissue size constraints. Importantly, this validation underlines the potential for this technology to function as a reliable surrogate for imaging, rather than merely a theoretical alternative.
One of the most transformative implications of this technology is its scalability. The integration of diffusion-based spatial encoding with computation allows for gene expression mapping across previously unattainable spatial scales. Traditional imaging techniques often struggle with centimeter-scale tissue due to limits in optical field of view, imaging time, and resolution trade-offs. The novel computational strategy detaches the spatial resolution from optical constraints, enabling large tissues or even whole organs to be profiled spatially in a fraction of the time and without specialized instruments. This leap expands the horizon for spatial transcriptomics from small biopsies to expansive tissue landscapes.
Beyond throughput and scale, this approach markedly democratizes access to spatial transcriptomics. High-end microscopy setups are costly, require expert operation, and represent a bottleneck that restricts smaller laboratories and clinical centers from utilizing spatial transcriptomic analyses fully. The imaging-free computational reconstruction method removes these barriers, enabling a broader swath of the scientific and medical community to interrogate tissue architecture. The implications for disease research, biomarker discovery, and personalized medicine are profound, as vast and diverse tissue samples can now be spatially profiled with minimal equipment.
Further technical details reveal the thoughtful experimental design that complements the computational reconstruction. The method begins by labeling tissue sections with molecular barcodes that diffuse through the extracellular space. The diffusion pattern is intentionally optimized through controlled application of reagents and environmental parameters to ensure that spatial signal gradients are sufficiently distinguishable. Once sequencing data is acquired from these barcoded tissues, the computational pipeline reconstructs spatial arrangements by solving inverse problems related to diffusion pattern deconvolution, leveraging statistical regularization methods to handle noise and ambiguity.
The algorithms developed incorporate advanced machine learning techniques capable of iterative refinement, improving spatial resolution as data quality increases. Importantly, these computational tools are adaptable, with parameters tunable to different tissue types, barcode densities, and diffusion characteristics. This flexibility means the methodology is not a one-size-fits-all but rather a versatile platform designed to accommodate the wide heterogeneity of biological tissues and experimental setups intrinsic to spatial biology research.
The study also discusses the potential for integrating this computational approach with multi-omic spatial data modalities. As spatial transcriptomics converges with proteomics and epigenomics, the ability to spatially localize multiple molecular layers without imaging simultaneously could accelerate systems-level understanding of tissue function. Since imaging constraints often limit simultaneous multi-modal capture, this imaging-free reconstruction provides a promising route to overcome such bottlenecks, enabling richer, multi-dimensional tissue profiling.
Moreover, this technology’s ability to generate comprehensive spatial maps without the need for optical access has exciting implications for in situ applications where imaging is impossible due to tissue opacity or damage. For example, fibrotic or calcified tissues, or clinical specimens embedded in dense matrices, often pose insurmountable challenges for microscopy. Here, the diffusion-based computational reconstruction offers a powerful alternative to glean spatial transcriptomic insights, potentially transforming how such difficult samples are analyzed.
Despite its many advantages, the researchers acknowledge future challenges requiring further refinement. For instance, achieving ultra-high spatial resolution comparable to single-cell imaging may necessitate enhanced barcode design and more sophisticated modeling of diffusion and molecular interactions. Additionally, integrating real-time or dynamic spatial transcriptomic profiling remains an aspirational goal, as current diffusion-based strategies capture static snapshots. Nonetheless, the groundwork laid by this study paves the way for continuous technological evolution.
In the broader context of spatial biology, the imaging-free computational reconstruction emerges as a disruptive innovation that could redefine the boundaries of where and how spatial transcriptomics is applied. By decoupling spatial mapping from microscopy, it frees research from traditional constraints, enabling unprecedented throughput, accessibility, and scalability. Whether applied in developmental biology, neuroscience, oncology, or regenerative medicine, this technique promises to catalyze discoveries that hinge on understanding spatial cellular heterogeneity.
Finally, the open accessibility of the computational tools aside from standard sequencing workflows highlights the research team’s commitment to fostering community-wide adoption. They have made their software pipeline available to the scientific public, encouraging widespread utilization and iterative improvement. This openness is crucial for the rapid dissemination of cutting-edge methodologies and will likely accelerate innovations spurred by this imaging-free spatial transcriptomics platform.
As spatial transcriptomics continues its rapid ascent as a cornerstone technique for biological insight, innovations like the one presented by Hu, Borji, Marrero, and colleagues underscore the power of interdisciplinary synergy. By harnessing the physics of molecular diffusion and the precision of computational analytics, they have charted a novel pathway that sidesteps the complexities of imaging, democratizes spatial biology, and accelerates large-scale, high-resolution molecular mapping. The future of spatial transcriptomics is not only brighter but more accessible than ever before.
Article References:
Hu, C., Borji, M., Marrero, G.J. et al. Scalable spatial transcriptomics through computational array reconstruction. Nat Biotechnol (2025). https://doi.org/10.1038/s41587-025-02612-0
Tags: applications in clinical researchbreakthroughs in tissue architecture studieschallenges in spatial barcodingcomputational array reconstruction techniquesdimensionality reduction in biologygene expression mapping innovationshigh-resolution imaging limitationsimaging-free transcriptomics methodsmolecular diffusion in transcriptomicsNature Biotechnology publication highlightsscalable tissue analysis technologiesspatial transcriptomics advancements
What's Your Reaction?
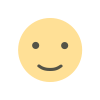
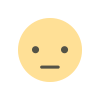
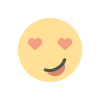
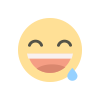
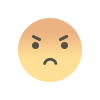
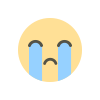
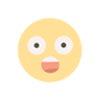