Revolutionizing Melt Pool Prediction: Introducing the Transfer Learning-Enhanced Physics-Informed Neural Network (TLE-PINN)
Researchers are on the cutting edge of improving manufacturing efficiency with the development of a groundbreaking method known as the Transfer Learning-Enhanced Physics-Informed Neural Network (TLE-PINN). This novel technique promises to revolutionize prediction models in selective laser melting (SLM), a sophisticated technique that underpins the additive manufacturing of complex metal components. The incredibly intricate process […]

Researchers are on the cutting edge of improving manufacturing efficiency with the development of a groundbreaking method known as the Transfer Learning-Enhanced Physics-Informed Neural Network (TLE-PINN). This novel technique promises to revolutionize prediction models in selective laser melting (SLM), a sophisticated technique that underpins the additive manufacturing of complex metal components. The incredibly intricate process of accurately estimating the melt pool morphology—crucial for determining material properties and quality—has traditionally struggled with significant challenges due to its complex, multi-physics nature. However, researchers from Wuhan University have made a profound impact by combining physics-informed neural networks with deep learning processes.
The need for a paradigm shift in SLM modeling arises from the limitations seen in conventional approaches. Traditional numerical simulations tend to be computationally expensive and inherently time-consuming, making them less suitable for real-time applications. Furthermore, purely data-driven models often lack the necessary physical consistency, leading to predictions that may not accurately represent the behavior of the melt pool under diverse manufacturing conditions. The demand for high accuracy in this context directly correlates with the quality of end products across industries like aerospace, automotive engineering, and healthcare, where material precision is non-negotiable.
Integrating deep learning into the predictive modeling of melt pool morphologies offers a unique solution to these complicated issues. The TLE-PINN method cleverly employs a transfer learning framework that draws from a wealth of existing data and simulations. This framework allows for a more efficient modeling approach by fine-tuning only the final layers of the model, keeping earlier network parameters intact. By doing so, the researchers circumvent the extensive training times typically associated with machine learning models while simultaneously ensuring that predictions retain high accuracy.
One of the fundamental advantages of TLE-PINN lies in its robust ability to incorporate physical constraints into its algorithms. The EPINN component integrates crucial heat transfer equations and boundary conditions directly into the neural network’s loss function. This incorporation ensures that the predictions generated by the model stay true to physical realities, even under complex manufacturing conditions. The result is a model that not only predicts melt pool morphology but actively respects the thermal dynamics governing that process, making predictions more reliable.
Professor Yaowu Hu of Wuhan University has emphasized the significance of this innovative methodology in additive manufacturing. He asserts that TLE-PINN serves as a bridge between traditional numerical simulations and artificial intelligence tools, effectively merging two often disparate schools of thought into a coherent, effective predictive model. This holds great promise for various industries where the production of high-quality materials is paramount. Coupled with the ability to enable intelligent, real-time process controls, TLE-PINN is set to improve manufacturing quality dramatically.
In a validation study, the TLE-PINN framework was tested against a variety of laser scanning speeds using 42CrMo steel samples. Comparisons of the model’s predictions with high-fidelity simulation data and experimental outcomes revealed remarkable accuracy. Not only did TLE-PINN demonstrate improved performance in predicting melt pool morphologies, but it also exhibited significantly lower temperature deviations when juxtaposed with traditional Physics-Informed Neural Networks (PINN) and other data-driven methods. These findings underscore TLE-PINN’s robustness and reliability in challenging scenarios.
Furthermore, its computational efficiency stands out as a game-changer. Traditional predictive models often demand extensive computational resources and countless hours of training, rendering them impractical for immediate, real-time applications. In contrast, TLE-PINN is able to achieve faster convergence rates while utilizing fewer computational resources, marking it as a cost-effective option for large-scale manufacturing settings. This efficiency, coupled with the model’s adaptability to various SLM parameters and material types, points toward a future where complex manufacturing processes can be controlled and optimized more effectively.
As researchers look beyond the current capabilities of TLE-PINN, the prospects for its application in the SLM field appear vast and promising. Intended expansions might include addressing challenges posed by even more complex material systems and larger parameter ranges, which could improve the model’s applicability in industrial scenarios. Notably, smoother integration of TLE-PINN into real-time process control applications could facilitate immediate adjustments in manufacturing parameters without sacrificing quality or efficiency.
With these accomplishments, the TLE-PINN method signifies a substantial leap forward in hybridizing artificial intelligence with physics-based modeling. The potential applications of this transformative approach extend beyond SLM, potentially influencing a variety of sectors where precision in manufacturing is of utmost importance. This research represents more than just a technological advance; it suggests a paradigm shift likely to influence future trends in smart manufacturing.
While substantial work remains to perfectly capture the myriad complexities of melt pool behavior across differing conditions, this innovative study is an essential stride toward smarter, more efficient manufacturing methodologies. As industries push towards incorporating more intelligent and automated solutions, TLE-PINN could very well be the key to unlocking greater operational efficiencies, enhanced product quality, and increased adaptability in production processes.
The implications of this study extend not only to academic and industrial circles but also present broader opportunities for integrating rigorous scientific principles with cutting-edge technological advancements. TLE-PINN is more than just a model—it embodies the future of additive manufacturing, illustrating how the right blend of science, engineering, and machine learning can solve real-world challenges in innovative ways.
By advancing the capacity for accurate melt pool predictions in laser melting, this research paves the way for a new era in manufacturing where operations are not only faster but more precise, responsive, and capable of adapting to the evolving landscape of industry needs. The insights gained through TLE-PINN hold the promise of informing future advancements across a spectrum of applications within the realm of additive manufacturing technology.
Subject of Research:
Article Title: Transfer learning-enhanced physics informed neural network for accurate melt pool prediction in laser melting
News Publication Date: 3-Jan-2025
Web References: DOI
References: Zhu Q, Lu Z, Hu Y. Transfer learning-enhanced physics informed neural network for accurate melt pool prediction in laser melting. Adv. Manuf. 2025(1):0001
Image Credits: Qingyun Zhu/ Wuhan University, Zhengxin Lu/ Wuhan University, Yaowu Hu/ Wuhan University
Keywords
Deep learning, Transfer learning, Selective laser melting, Physics-informed neural networks, Manufacturing efficiency.
What's Your Reaction?
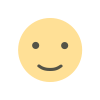
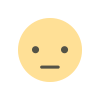
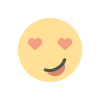
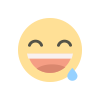
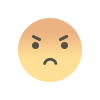
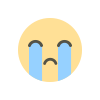
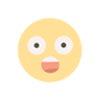