Revolutionary AI Platform Pinpoints Patients Most Likely to Gain from Clinical Trials
A groundbreaking study spearheaded by the Winship Cancer Institute of Emory University in conjunction with the Abramson Cancer Center of the University of Pennsylvania has unveiled an innovative artificial intelligence (AI) platform that stands poised to revolutionize the way clinicians and patients evaluate the potential benefits of therapies under investigation in clinical trials. This cutting-edge […]

A groundbreaking study spearheaded by the Winship Cancer Institute of Emory University in conjunction with the Abramson Cancer Center of the University of Pennsylvania has unveiled an innovative artificial intelligence (AI) platform that stands poised to revolutionize the way clinicians and patients evaluate the potential benefits of therapies under investigation in clinical trials. This cutting-edge AI tool, dubbed TrialTranslator, provides a sophisticated means for making nuanced treatment decisions and anticipating the outcomes associated with novel interventions, thereby enhancing the overall quality of patient care and strategic planning for future medical interventions.
Published in the prestigious journal Nature Medicine, the research is fronted by the distinguished medical oncologist Dr. Ravi B. Parikh, who serves as the medical director of the Data and Technology Applications Shared Resource at Winship Cancer Institute, along with Dr. Qi Long from the University of Pennsylvania, who is a leading figure in biostatistical research. Their collaborative effort represents a significant evolution in the interface between AI and oncology, emphasizing the crucial role of machine learning in bridging the existing gaps between clinical trial outcomes and real-world patient experiences.
The underlying premise of TrialTranslator hinges on its ability to leverage vast datasets drawn from electronic health records (EHR) to emulate results from landmark clinical trials. This is a pivotal development, especially considering that traditional clinical trials often encompass only a fraction of the patient population afflicted by various cancers. By utilizing real-world data to emulate the methodologies of eleven prominent randomized controlled trials focused on the most prevalent advanced solid malignancies in the United States, the research team was able to draw parallels that could directly influence patient treatment plans.
Through this sophisticated AI framework, the researchers effectively demonstrated that distinct patient phenotypes—identified through machine learning algorithms—could yield varied responses to the same treatment protocols. It was identified that individuals categorized with low- and medium-risk prognoses showcased survival metrics closely aligned with those documented in the randomized trials. Conversely, patients classified as high-risk exhibited significantly poorer survival outcomes, emphasizing the nuanced differences in responses that AI can help illuminate.
Dr. Parikh articulates the potential utility of this platform, expressing hopes that it will empower both clinicians and patients to make more informed decisions about whether clinical trial results are applicable to their specific clinical situations. The ramifications of this research extend beyond patient decision-making; it also lays down the groundwork for future clinical trials targeting those populations for whom standard therapies may prove ineffective.
The importance of this research cannot be overstated, as it sheds light on the restrictive eligibility criteria that often characterize clinical trials. These criteria can alienate substantial cohorts of patients, leading to outcomes that fail to encapsulate the true diversity of responses found in the general cancer patient population. By shifting the focus to overarching prognostic factors rather than strictly adhering to eligibility benchmarks, future research can continue to evolve to more accurately reflect real-world scenarios.
Dr. Long encapsulates the broader implications of the study, showcasing its potential to harness the intricate nature of real-world data through AI and machine learning methodologies to significantly advance precision medicine. His optimism conveys that future implementations of AI in oncological research could yield profound insights into patient responses, diagnostic accuracy, and even early cancer detection.
Utilizing Flatiron Health’s extensive nationwide database of electronic health records, the researchers scrutinized the dynamics of treatment regimens across various malignancies, including advanced non-small cell lung cancer, metastatic breast cancer, metastatic prostate cancer, and metastatic colorectal cancer. This comprehensive approach allowed for a robust comparative analysis of treatment effects as gleaned from randomized controlled trials.
The focus on patient heterogeneity evident in the findings suggests a stark contrast to traditional therapeutic applications derived from clinical trials, which typically operate under an oversimplified notion of patient responses. The recognition that real-world patient populations exhibit a greater spectrum of prognostic traits can lead to more tailored treatment approaches that significantly improve care quality.
With recommendations from notable organizations such as the American Society of Clinical Oncology advocating for improved representation of high-risk populations in clinical trials, the study aligns perfectly with contemporary discussions in the field regarding inclusivity and the need for a paradigm shift in clinical research methodologies.
Looking ahead, Dr. Parikh envisions a future where AI-based biomarkers become commonplace in oncological settings, capable of dissecting complex datasets from pathology reports, radiological imaging, and electronic health records. Such advancements would enable precision medicine to not only tailor treatment selections but also catch cancers at earlier stages, ultimately leading to enhanced patient outcomes and survival rates.
In summary, the intersection of AI and oncology as illustrated in this study encapsulates an exciting frontier in medical research, raising the bar for how we define and approach cancer treatment strategies. The implications of this research extend far beyond the laboratory, possessing the potential to meaningfully alter clinical practices and improve lives through more personalized healthcare solutions.
Subject of Research: People
Article Title: Evaluating generalizability of oncology trial results to real-world patients using machine learning-based trial emulations
News Publication Date: 3-Jan-2025
Web References: Link to the published study
References: DOI: 10.1038/s41591-024-03352-5
Image Credits: Nature Medicine
Keywords: Clinical trials, artificial intelligence, oncology, machine learning, precision medicine, treatment decision-making, survival outcomes, patient heterogeneity, electronic health records.
What's Your Reaction?
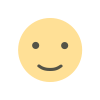
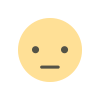
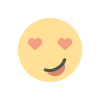
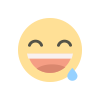
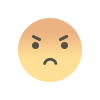
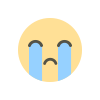
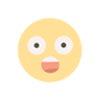