Predicting Clinical Outcomes with Machine Learning and Real Data
In a landmark study poised to redefine the precision medicine landscape, researchers have successfully harnessed real-world data alongside advanced machine learning techniques to identify predictive subphenotypes that forecast clinical outcomes with unprecedented accuracy. This breakthrough, detailed in a recent publication in Nature Communications, promises to revolutionize how clinicians stratify patients, tailor treatments, and ultimately improve […]

In a landmark study poised to redefine the precision medicine landscape, researchers have successfully harnessed real-world data alongside advanced machine learning techniques to identify predictive subphenotypes that forecast clinical outcomes with unprecedented accuracy. This breakthrough, detailed in a recent publication in Nature Communications, promises to revolutionize how clinicians stratify patients, tailor treatments, and ultimately improve prognoses across a spectrum of diseases. The collaborative effort led by Pan, W., Hathi, D., Xu, Z., and colleagues represents a compelling demonstration of the power of integrating vast, real-world clinical datasets with cutting-edge artificial intelligence methodologies.
At the core of this study lies the challenge of patient heterogeneity, a persistent obstacle in clinical management where variations in disease presentation and progression complicate treatment decisions. Traditional approaches often treat patients as monolithic groups, thereby obscuring subtle but clinically significant differences that influence outcomes. The team circumvented this limitation by employing unsupervised machine learning algorithms capable of dissecting the multifaceted patterns embedded in large-scale health records. These algorithms uncovered distinct subphenotypes—essentially patient subgroups characterized by specific combinations of clinical features—that bear predictive relevance to disease trajectories and therapy responses.
The datasets underpinning this research were drawn from a rich tapestry of real-world sources, including electronic health records (EHRs), claims data, laboratory results, and longitudinal follow-ups that reflect the uncontrolled complexity of routine clinical practice. Such data captures the often-overlooked nuances of patient variability, co-morbidities, and treatment adherence, factors traditionally underrepresented in clinical trials. By leveraging this wealth of information, the authors could ensure that the derived subphenotypes possess strong external validity and pragmatic utility in everyday healthcare environments.
A pivotal methodological pillar of the project involved feature engineering strategies adept at transforming multifarious clinical variables into a high-dimensional representation adequate for machine learning analysis. The team meticulously curated and normalized clinical metrics ranging from biochemical markers to imaging findings and demographic data, layering these into a harmonized framework. Dimensionality reduction techniques, including principal component analysis and t-distributed stochastic neighbor embedding, were deployed to visualize and interpret complex phenotypic clusters before confirming their prognostic significance through rigorous statistical validation.
What sets this research apart from previous endeavors is its focus on predictive functionality rather than descriptive clustering. The machine learning models were trained not merely to categorize patient data but to forecast meaningful clinical endpoints—such as mortality risk, disease exacerbation, and treatment responsiveness. This predictive lens ensures that identified subphenotypes translate directly into actionable insights, equipping clinicians with tools to anticipate patient trajectories and modify therapeutic strategies proactively.
Importantly, the study also highlights the interpretability of the machine learning models employed, addressing a frequently cited criticism of AI applications in medicine—namely, the “black box” problem. Through the application of explainability techniques like SHAP (SHapley Additive exPlanations) values and feature importance rankings, the researchers elucidated the specific clinical attributes driving subphenotype differentiation. This transparency fosters clinician trust and facilitates collaborative decision-making between human expertise and algorithmic recommendations.
The impact of this research extends beyond individual patient care. By identifying reproducible and clinically meaningful subphenotypes, this work lays the foundation for more nuanced patient stratification in clinical trials, potentially enhancing the discovery of targeted therapies and increasing trial efficiency. Moreover, the approach paves the way for population health management strategies that can allocate medical resources more judiciously by focusing interventions on groups with highest predicted risk or vulnerability.
Another striking dimension of the study is its demonstration of cross-disease applicability. While many phenotyping efforts focus narrowly on single conditions, the framework advanced by Pan et al. is adaptable to multiple disease domains, including chronic illnesses such as heart failure, chronic obstructive pulmonary disease, and autoimmune disorders. This versatility is facilitated by the modularity of the analytic pipeline and the robustness of machine learning models in capturing complex clinical interactions.
The authors did not shy away from addressing the challenges intrinsic to real-world data. They confronted issues of missingness, heterogeneity, and noise through sophisticated imputation techniques and robust sensitivity analyses, ensuring that the identified subphenotypes reflect genuine biological and clinical signals rather than artifacts of data quality. These stringent safeguards bolster confidence in the generalizability and reproducibility of their findings.
Ethical considerations also surfaced prominently in the study framework. The utilization of patient data necessitates rigorous protections to ensure privacy and confidentiality, criteria that were met through secure data governance policies and anonymization protocols. The researchers highlight the importance of maintaining these standards to preserve public trust while unlocking the transformative potential of AI-guided medical research.
Looking forward, the integration of these predictive subphenotyping methods into clinical decision support systems holds immense promise. Real-time application of such models could empower healthcare providers with personalized risk assessments at the point of care, facilitating timely interventions that improve patient outcomes. Moreover, the dynamic nature of these algorithms allows continuous learning from new incoming data, fostering adaptive models that evolve in parallel with emerging clinical knowledge.
The broader implications of this research resonate with ongoing efforts to move beyond one-size-fits-all medicine towards truly individualized care. By capturing the intricate interplay of lifestyle, biology, and treatment history encoded in real-world data, machine learning-driven subphenotypes offer a roadmap for transforming heterogeneous patient populations into actionable clusters. This transformation has the potential to reduce healthcare disparities by aligning resources with patient-specific risks and optimizing therapeutic efficacy.
Given the accelerating accumulation of health data worldwide, the scalability of the proposed framework is particularly relevant. As digital health ecosystems expand, the capacity to translate big data into clinically meaningful insights becomes imperative. The study by Pan and colleagues serves as a proof of concept that harnessing real-world evidence with sophisticated AI tools can bridge the gap between data abundance and patient-centric care.
In sum, this pioneering research signals a paradigm shift in clinical phenotyping—from retrospective descriptive models to proactive, predictive stratification in real-world settings. It underscores the vital synergy between clinicians, data scientists, and machine learning engineers in navigating the complexities of medical data to unearth signals that can guide personalized medicine. As these methods become integrated into standard practice, they are poised to enhance diagnostic precision, prognostic accuracy, and therapeutic personalization on an unprecedented scale.
While challenges remain—including the need for prospective validation across diverse populations and seamless integration into diverse healthcare workflows—the trajectory set by this study is undeniably exciting. The confluence of sophisticated AI and comprehensive real-world data heralds a new era of precision health where patient care is informed by nuanced, predictive insights drawn from the collective clinical experience of millions.
The future of medicine may soon be defined not just by the availability of data but by our ability to extract meaningful knowledge using intelligent, transparent algorithms. The work of Pan, Hathi, Xu, and collaborators exemplifies this transformative potential, marking a critical step forward in the quest to harness machine learning for better health outcomes worldwide.
Subject of Research: Identification of predictive subphenotypes for clinical outcomes through integration of real-world clinical data and machine learning methods.
Article Title: Identification of predictive subphenotypes for clinical outcomes using real world data and machine learning.
Article References:
Pan, W., Hathi, D., Xu, Z. et al. Identification of predictive subphenotypes for clinical outcomes using real world data and machine learning. Nat Commun 16, 3797 (2025). https://doi.org/10.1038/s41467-025-59092-8
Image Credits: AI Generated
Tags: clinical decision-making improvementsintegrating health records with AImachine learning in healthcarepatient heterogeneity challengesPrecision Medicine Advancementspredicting clinical outcomesreal-world data in medicinerevolutionizing disease prognosissubphenotype identification techniquestreatment personalization strategiesunsupervised machine learning applications
What's Your Reaction?
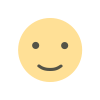
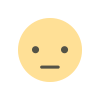
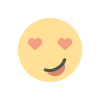
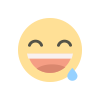
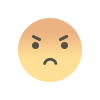
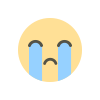
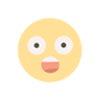