Peering into the mind of artificial intelligence to make better antibiotics
DENVER, Aug. 18, 2024 — Artificial intelligence (AI) has exploded in popularity. It powers models that help us drive vehicles, proofread emails and even design new molecules for medications. But just like a human, it’s hard to read AI’s mind. Explainable AI (XAI), a subset of the technology, could help us do just that by […]

DENVER, Aug. 18, 2024 — Artificial intelligence (AI) has exploded in popularity. It powers models that help us drive vehicles, proofread emails and even design new molecules for medications. But just like a human, it’s hard to read AI’s mind. Explainable AI (XAI), a subset of the technology, could help us do just that by justifying a model’s decisions. And now, researchers are using XAI to not only scrutinize predictive AI models more closely, but also to peer deeper into the field of chemistry.
Credit: American Chemical Society
DENVER, Aug. 18, 2024 — Artificial intelligence (AI) has exploded in popularity. It powers models that help us drive vehicles, proofread emails and even design new molecules for medications. But just like a human, it’s hard to read AI’s mind. Explainable AI (XAI), a subset of the technology, could help us do just that by justifying a model’s decisions. And now, researchers are using XAI to not only scrutinize predictive AI models more closely, but also to peer deeper into the field of chemistry.
The researchers will present their results at the fall meeting of the American Chemical Society (ACS). ACS Fall 2024 is a hybrid meeting being held virtually and in person Aug. 18-22; it features about 10,000 presentations on a range of science topics.
AI’s vast number of uses has made it almost ubiquitous in today’s technological landscape. However, many AI models are black boxes, meaning it’s not clear exactly what steps are taken to produce a result. And when that result is something like a potential drug molecule, not understanding the steps might stir up skepticism with scientists and the public alike. “As scientists, we like justification,” explains Rebecca Davis, a chemistry professor at the University of Manitoba. “If we can come up with models that help provide some insight into how AI makes its decisions, it could potentially make scientists more comfortable with these methodologies.”
One way to provide that justification is with XAI. These machine learning algorithms can help us see behind the scenes of AI decision making. Though XAI can be applied in a variety of contexts, Davis’ research focuses on applying it to AI models for drug discovery, such as those used to predict new antibiotic candidates. Considering that thousands of candidate molecules can be screened and rejected to approve just one new drug — and antibiotic resistance is a continuous threat to the efficacy of existing drugs — accurate and efficient prediction models are critical. “I want to use XAI to better understand what information we need to teach computers chemistry,” says Hunter Sturm, a graduate student in chemistry in Davis’ lab who’s presenting the work at the meeting.
The researchers started their work by feeding databases of known drug molecules into an AI model that would predict whether a compound would have a biological effect. Then, they used an XAI model developed by collaborator Pascal Friederich at Germany’s Karlsruhe Institute of Technology to examine the specific parts of the drug molecules that led to the model’s prediction. This helped explain why a particular molecule had activity or not, according to the model, and that helped Davis and Sturm understand what an AI model might deem important and how it creates categories once it has examined many different compounds.
The researchers realized that XAI can see things that humans might have missed; it can consider far more variables and data points at once than a human brain. For example, when screening a set of penicillin molecules, the XAI found something interesting. “Many chemists think of penicillin’s core as the critical site for antibiotic activity,” says Davis. “But that’s not what the XAI saw.” Instead, it identified structures attached to that core as the critical factor in its classification, not the core itself. “This might be why some penicillin derivatives with that core show poor biological activity,” explains Davis.
In addition to identifying important molecular structures, the researchers hope to use XAI to improve predictive AI models. “XAI shows us what computer algorithms define as important for antibiotic activity,” explains Sturm. “We can then use this information to train an AI model on what it’s supposed to be looking for,” Davis adds.
Next, the team will partner with a microbiology lab to synthesize and test some of the compounds the improved AI models predict would work as antibiotics. Ultimately, they hope XAI will help chemists create better, or perhaps entirely different, antibiotic compounds, which could help stem the tide of antibiotic-resistant pathogens.
“AI causes a lot of distrust and uncertainty in people. But if we can ask AI to explain what it’s doing, there’s a greater likelihood that this technology will be accepted,” says Davis.
Sturm adds that he thinks AI applications in chemistry and drug discovery represent the future of the field. “Someone needs to lay the foundation. That’s what I hope I’m doing.”
The research was funded by the University of Manitoba, the Canadian Institutes of Health Research and the Digital Research Alliance of Canada.
A Q&A with the researcher will be posted on Sunday, Aug. 18. Reporters can access the video during the embargo period, and once the embargo is lifted the same URL will allow the public to access the content. Visit the ACS Fall 2024 program to learn more about this presentation, “Using Explainable Artificial Intelligence to explore the relationship between structure and activity,” and other science presentations.
###
The American Chemical Society (ACS) is a nonprofit organization chartered by the U.S. Congress. ACS’ mission is to advance the broader chemistry enterprise and its practitioners for the benefit of Earth and all its people. The Society is a global leader in promoting excellence in science education and providing access to chemistry-related information and research through its multiple research solutions, peer-reviewed journals, scientific conferences, eBooks and weekly news periodical Chemical & Engineering News. ACS journals are among the most cited, most trusted and most read within the scientific literature; however, ACS itself does not conduct chemical research. As a leader in scientific information solutions, its CAS division partners with global innovators to accelerate breakthroughs by curating, connecting and analyzing the world’s scientific knowledge. ACS’ main offices are in Washington, D.C., and Columbus, Ohio.
Registered journalists can subscribe to the ACS journalist news portal on EurekAlert! to access embargoed and public science press releases. For media inquiries, contact newsroom@acs.org.
Note to journalists: Please report that this research was presented at a meeting of the American Chemical Society. ACS does not conduct research, but publishes and publicizes peer-reviewed scientific studies.
Follow us: X, formerly Twitter | Facebook | LinkedIn | Instagram
Title
Using explainable artificial intelligence to explore the relationship between structure and activity
Abstract
Antibiotic resistance is a threat to society and novel antibiotics are needed in order to combat this issue. To accelerate antibiotic discovery, researchers have begun to use Machine Learning (ML) in the identification of new antibiotics. However, the downfall of many ML methods is that they are black boxes, meaning we know which compounds are identified without knowing why the algorithm has selected said compounds. This research aims to better understand ML methods in antibiotic discovery by expanding upon our previous work using eXplainable Artificial Intelligence (XAI) to now help understand what features of small molecules computers deem important for the prediction of antibiotic activity. XAI, as the name suggests, is a type of ML method wherein the output of the model contains an explanation as to why the results are classified in the way they are. Using publicly available databases and models, libraries of compounds with known (in)activity have been studied and explanations analyzed as to why our model considers a small molecule as having antibiotic activity or not. Alternatively, once it is known what features are important can a ML model be trained to accurately predict multiple classes of antibiotics.
What's Your Reaction?
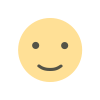
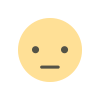
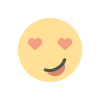
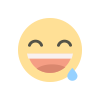
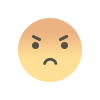
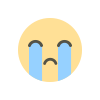
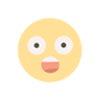