NTT Research Collaborates with Harvard Scientists to Enhance Biohybrid Ray Development Using Machine Learning
The Harvard John A. Paulson School of Engineering and Applied Sciences (SEAS), in collaboration with NTT Research, Inc., has made significant strides in the realm of biohybrid robotics thanks to the innovative application of machine-learning directed optimization (ML-DO). Their recent findings showcase a breakthrough method that efficiently navigates the intricate search for optimal design configurations, […]

The Harvard John A. Paulson School of Engineering and Applied Sciences (SEAS), in collaboration with NTT Research, Inc., has made significant strides in the realm of biohybrid robotics thanks to the innovative application of machine-learning directed optimization (ML-DO). Their recent findings showcase a breakthrough method that efficiently navigates the intricate search for optimal design configurations, specifically in the realm of biohybrid robots, which are synthetic organisms that meld biological components with artificial materials. The researchers focused on creating mini biohybrid rays composed of cardiomyocytes—heart muscle cells—combined with rubber materials. These biohybrid rays possess a wingspan of roughly 10 millimeters and boast swimming efficiency that is nearly double that of their predecessors, which were developed using traditional biomimetic methods.
The research team was spearheaded by John Zimmerman, a postdoctoral fellow at Harvard SEAS. He was joined by a distinguished group of researchers, including Ryoma Ishii, a medical and health informatics scientist at NTT Research, and Kevin Kit Parker, the Tarr Family Professor of Bioengineering and Applied Physics at Harvard SEAS. The collaborative effort also included contributions from the Harvard SEAS Disease Biophysics Group, which Parker leads. Their joint efforts culminated in the publication of a paper in the journal Science Robotics titled “Bioinspired Design of a Tissue Engineered Ray with Machine Learning.”
The core of this research addresses a pressing question in the development of biohybrid robots, particularly focusing on the marine ray model: How do researchers select fin geometries that are effective in novel working environments while still adhering to the natural scaling laws associated with swimming speed and efficiency? Ishii articulated this query, emphasizing the challenges faced in designing biohybrid robots that effectively emulate real biological forms.
Traditional biomimetic approaches in engineering biohybrids often involve replicating existing biological structures, an approach that can come with inherent limitations. For example, when designing biohybrid equivalents of batoid fishes—like skates and rays—engineers grapple with the vast range of natural aspect ratios and fin shapes. Determining which characteristics to replicate can be daunting, and existing models may sometimes overlook essential biomechanical principles that dictate swimming performance. This can be detrimental, leading to designs that squander muscle mass or fail to achieve optimal swimming velocities.
Given these obstacles, the research team sought an innovative pathway to determine fin geometries that can excel under varying conditions, without violating the principles of natural scaling laws. They turned to the realm of machine learning as a potential solution to optimize the design process effectively. The interdisciplinary nature of this endeavor meant that traditional computational modeling techniques would likely require excessive computational power. This led the team to the hypothesis that ML-DO could permit a more systematic and efficient exploration of design options that maximize swimming speeds.
To validate their hypothesis, the researchers undertook a structured approach that unfolded in three pivotal steps. Initially, they developed an algorithm capable of expressing a wide array of fin geometries. This was followed by the formulation of a generalized ML-DO framework aimed at navigating the expansive and often disjointed configuration space of available designs. Finally, the team employed this methodology to pinpoint biohybrid fin geometries that favorably impacted performance by allowing for smooth and efficient aquatic movement.
The insightful results gleaned from the ML-DO approach provided quantitative evidence regarding fin structure-function correlations while simultaneously reconstructing prevalent patterns found in the morphology of open-sea batoid species. Significantly, the team emerged with a superior design concept: fins characterized by large aspect ratios paired with delicately tapered tips, which proved to be versatile across various swimming scales. Utilizing these findings, the team successfully engineered biohybrid mini-rays that utilized cardiac muscle tissue and demonstrated impressive self-propelled swimming capabilities at the millimeter scale. These mini-rays showcased swimming efficiencies that were approximately double those observed in previous biomimetic designs.
While the outcomes from the current study are commendable, researchers acknowledge that further advancements must be made to reconcile the discrepancies between engineered devices and naturally occurring marine organisms—particularly regarding efficiency. The devices showcased in the study, though superior to their biomimetic counterparts, still exhibited a slightly lower efficiency compared to natural marine life, highlighting the room for continued refinement in their designs.
Looking forward, the research team anticipates ongoing developments in biohybrid robotics that will have a significant impact on various applications. These include but are not limited to deploying remote sensors, creating probes for hostile environments, and designing vehicles for therapeutic delivery. The ML-DO-informed methodology is hoped to provide insights that parallel the selective pressures faced by natural evolution, giving researchers a deeper understanding of the factors that shape biological tissues in both healthy and pathological states.
Furthermore, this research is poised to contribute to the burgeoning field of 3D organ biofabrication, aiding in the eventual goal of creating complex structures such as biohybrid hearts. Parker, highlighting the collaborative nature of this research, noted that a joint research agreement established two years ago with NTT Research aimed to enhance understanding of cardiac physiology while driving the development of biohybrid devices. He expressed excitement regarding the progress made thus far and the potential future accomplishments stemming from this partnership.
In 2022, a significant three-year joint research agreement was formalized between NTT Research and Harvard SEAS, focusing on engineering a model of the human heart while investigating the fundamental principles that govern muscular pumps. The goal is to leverage their findings to contribute to a cardiovascular bio digital twin model—an innovative fusion of biological understanding and engineering prowess that could pave the way for new advancements in medical technology.
This research not only illuminates new avenues in bioengineering but emphasizes the transformative potential of machine learning in optimizing biological designs. As researchers continue to explore, the marriage of biological inspiration and artificial intelligence may yield unprecedented innovations, leading to breakthroughs not only in robotics but also in our comprehension of biological systems and their applications in medicine and human health.
Subject of Research: Development of biohybrid robots using machine-learning directed optimization
Article Title: Bioinspired Design of a Tissue Engineered Ray with Machine Learning
News Publication Date: October 2023
Web References: Science Robotics
References: None provided
Image Credits: None provided
Keywords: Machine learning, biohybrid robots, biomimetics, tissue engineering, robotics, cardiac muscle, swimming efficiency, design optimization.
Tags: advancements in swimming efficiencybiohybrid roboticsbiomimetic design improvementscardiomyocytes in roboticsHarvard NTT Research collaborationHarvard SEAS research contributionsinnovative applications of ML-DOinterdisciplinary bioengineering researchmachine learning in bioengineeringmini biohybrid rays developmentoptimization of biohybrid designssynthetic organisms research
What's Your Reaction?
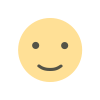
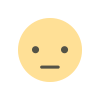
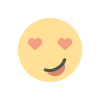
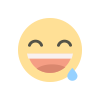
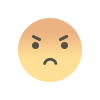
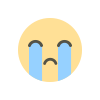
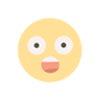