Neural Networks Predict Stress-Strain in Porous Materials
In a groundbreaking advance at the crossroads of materials science and artificial intelligence, a team of researchers has unveiled a novel approach to predict the compressive stress-strain behavior of elasto-plastic porous media. This development, led by Lindqwister, Peloquin, Dalton, and colleagues, hinges on morphology-informed neural networks that bring unprecedented accuracy and efficiency to simulating complex […]

In a groundbreaking advance at the crossroads of materials science and artificial intelligence, a team of researchers has unveiled a novel approach to predict the compressive stress-strain behavior of elasto-plastic porous media. This development, led by Lindqwister, Peloquin, Dalton, and colleagues, hinges on morphology-informed neural networks that bring unprecedented accuracy and efficiency to simulating complex mechanical responses in porous materials. The implications for fields ranging from civil engineering to biomedical implants are vast, reflecting an exciting shift where AI paradigms intimately understand physical microstructures to forecast macroscopic material behavior.
Porous media — materials characterized by networks of voids or pores interspersed within a solid matrix — present formidable challenges to traditional mechanical modeling. Their irregular morphologies cause stress distribution and deformation behaviors to deviate significantly from homogeneous solids. Historically, capturing their elasto-plastic compressive responses has relied on computationally expensive finite element methods and empirically derived models that often fail to fully integrate microstructural information. The morphology of pore spaces, including shape, connectivity, and size distribution, plays a crucial role in dictating mechanical properties under load, yet leveraging this intricate morphology explicitly in constitutive models has remained elusive.
The team’s approach capitalizes on the recent surge of machine learning’s capabilities, designing neural networks that are informed directly by morphological descriptors extracted from microstructural images or synthetic representations. Unlike traditional black-box models that predict mechanical responses solely from bulk parameters, these networks embed spatial and morphological context, capturing subtle influences that dictate elasto-plastic behavior. This allows for learning complex, nonlinear mappings between microstructure and the resulting stress-strain response, significantly outperforming prior methods in both accuracy and computational speed.
To achieve this, the researchers first amassed a comprehensive dataset amalgamating synthetic porous structures and corresponding mechanical responses simulated via high-fidelity computational mechanics tools. Each sample’s morphology was quantified through advanced image analysis techniques, characterizing features such as pore volume fraction, shape anisotropy, and connectivity metrics. These form the input feature space for the neural network, which is architected to process these descriptors hierarchically and extract salient patterns that relate morphology to mechanical behavior. Through rigorous training and validation cycles, the network iteratively refines its parameters, ultimately developing a robust predictive model capable of generalizing across a wide array of microstructural variations.
One of the key innovations of this work is the integration of elasto-plastic constitutive behavior directly into the learning framework. Porous media often exhibit nonlinear stress-strain responses characterized by initial elasticity, yielding, and subsequent plastic deformation, phenomena that pose complex challenges for conventional modeling. By feeding the neural network with morphology-driven inputs and coupling them with elasto-plastic constitutive principles, the model internalizes not only structural responses but also fundamental material physics, enabling it to predict stress-strain curves with remarkable fidelity.
The practical impact of this capability cannot be overstated. Porous materials underpin numerous applications—from lightweight structural components in aerospace and automotive industries to bone scaffolds in medical implants and filtration membranes in chemical processing. Accurately predicting their mechanical responses under compression is essential for optimizing designs and ensuring performance reliability. By dramatically accelerating the evaluation process while preserving accuracy, morphology-informed neural networks promise to slash development cycles and reduce costs, all while empowering engineers with deeper insights into the structure-property relationships governing these complex materials.
Moreover, the researchers highlight that their method affords a degree of interpretability often lacking in AI-driven material models. By linking learned features back to morphological descriptors, the model not only predicts outcomes but also elucidates which microstructural traits most heavily influence performance. This opens avenues for guided materials design, where engineers can tailor microstructures to target desirable mechanical properties—ushering in a new paradigm of morphology-informed materials engineering driven by data-centric intelligence.
The study also investigates the network’s robustness across different scales of morphology and varying porous architectures. Testing against diverse microstructures, including randomly distributed pores and more ordered cellular constructs, the neural network adapts effectively, underscoring its versatility. This adaptability is crucial since porous media span an extraordinary range of configurations—biological tissues, engineered foams, and geological formations—each exhibiting unique morphological signatures that must be accommodated for accurate stress-strain prediction.
Critically, the framework provides real-time predictions, an enormous advantage over traditional numerical simulations that require hours or days per sample. This opens possibilities for integration within iterative design workflows and real-time monitoring scenarios, such as in-situ assessment of implant loading or structural health monitoring in porous components under service conditions. Engineers can promptly evaluate how morphological modifications will influence mechanical resilience under compression, enabling rapid design iteration previously impossible with conventional computational methods.
While this work centers on compressive stress-strain behavior, the authors suggest their morphology-informed neural network approach is extensible to other mechanical tests such as tension, shear, and cyclic loading. Given that porous media often experience multiaxial loading states in real-world applications, this adaptability further enhances the method’s utility. Furthermore, their general framework is poised to incorporate additional physical effects like damage evolution, fracture propagation, and time-dependent viscoplasticity, paving the way for comprehensive predictive capabilities in porous media mechanics.
This research exemplifies how harnessing machine learning in conjunction with physical morphology can overcome longstanding limitations in materials modeling. By bridging microstructural characteristics and macroscopic mechanical responses through a data-driven yet physically grounded approach, the study charts a promising course toward smarter, faster, and more insightful engineering of porous materials.
In conclusion, Lindqwister, Peloquin, Dalton, and their collaborators have delivered an exciting leap forward in predictive modeling of porous media mechanics. Their morphology-informed neural network model holds remarkable promise for revolutionizing how engineers simulate and optimize elasto-plastic compressive behavior in these ubiquitous and technologically critical materials. As AI continues to reshape scientific discovery, this work stands as a vivid testament to the profound gains achievable when data-driven models are thoughtfully integrated with domain-specific physical insights.
Subject of Research:
Predictive modeling of compressive stress-strain behavior in elasto-plastic porous media using morphology-informed neural networks.
Article Title:
Predicting compressive stress-strain behavior of elasto-plastic porous media via morphology-informed neural networks.
Article References:
Lindqwister, W., Peloquin, J., Dalton, L.E. et al. Predicting compressive stress-strain behavior of elasto-plastic porous media via morphology-informed neural networks. Commun Eng 4, 73 (2025). https://doi.org/10.1038/s44172-025-00410-9
Image Credits: AI Generated
Tags: advancements in civil engineering materialsAI in materials sciencebiomedical applications of porous materialscompressive behavior of porous materialscomputational modeling innovationselasto-plastic modeling techniquesmachine learning for mechanical responsesmicrostructural analysis in engineeringmorphology-informed neural networksneural networks for stress-strain predictionporous media mechanical propertiespredicting material behavior with AI
What's Your Reaction?
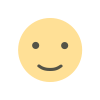
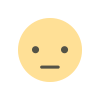
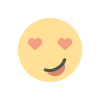
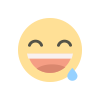
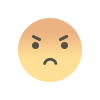
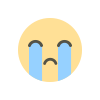
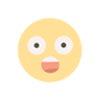