MSK Research Highlights, April 26, 2024
New research from Memorial Sloan Kettering Cancer Center (MSK) provides two examples of computational tools developed at MSK that leverage data about cells’ locations to expand our understanding of cancer. One approach describes cellular “neighborhoods” not just in terms of which cell types are present, but also what the cells are doing and expressing. The […]
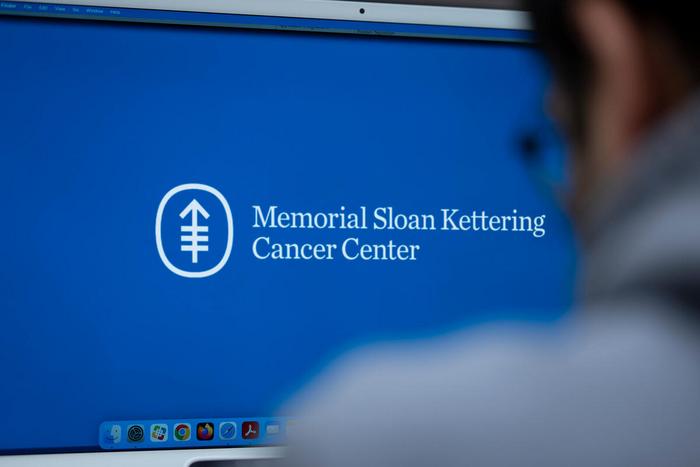
New research from Memorial Sloan Kettering Cancer Center (MSK) provides two examples of computational tools developed at MSK that leverage data about cells’ locations to expand our understanding of cancer. One approach describes cellular “neighborhoods” not just in terms of which cell types are present, but also what the cells are doing and expressing. The other can determine which immune cells are present and active in specific regions of a tumor.
Credit: Memorial Sloan Kettering Cancer Center
New research from Memorial Sloan Kettering Cancer Center (MSK) provides two examples of computational tools developed at MSK that leverage data about cells’ locations to expand our understanding of cancer. One approach describes cellular “neighborhoods” not just in terms of which cell types are present, but also what the cells are doing and expressing. The other can determine which immune cells are present and active in specific regions of a tumor.
Modeling cellular neighborhoods using spatial data
New high-resolution spatial profiling technologies are making it possible to investigate how cells interact with their neighbors within a particular tissue. Existing computational approaches, however, tend to discard most of the information in the data by describing cellular neighborhoods only in terms of which cell types are present, rather than what these cells are doing and expressing.
To overcome these limitations, a research team led by the lab of MSK’s Dana Pe’er, PhD, developed a computational framework that produces a rich, detailed picture of coordinated gene expression within a spatial context. The team — which brought together expertise in machine-learning, cancer immunology and developmental biology — developed two methods, which they call COVET and ENVI.
COVET (for covariance environment) provides a mathematical representation of the cellular environment that is robust and expressive, allowing it to capture the complex heterogeneity within cellular neighborhoods at the level of gene activity. It can be plugged directly into the suite of widely used, powerful tools that exist for single-cell analysis, bringing interpretable spatial analysis within the reach of every researcher.
ENVI (for environmental variational inference) is an AI approach that incorporates COVET and integrates spatial and single-cell data to create a more comprehensive picture of the tissue environment. “The algorithm provides superior performance for imputing missing gene expression in spatial modalities; it scales to millions of cells; and it has the distinctive ability to infer the spatial context of dissociated cells, even across multiple cell types in complex tissues,” the study authors write.
In a related research briefing, Nature Biotechnology editors note: “While there are an abundance of computational analysis tools for spatial transcriptomics data, this paper stood out because of the innovative way in which spatial correlations are modeled.”
Learn more in Nature Biotechnology.
Picturing immune cell activity in tumor neighborhoods
One that way tumors protect themselves from immune attack is by manipulating the noncancerous cells around them. This includes immune T cells that flock to the tumors and infiltrate them, including regulatory T cells that tamp down the immune attack. But tumors are known to be heterogeneous, with different types of cancer cells in different spots. This heterogeneity of tumors appears to be reflected in heterogeneity of the immune cells in their midst.
A team led by MSK breast surgeon George Plitas, MD, immunologist Alexander Rudensky, PhD, and biomedical engineer Elham Azizi, PhD, at Columbia University developed a computational tool called Starfysh that can determine which immune cell types are present and active in specific regions of a tumor. Using Starfysh to analyze aggressive breast tumors, the researchers found that different tumor neighborhoods have different cellular interactions. For example, tumor regions that are hypoxic (have low-oxygen levels) seem to attract more regulatory T cells.
“Defining these interactions on a spatial level can be very informative in terms of how we understand tumor biology,” Dr. Plitas says. “The biomarkers we have for predicting response to immunotherapy are crude. But with this kind of technology, we could potentially start to define it on an individual level for each person.”
Read more in Nature Biotechnology.
Journal
Nature Biotechnology
What's Your Reaction?
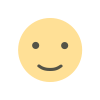
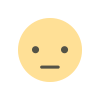
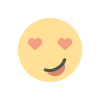
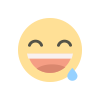
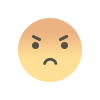
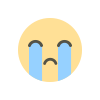
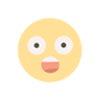