MRI Radiomics Predicts EGFR Mutations Accurately
In the rapidly evolving landscape of oncology, the integration of advanced imaging techniques with computational analysis has illuminated new pathways for personalized cancer diagnostics. One such innovation, MRI radiomics, is emerging as a transformative tool in predicting genetic mutations that drive tumor behavior. A recent comprehensive systematic review and meta-analysis published in BioMedical Engineering OnLine […]

In the rapidly evolving landscape of oncology, the integration of advanced imaging techniques with computational analysis has illuminated new pathways for personalized cancer diagnostics. One such innovation, MRI radiomics, is emerging as a transformative tool in predicting genetic mutations that drive tumor behavior. A recent comprehensive systematic review and meta-analysis published in BioMedical Engineering OnLine sheds light on the promising potential of MRI radiomics, particularly focused on extrapulmonary metastases, to noninvasively predict epidermal growth factor receptor (EGFR) mutations in non-small cell lung cancer (NSCLC).
EGFR mutations have long been recognized as critical markers in NSCLC, influencing tumor progression, metastasis, and responses to targeted therapies. Traditionally, molecular testing to detect these mutations relies on biopsies from the primary lung tumor. However, challenges arise when the primary lesion is either surgically removed or inaccessible for sampling, necessitating alternative methods to assess mutation status. This study explores the feasibility of utilizing MRI radiomic features extracted from metastatic lesions outside the lungs, offering a novel window into the tumor’s genetic landscape.
Radiomics, the high-throughput extraction of quantitative features from medical images, transforms standard MRI scans into complex datasets reflecting tumor heterogeneity, texture, shape, and intensity variations. These multidimensional signatures can correlate with underlying genetic alterations such as EGFR mutations. By systematically aggregating data from thirteen studies encompassing 2369 metastatic NSCLC cases, the authors rigorously evaluated the diagnostic performance of MRI radiomics in this context.
The meta-analysis reveals a compelling diagnostic accuracy for MRI radiomics when applied to extrapulmonary metastatic lesions. For predicting EGFR mutations in primary NSCLC lesions, the pooled area under the curve (AUC) reaches an impressive 0.90, accompanied by a sensitivity of 0.80 and specificity of 0.85. These values indicate a high level of discrimination, potentially surpassing radiomics analyses directly targeting primary tumors. This finding suggests that extrapulmonary metastatic sites may harbor radiomic features reflective of the primary tumor’s mutational status, thus serving as reliable surrogates.
When focusing on the prediction of EGFR mutations within metastatic lesions themselves, the pooled AUC remains robust at 0.86, with sensitivity and specificity values each around 0.79. This moderate yet respectable performance underscores the utility of MRI radiomics in assessing metastatic tumor biology, further supporting its clinical application where tissue biopsy may be invasive or unsafe.
The review highlights the multifaceted nature of MRI radiomics as an adjunct to molecular assays. Unlike conventional biopsy methods limited by sampling bias and procedural risks, this noninvasive imaging approach offers a holistic overview of tumor heterogeneity across multiple metastatic sites. The ability to glean mutational insights from routinely acquired MRI scans could revolutionize diagnostic workflows, enabling real-time monitoring of mutation status and potentially guiding therapeutic decisions without additional invasive interventions.
Technically, radiomics harnesses sophisticated image processing algorithms to extract features such as histogram-based intensity metrics, texture analysis measures like gray-level co-occurrence matrices, and morphological characteristics. Machine learning models then integrate these features to classify mutation status with increasing accuracy. The reviewed studies utilized diverse MRI sequences, including T1- and T2-weighted images, emphasizing the adaptability of radiomics across imaging protocols.
The implications of this research extend beyond NSCLC. The methodology exemplifies how quantitative imaging can bridge gaps between radiology and genomics, paving the way for precision oncology. As research in this field accelerates, harmonization of imaging acquisition, feature extraction standards, and robust validation across multicenter cohorts remain essential to translate these findings into routine clinical use.
Moreover, MRI radiomics could enhance patient stratification for targeted EGFR therapies, such as tyrosine kinase inhibitors, by providing rapid and repeatable assessments of mutation dynamics, especially in metastatic disease contexts where tumor biology may evolve. This dynamic monitoring may improve outcomes through timely adjustments in treatment strategies and reduce reliance on invasive biopsy procedures.
However, challenges such as reproducibility, feature selection bias, and model interpretability warrant cautious optimism. The heterogeneity of included studies in terms of MRI protocols and analysis pipelines in the meta-analysis highlights the need for standardized radiomic workflows. Future prospective studies with larger cohorts and integration of artificial intelligence-driven analytics hold promise to refine predictive performance further.
In conclusion, this comprehensive review elucidates the considerable potential of MRI radiomics applied to extrapulmonary metastases for predicting EGFR mutations in NSCLC. By offering a high-precision, noninvasive complement to molecular diagnostics, this approach stands poised to augment current clinical practice, promoting more personalized and adaptive oncological care. As oncology increasingly embraces multi-omics and advanced imaging integration, MRI radiomics emerges at the forefront of this paradigm shift, heralding a new era in the molecular characterization of cancer.
Subject of Research: MRI Radiomics for Predicting EGFR Mutations in NSCLC Based on Extrapulmonary Metastases
Article Title: The potential of MRI radiomics based on extrapulmonary metastases in predicting EGFR mutations: a systematic review and meta-analysis
Article References:
Wu, L., Wei, D., Li, S. et al. The potential of MRI radiomics based on extrapulmonary metastases in predicting EGFR mutations: a systematic review and meta-analysis. BioMed Eng OnLine 24, 4 (2025). https://doi.org/10.1186/s12938-025-01331-6
Image Credits: AI Generated
DOI: https://doi.org/10.1186/s12938-025-01331-6
Tags: advanced imaging techniques in oncologycomputational analysis in radiologyextrapulmonary metastases imagingmolecular testing alternatives for lung cancerMRI radiomics for cancer diagnosticsnoninvasive cancer mutation analysispersonalized cancer treatment strategiespredicting EGFR mutations in NSCLCsystematic review of MRI in cancertargeted therapies for EGFR mutations.tumor heterogeneity assessment with MRIunderstanding tumor behavior through imaging
What's Your Reaction?
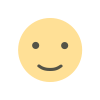
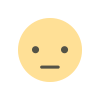
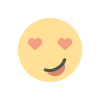
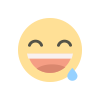
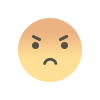
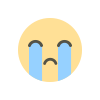
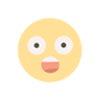