Machine Learning Unveils Bladder Cancer Stemness
In a groundbreaking advancement in bladder cancer research, scientists have unveiled a novel machine learning-based framework that deciphers the complex stemness features of this elusive disease, offering new horizons for personalized treatment strategies. Bladder cancer, notorious for its heterogeneity and unpredictable clinical outcomes, has long confounded oncologists striving for reliable prognostic indicators and targeted therapies. […]

In a groundbreaking advancement in bladder cancer research, scientists have unveiled a novel machine learning-based framework that deciphers the complex stemness features of this elusive disease, offering new horizons for personalized treatment strategies. Bladder cancer, notorious for its heterogeneity and unpredictable clinical outcomes, has long confounded oncologists striving for reliable prognostic indicators and targeted therapies. At the heart of this complexity lies the concept of cancer stem cells, a subpopulation of cells driving tumor initiation, progression, and resistance to treatments. Understanding the stemness – or the intrinsic ability of tumor cells to self-renew and sustain malignancy – has become a pivotal challenge. The latest study leverages sophisticated computational techniques to classify bladder cancer patients into distinct stemness subtypes, with profound implications for prognosis and therapy.
The researchers commenced by harnessing large-scale bladder cancer datasets from The Cancer Genome Atlas (TCGA) and the Gene Expression Omnibus (GEO), integrating these extensive molecular profiles with curated stemness gene sets sourced from the StemChecker database. Employing consensus clustering, a robust machine learning algorithm, they segmented patients based on the enrichment scores of stemness-related genes. This approach transcended traditional sampling biases, enabling a reproducible and biologically meaningful classification reflecting underlying tumor biology. Simultaneously, the team applied the One-Class Logistic Regression (OCLR) algorithm to compute the mRNA expression-based stemness index (mRNAsi), quantifying the self-renewal capacity of each tumor sample at a molecular level.
The meticulous analysis unearthed two discrete bladder cancer stemness subtypes, each characterized by distinctive genomic, immunologic, and therapeutic response profiles. Patients categorized within the first subtype exhibited elevated mRNAsi scores that paradoxically correlated with more favorable overall survival. This subtype manifested an immunologically active tumor microenvironment, hallmarked by abundant antitumor immune cell infiltration, potentially enhancing responsiveness to emerging immunotherapies. Conversely, the second subtype demonstrated marked genomic instability, including increased aneuploidy and homologous recombination defects, coupled with a heightened tumor mutation burden. Clinically, this group showed increased susceptibility to conventional chemotherapeutic agents rather than immunotherapy, underscoring the heterogeneity of treatment responses linked to tumor stemness.
Driven by the imperative for clinical translation, the team constructed a predictive classifier distinguishing these stemness subtypes through a rigorous machine learning paradigm incorporating LASSO regression, random forest algorithms, and multivariate logistic regression. This multifaceted strategy distilled an initial pool of candidates to six key differentially expressed genes with the highest predictive power. Validation across multiple independent GEO datasets and two non-muscle invasive bladder cancer cohorts confirmed the classifier’s robustness and prognostic accuracy, solidifying its potential as a practical tool in clinical oncology to stratify patients for tailored therapies.
Among the pivotal classifier genes, TNFAIP6 emerged as a critical mediator of bladder cancer stemness, verified through experimental assays including tumor sphere formation and western blot analyses. Silencing of TNFAIP6 significantly impaired the stem-like properties of bladder cancer cells, substantiating its functional importance. Intriguingly, TNFAIP6 knockdown also sensitized tumor cells to frontline chemotherapeutic drugs such as cisplatin, docetaxel, and paclitaxel, suggesting an actionable vulnerability that could be exploited to overcome chemoresistance. Moreover, repression of TNFAIP6 led to downregulation of the immune checkpoint gene PD-L1, highlighting its potential role in modulating tumor immune evasion.
The integration of computational predictions with wet-lab validations underscores a paradigm shift in cancer research, where data-driven discoveries refine our molecular understanding and catalyze therapeutic innovations. By elucidating the dualistic nature of bladder cancer stemness—where subtype 1’s immune-engaged state contrasts with subtype 2’s genomic instability-driven vulnerability—the study opens new avenues to personalize treatment protocols. Precision oncology, long the aspirational frontier, stands to benefit immensely from such stratified approaches, ensuring patients receive immunotherapy or chemotherapy tailored to their tumor’s stemness landscape.
Importantly, this research sheds light on the intricate interplay between tumor stemness and the tumor microenvironment. The antitumor immune milieu observed in stemness subtype 1 could be leveraged to optimize immunotherapeutic regimens, including checkpoint inhibitors that have revolutionized bladder cancer care in recent years. Simultaneously, patients harboring tumors classified within subtype 2 might gain enhanced efficacy through DNA damage repair-targeted therapies given their homologous recombination deficiencies, complementing standard chemotherapy.
Beyond prognostic and predictive dimensions, this study positions TNFAIP6 as a promising molecular target for future drug development. Its role in sustaining stemness and modulating immune checkpoints implicates it as a dual facilitator of tumor progression and immune suppression. Therapeutic strategies aimed at TNFAIP6 inhibition could potentially dismantle cancer stem cell reservoirs while improving the tumor’s immunogenicity, thus creating synergistic effects with existing modalities.
The authors acknowledge the complexity intrinsic to cancer stemness, emphasizing that the binary classification, while enlightening, represents a simplification of a spectrum of cellular states within bladder tumors. Nevertheless, the reproducibility of the classifier across diverse cohorts and the corroborative functional assays signify a robust framework for subsequent translational studies. Further investigations will undoubtedly refine these insights, possibly incorporating single-cell transcriptomics and proteomics to resolve heterogeneity at an even finer scale.
Clinical integration of this stemness subtype classifier may revolutionize patient management by enabling oncologists to predict not only prognosis but also optimal therapeutic avenues before treatment initiation. This preemptive stratification will minimize unnecessary exposure to ineffective therapies, reduce adverse effects, and improve survival rates. Additionally, the classifier could serve as a dynamic biomarker for monitoring therapeutic response and disease progression, underpinning adaptive treatment strategies.
From a broader perspective, the melding of machine learning with molecular oncology exemplifies the transformative potential of artificial intelligence in medicine. As datasets burgeon and computational algorithms mature, similar approaches could redefine classification schemas across myriad cancers, tailoring therapies with unprecedented precision.
In conclusion, this pioneering study offers compelling evidence that the molecular characterization of bladder cancer stemness through machine learning not only enhances our biological comprehension but also provides tangible clinical tools. The identification of two distinct stemness subtypes, coupled with a validated genetic classifier and functional exploration of TNFAIP6, lays the groundwork for next-generation therapies. As the oncology community grapples with the challenges of tumor heterogeneity and treatment resistance, such integrative, data-driven innovations herald a new chapter in the fight against bladder cancer.
Subject of Research: Bladder cancer stemness characterization and molecular classification using machine learning algorithms.
Article Title: Machine learning-based characterization of stemness features and construction of a stemness subtype classifier for bladder cancer.
Article References:
Qiu, H., Deng, X., Zha, J. et al. Machine learning-based characterization of stemness features and construction of a stemness subtype classifier for bladder cancer. BMC Cancer 25, 717 (2025). https://doi.org/10.1186/s12885-025-14109-9
Image Credits: Scienmag.com
DOI: https://doi.org/10.1186/s12885-025-14109-9
Tags: advancements in bladder cancer researchbiological classification of tumorsbladder cancer prognosis and treatmentcancer stem cells in bladder cancercomputational techniques in cancer classificationconsensus clustering in cancer researchGene Expression Omnibus data integrationlarge-scale cancer datasets analysismachine learning in cancer researchpersonalized treatment strategies for cancerstemness features in oncologyThe Cancer Genome Atlas bladder cancer
What's Your Reaction?
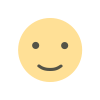
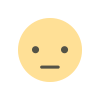
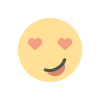
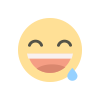
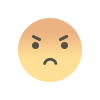
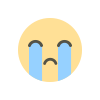
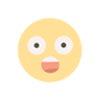