Machine Learning Unlocks Cellular Condensate Localization Beyond Peptides
In the rapidly evolving landscape of cellular biology, understanding the precise mechanisms that dictate the localization of proteins within the complex milieu of the cell remains one of the great challenges. A groundbreaking study by Ditlev and Forman-Kay, published in Cell Research in 2025, pushes the boundaries of our knowledge by leveraging machine learning to […]

In the rapidly evolving landscape of cellular biology, understanding the precise mechanisms that dictate the localization of proteins within the complex milieu of the cell remains one of the great challenges. A groundbreaking study by Ditlev and Forman-Kay, published in Cell Research in 2025, pushes the boundaries of our knowledge by leveraging machine learning to decode how proteins are targeted to cellular condensates beyond classical peptide sequences. This transformative approach marks a pivotal shift, inviting the scientific community to reconsider traditional paradigms of protein localization and the molecular grammar that governs intracellular compartmentalization.
The traditional dogma posits that short peptide sequences—often termed targeting or localization signals—serve as the primary barcodes instructing proteins where to reside within the crowded cellular environment. These motifs guide proteins to well-characterized organelles such as the nucleus, mitochondria, and endoplasmic reticulum. However, the discovery of membraneless organelles, also known as biomolecular condensates, has complicated the narrative. These condensates form through phase separation processes, allowing dynamic and reversible compartmentalization without membrane boundaries. The rules governing protein residency within these condensates have remained elusive, predominantly because they lack canonical sorting signals.
Ditlev and Forman-Kay’s study boldly ventures beyond the peptide targeting sequences to develop a comprehensive machine learning framework that captures the subtle, multifaceted sequence features contributing to condensate localization. By integrating high-throughput proteomic datasets with sophisticated computational algorithms, the researchers decode intricate patterns that hint at the molecular determinants enabling proteins to phase-separate and condense selectively in specific cellular contexts. This approach heralds a paradigm where traditional sequence motifs are supplemented—and sometimes superseded—by emergent biophysical properties encoded within amino acid composition and sequence architecture.
Central to their methodology is the utilization of deep learning models, computational structures inspired by neural networks, capable of identifying nonlinear relationships and complex patterns in large datasets. Training these models on experimentally validated datasets of proteins known to localize within various condensates enables the extraction of nuanced determinants beyond simple motif recognition. For instance, intrinsic disorder regions, sequence charge distribution, aromatic residue content, and multivalent interaction motifs collectively inform the predictive framework. This multilayered feature space allows for remarkable accuracy in predicting protein condensate residency, opening doors not just for identification but also for rational engineering of phase behavior.
The implications for cell biology are profound. Accurately mapping the sequence-encoded determinants of condensate localization transforms our understanding of intracellular organization, particularly concerning the spatial-temporal dynamics that underpin key processes like gene expression regulation, signal transduction, and stress responses. Many condensates, such as P-bodies, stress granules, and nucleoli, serve as hubs for critical biochemical reactions. Disruptions in their formation or composition are increasingly linked to pathological states including neurodegeneration and cancer. By illuminating the molecular grammar of condensate targeting, this study offers mechanistic insights that could drive therapeutic innovation.
Moreover, this research provides a fresh vantage point on the evolutionary pressures shaping protein sequences. It suggests that natural selection not only fine-tunes canonical targeting signals but also sculpts broader physicochemical features to facilitate appropriate condensate localization. The study’s findings hint at a hidden layer of evolutionary information, revealing how proteins have adapted sequence properties to navigate the complex intracellular landscape and dynamically partition within phase-separated compartments.
Another remarkable aspect of the study is its demonstration of machine learning as a formidable tool in unraveling complex biological codes. The authors tackle a problem that defied classical computational methods—parsing a ‘code’ that does not adhere to simplistic or linear rules but instead emerges from distributed and context-dependent sequence features. The success of their approach underscores a growing trend in molecular biology, where artificial intelligence complements experimental data to solve intricate puzzles related to protein function and cellular architecture.
Beyond its scientific implications, the study holds promise for synthetic biology and bioengineering. By harnessing the predictive power of the model, researchers can design proteins with customized localization profiles, generating synthetic condensates with tailored properties or modulating existing ones for desired cellular outcomes. Such capabilities open avenues in biotechnology, including the construction of intracellular reaction centers or the sequestration of deleterious proteins, with far-reaching applications from drug development to tissue engineering.
Critically, the study also confronts the limitations and challenges of the machine learning approach. The complexity of protein condensates, influenced by transient interactions and cellular context, means that predictions, while robust, require cautious interpretation. The authors emphasize the need for ongoing integration of experimental validation and refinement of computational models to enhance predictive accuracy across diverse cell types and physiological conditions.
In practical terms, this work is timely given the explosion of interest in phase separation phenomena over the past decade. The recognition that aberrant condensate behavior contributes to diseases such as ALS, Alzheimer’s, and certain cancers has energized efforts to map the molecular determinants involved. Ditlev and Forman-Kay’s framework equips researchers with a novel method to sift through vast proteomes and identify candidate proteins implicated in condensate biology, accelerating target discovery and hypothesis generation.
The study also navigates the challenge of heterogeneity inherent in condensates, which often consist of overlapping yet distinct protein and RNA components that dynamically exchange with the surrounding cytoplasm or nucleoplasm. By training their models on diverse datasets, the authors capture commonalities as well as unique sequence features dictating condensate specificity. This balance underscores the complexity of biological phase separation and reveals that condensate localization is a modular and context-sensitive phenomenon.
Ultimately, this research redefines our conceptual framework for protein targeting within cells. It moves away from viewing localization merely as a deterministic process guided by discrete signals, towards understanding it as an emergent property encoded in a distributed sequence code influenced by intrinsic disorder, multivalency, and physicochemical heterogeneity. This shift has broad ramifications—from fundamental biology to translational medicine—and highlights the power of interdisciplinary approaches blending computational prowess with molecular insight.
As the field of cellular biophysics continues to unravel, the integration of machine learning promises to be an indispensable ally. The results from Ditlev and Forman-Kay not only provide a powerful tool but also inspire optimism about future discoveries at the intersection of data science and life sciences. Their work stands as a testament to the potential for AI-driven methodologies to decode the dynamic and complex language cells use to orchestrate life at the molecular scale.
The scientific community eagerly anticipates future expansions of this work, including integration with live-cell imaging, biochemical perturbations, and multi-omics datasets to further refine the understanding of condensate targeting. Such multidisciplinary endeavors will accelerate the quest to map the ‘condensate proteome’ comprehensively and elucidate how cellular organization contributes to health and disease.
In conclusion, the study “Beyond peptide targeting sequences: machine learning of cellular condensate localization” offers a visionary blueprint for decoding the enigmatic rules that govern protein condensation and intracellular positioning. By harnessing the synergy between machine learning algorithms and biological data, it transforms our understanding of cellular compartmentalization, opening new frontiers in both basic research and applied biotechnology. As the mysteries of phase separation continue to captivate scientists worldwide, this research lights the way toward a future where we can predict, manipulate, and harness condensate biology with unprecedented precision.
Subject of Research: Protein localization within cellular condensates and the application of machine learning to predict condensate residency beyond classical peptide targeting sequences.
Article Title: Beyond peptide targeting sequences: machine learning of cellular condensate localization.
Article References:
Ditlev, J.A., Forman-Kay, J.D. Beyond peptide targeting sequences: machine learning of cellular condensate localization.
Cell Res (2025). https://doi.org/10.1038/s41422-025-01115-6
Image Credits: AI Generated
Tags: biomolecular condensates researchcellular condensates and phase separationdecoding protein targeting using AIDitlev and Forman-Kay studyintracellular compartmentalization understandinglocalization signals in proteinsmachine learning applications in protein studiesmachine learning in cellular biologymembraneless organelles explorationprotein localization mechanismsprotein residency without sorting signalstraditional protein targeting paradigms
What's Your Reaction?
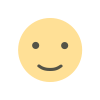
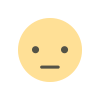
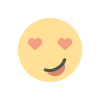
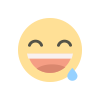
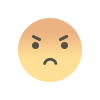
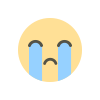
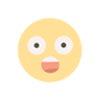