Machine Learning Reveals Flood Risks in Yangtze Delta
In an era increasingly defined by climate uncertainty and the devastating impacts of extreme weather events, understanding the factors that contribute to flood susceptibility is essential for safeguarding vulnerable regions. A recent study spearheaded by Zhu, K., Wang, Z., Lai, C., and their colleagues, published in the International Journal of Disaster Risk Science, delivers groundbreaking […]

In an era increasingly defined by climate uncertainty and the devastating impacts of extreme weather events, understanding the factors that contribute to flood susceptibility is essential for safeguarding vulnerable regions. A recent study spearheaded by Zhu, K., Wang, Z., Lai, C., and their colleagues, published in the International Journal of Disaster Risk Science, delivers groundbreaking insights into flood risk within one of the most economically significant and densely populated regions of the world—the Yangtze River Delta. Their research leverages cutting-edge machine learning techniques to dissect and quantify the multifaceted environmental and anthropogenic factors that elevate flood susceptibility in this critical geographic nexus.
The Yangtze River Delta, spanning expansive territories of China’s eastern coast, is historically prone to flooding events due to its unique topography, hydrological characteristics, and intense urban development. The study’s significance stems from its comprehensive approach: integrating vast datasets across climatic patterns, land use, soil properties, topography, and hydrological dynamics, and applying sophisticated algorithms capable of identifying subtle, nonlinear interactions among these variables. By doing so, the researchers move beyond traditional flood risk assessments that often rely on limited data or oversimplified models.
At the core of their analysis lay several machine learning models, including random forests, gradient boosting machines, and artificial neural networks. These algorithms were trained on extensive spatial and temporal datasets, enabling the research team to develop predictive models with high accuracy in identifying flood-prone areas. This methodological choice is instrumental, as machine learning’s ability to handle complex, high-dimensional data offers unparalleled opportunities to refine hazard assessment frameworks and inform disaster risk management strategies.
One of the standout findings of this study is the identification of the relative importance of various determinants shaping flood susceptibility. While climatic variables such as extreme rainfall intensity and frequency remain primary drivers, human-induced changes—most notably urban expansion and land-use transformation—play an equally critical, sometimes underestimated role. The proliferation of impervious surfaces within urban centers disrupts natural water infiltration and elevates runoff, exacerbating flood risks. Simultaneously, modifications in river channel morphology, often resulting from infrastructural projects, alter hydrodynamics and increase flood vulnerability downstream.
The Yangtze River Delta’s characteristic flat terrain, combined with its dense network of rivers and canals, complicates flood risk scenarios and demands localized assessments. The machine learning models enabled by the researchers could capture subtle topographical variations and microclimatic influences that traditional models might overlook. For instance, slight elevation differences, proximity to water bodies, soil moisture retention capacity, and vegetation cover emerged as nuanced yet pivotal factors influencing where and how floods initiate and propagate.
Significantly, the study underscores the dynamic nature of flood susceptibility, emphasizing the need for iterative risk evaluations. Climate change projections predict shifts in precipitation patterns and the increasing likelihood of extreme weather phenomena, suggesting that flood-prone zones are not static but evolving entities. Machine learning frameworks, with their capacity for continuous learning and adaptation, hold immense potential in providing real-time risk updates and facilitating responsive urban planning, emergency preparedness, and resilience-building measures.
Further technical analysis revealed that integrating remote sensing data, such as satellite-derived land surface temperature and vegetation indices, enhanced the models’ predictive power. These auxiliary datasets offer vital clues about surface characteristics, ecosystem health, and anthropogenic stressors, all of which modulate the region’s hydrological response. Coupling ground-based observations with high-resolution remote sensing thus constitutes a pivotal methodological advancement in flood susceptibility research.
Another intricate layer unveiled through this work involves soil characteristics, particularly permeability and water retention properties. Variability in soil composition across the Yangtze River Delta affects infiltration rates and groundwater recharge, factors intrinsically linked to flood dynamics. Incorporating detailed pedological data sharpened the models’ spatial precision, enabling differentiation between areas where surface runoff accelerates flood onset and regions where gradual seepage mitigates immediate inundation risks.
The authors also highlight the importance of modeling temporal dynamics, noting that flood susceptibility fluctuates not only spatially but seasonally and inter-annually. Incorporating historic flood records, precipitation trends, and seasonal hydrological cycles allowed the construction of probabilistic flood hazard maps that better reflect actual risk patterns. This temporal sensitivity is a critical advancement from conventional static flood risk maps, which often fail to capture the volatility inherent in natural and human systems.
What elevates this study’s impact beyond academia is the clear pathway it outlines for actionable policy and urban planning interventions. By pinpointing high-risk zones informed by data-driven machine learning outputs, authorities can prioritize infrastructural reinforcements, optimize drainage networks, and design green infrastructure solutions aimed at flood attenuation. Moreover, accurate susceptibility maps support informed land-use zoning, preventing unsustainable development in vulnerable areas and safeguarding critical ecosystems that act as natural buffers.
The research also acknowledges several challenges inherent to data-driven flood modeling, including data quality, spatial and temporal resolution disparities, and the intrinsic uncertainties of environmental systems. The team addresses these limitations through rigorous cross-validation, ensemble modeling approaches, and sensitivity analyses, bolstering the reliability and robustness of the findings. Their transparent discussion of methodological constraints sets a benchmark for future studies aiming to apply machine learning in disaster risk science.
Importantly, the methodology and insights from this work have strong transferability to other deltas and flood-prone systems worldwide. The Yangtze River Delta’s complex interplay of natural and human factors is mirrored in many coastal, riverine, and urban agglomerations globally, underlining the broader applicability of machine learning-driven flood susceptibility assessment frameworks. This universality suggests that the study’s contribution transcends regional relevance, becoming a vital resource in global water and disaster risk governance.
Additionally, the study championed an interdisciplinary research framework, integrating expertise from geosciences, hydrology, environmental engineering, and data science. This collaboration exemplifies how addressing multifaceted challenges like flooding necessitates blending domain knowledge with advanced computational techniques. The synergistic approach fosters innovation in predictive modeling and risk management, crucial for mounting effective societal responses to climate hazards.
Readers of this research should also appreciate the ethical dimensions embedded within deploying AI and machine learning in disaster risk contexts. Issues of data privacy, equitable access to technological tools, and the potential biases ingrained in algorithmic decision-making require careful consideration. While the study primarily addresses technical aspects, its findings implicitly advocate for transparent, accountable, and inclusive practices when incorporating AI into public safety domains.
The timing of this publication is particularly pertinent as nations worldwide grapple with escalating natural disasters and strive to meet the United Nations Sendai Framework’s goals on disaster risk reduction. By harnessing machine learning’s capabilities, Zhu and colleagues offer a scientifically rigorous and operationally practical avenue to advance flood resilience in a region that serves as an economic powerhouse and population center, thereby setting a precedent for data-driven risk mitigation globally.
In conclusion, this seminal work elegantly demonstrates that the fusion of high-resolution geospatial data with advanced machine learning techniques can profoundly enhance understanding and prediction of flood susceptibilities. The Yangtze River Delta, with its intricate environmental fabric and rapid urban evolution, stands as a testament to the complexities of contemporary flood risk landscapes. Through meticulous analysis and innovative modeling, this study not only charts a path for informed disaster risk management in one of the world’s most vulnerable regions but also paves the way for integrating artificial intelligence into the broader realm of environmental hazard science.
Subject of Research: Evaluation of factors influencing flood susceptibility in the Yangtze River Delta using machine learning approaches.
Article Title: Evaluating Factors Affecting Flood Susceptibility in the Yangtze River Delta Using Machine Learning Methods.
Article References:
Zhu, K., Wang, Z., Lai, C. et al. Evaluating Factors Affecting Flood Susceptibility in the Yangtze River Delta Using Machine Learning Methods. Int J Disaster Risk Sci 15, 738–753 (2024). https://doi.org/10.1007/s13753-024-00590-6
Image Credits: AI Generated
Tags: advanced algorithms for flood predictionclimate change and floodingcomprehensive flood risk analysisdata-driven disaster risk managementenvironmental factors influencing floodsflood risk assessmenthydrological modeling techniquesmachine learning applications in flood predictionnonlinear interactions in flood modelingurban development and flood riskYangtze River Delta flood susceptibility
What's Your Reaction?
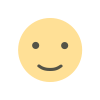
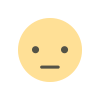
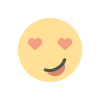
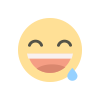
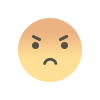
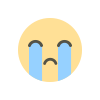
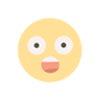