Machine Learning Predicts Cement Clinker Phases Industrially
In an era marked by rapid advancements in artificial intelligence and machine learning, the industrial sector is witnessing profound transformations in how materials and processes are understood and optimized. Among these, cement production—a cornerstone of global infrastructure—stands on the cusp of a technological revolution. Cement manufacture, long criticized for its environmental footprint and energy intensity, […]

In an era marked by rapid advancements in artificial intelligence and machine learning, the industrial sector is witnessing profound transformations in how materials and processes are understood and optimized. Among these, cement production—a cornerstone of global infrastructure—stands on the cusp of a technological revolution. Cement manufacture, long criticized for its environmental footprint and energy intensity, now finds a powerful ally in machine learning methodologies that promise not only to streamline production but also to drastically reduce waste and emissions. A groundbreaking study led by Fayaz, Montiel-Bohórquez, Bishnoi, and colleagues heralds a new chapter in the industrial-scale prediction of cement clinker phases by leveraging advanced AI techniques.
Cement clinker—the intermediate product formed in the high-temperature kiln during cement production—is composed of multiple crystalline phases. The precise composition and distribution of these phases critically determine the final cement’s physical properties, including strength, durability, and hydration behavior. Traditionally, predicting clinker phase compositions has relied heavily on extensive experimental trials and thermodynamic calculations, which are labor-intensive and often fail to capture the rapid variations encountered in industrial environments. Machine learning approaches provide an innovative pathway to overcome these limitations by extracting nuanced patterns from vast process data, enabling highly accurate and rapid predictions.
The study by Fayaz et al. introduces a comprehensive machine learning framework trained on large-scale industrial datasets, combining chemical composition, kiln operating conditions, and output properties. By synthesizing this multi-dimensional information, their model achieves unprecedented precision in predicting the relative abundance of critical clinker phases such as alite, belite, aluminate, and ferrite. This capability enables real-time adjustments in the production process, ensuring optimal clinker quality while minimizing energy consumption and unwanted emissions.
One of the significant challenges in applying machine learning to industrial cement production lies in the heterogeneity and noise inherent in operational data. Variability in raw material sources, temperature gradients, and kiln dynamics creates a complex, nonlinear system. The model developed integrates robust data preprocessing techniques and sophisticated feature engineering, allowing it to discern meaningful relationships without being misled by spurious correlations or outliers. This resilience makes it adaptable across diverse production plants and geological contexts.
Beyond predictive accuracy, the interpretability of the model is a pivotal concern highlighted by the researchers. Unlike black-box AI models that provide little insight into causal mechanisms, the framework emphasizes explainability by elucidating which input variables most heavily influence clinker phase formation. This transparency empowers engineers to not only predict but understand and control the underlying chemical reactions within the kiln. Such insights pave the way for rational process engineering and innovation in raw material selection and heating protocols.
The impact of this research extends far beyond theoretical elegance. Cement production is responsible for approximately 8% of global CO2 emissions, primarily due to the calcination of limestone and fossil fuel combustion required for clinker synthesis. By enabling precise control over clinker phases, machine learning allows plants to reduce clinker-to-cement ratios, optimize burn temperatures, and better utilize alternative fuels and supplementary cementitious materials. These adjustments translate directly into reduced greenhouse gas emissions and energy costs, aligning with global sustainability goals.
Moreover, the model facilitates accelerated product development cycles. Traditionally, introducing new cement formulations or scaling up novel industrial processes involves lengthy trial-and-error phases. The predictive tool streamlines this process by virtually simulating clinker phase outcomes under various conditions. This capability not only shortens innovation timelines but also reduces material waste and laboratory costs, encouraging experimentation with greener and more economical raw materials.
The researchers also demonstrate how their approach integrates seamlessly into existing industrial control systems via real-time data analytics pipelines. Such operationalization ensures continuous monitoring and adaptive control of clinker synthesis, effectively transforming kilns into intelligent manufacturing units. This integration highlights a promising synergy between traditional industrial engineering and cutting-edge AI, reaffirming the potential for Industry 4.0 applications in heavy manufacturing sectors.
While the benefits are manifold, the implementation of machine learning in cement manufacturing also raises critical considerations around data governance, cybersecurity, and workforce transition. The study emphasizes the necessity of robust data management protocols to safeguard proprietary information and ensure data quality. Additionally, adapting to AI-driven processes requires upskilling the existing workforce to foster collaboration between human expertise and automated decision-making systems.
Importantly, the generalizability of the model across different global regions was rigorously tested. Using datasets from multiple cement plants on distinct continents, encompassing a range of raw materials and kiln designs, the model maintained consistent performance. This scalability suggests broad applicability, enabling even smaller or less technologically advanced plants to benefit from AI enhancements without prohibitive investment.
In summarizing their contributions, Fayaz and colleagues call for a paradigm shift in cement production—from empirical and heuristic approaches to data-driven, predictive manufacturing. They envision a future where AI not only increases operational efficiency but also facilitates transparent and environmentally responsible cement production, essential for meeting urbanization and infrastructure demands under mounting climate pressures.
As the cement industry grapples with the dual challenge of economic competitiveness and sustainability, the integration of machine learning for phase prediction emerges as a beacon of innovation. This advancement is not merely a technical feat but a transformative step towards smarter, cleaner, and more agile infrastructure development worldwide. The work stands as a testament to the power of interdisciplinary research—melding materials science, chemical engineering, and artificial intelligence to redefine an age-old industrial process.
With the advent of this technology, policymakers and industry leaders have a powerful tool to drive decarbonization strategies. The research underscores the importance of investing in digital transformation as part of broader environmental initiatives. It also ignites a dialogue on how emerging technologies can reconcile industrial growth with planetary stewardship, catalyzing a more sustainable industrial revolution.
Looking forward, the research team highlights potential avenues for enhancing the model. Incorporating additional sensor data, such as in-situ spectroscopy or imaging techniques, could further refine predictions and provide deeper insights into clinker microstructures. Additionally, blending physics-based simulations with data-driven models could yield hybrid approaches that balance mechanistic understanding with empirical power.
Ultimately, this pioneering work exemplifies how machine learning transcends academic boundaries to deliver tangible industrial benefits. It challenges the cement sector to rethink traditional paradigms and embrace innovation at scale, setting a new standard for materials manufacturing in the 21st century. As the global community seeks resilient infrastructure solutions compatible with environmental constraints, such advancements will be indispensable in shaping a sustainable future.
Subject of Research: Industrial-scale prediction of cement clinker phases using machine learning
Article Title: Industrial-scale prediction of cement clinker phases using machine learning
Article References: Fayaz, S.J., Montiel-Bohórquez, N., Bishnoi, S. et al. Industrial-scale prediction of cement clinker phases using machine learning. Commun Eng 4, 94 (2025). https://doi.org/10.1038/s44172-025-00432-3
Image Credits: AI Generated
Tags: advanced AI techniques in industrydata-driven approaches in construction materialsenergy efficiency in cement manufacturingenhancing cement properties with AIenvironmental impact of cement productionindustrial applications of AIinnovations in industrial material sciencemachine learning for sustainable manufacturingmachine learning in cement productionoptimizing cement manufacturing processespredicting cement clinker phasesreducing cement production emissions
What's Your Reaction?
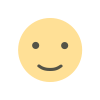
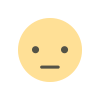
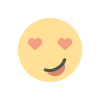
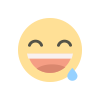
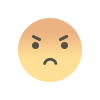
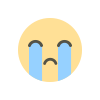
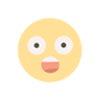