KNN Predicts Hemorrhagic Shock Severity Non-Invasively
In a groundbreaking advancement that could redefine trauma care in prehospital settings, researchers have developed a sophisticated machine learning model to non-invasively predict the severity of hemorrhagic shock. This innovative model, based on the K-nearest neighbors (KNN) algorithm, integrates multiple physiological parameters—mean arterial pressure (MAP), buccal mucosal carbon dioxide (P_BU_CO2), transcutaneous oxygen (P_TC_O2), and pulse […]

In a groundbreaking advancement that could redefine trauma care in prehospital settings, researchers have developed a sophisticated machine learning model to non-invasively predict the severity of hemorrhagic shock. This innovative model, based on the K-nearest neighbors (KNN) algorithm, integrates multiple physiological parameters—mean arterial pressure (MAP), buccal mucosal carbon dioxide (P_BU_CO2), transcutaneous oxygen (P_TC_O2), and pulse pressure variation (PPV)—to deliver highly accurate diagnostic predictions. The study, recently published in BioMedical Engineering OnLine, signals a significant leap forward in early trauma intervention and stratification.
Hemorrhagic shock remains one of the leading causes of preventable death following severe trauma, particularly in prehospital environments where rapid assessment is critical but challenging. Traditional monitoring techniques often rely on single-parameter measures that fail to capture the complex physiological interplay during hemorrhage. Recognizing this limitation, a team led by Zhao and colleagues sought to harness the power of data-driven models by combining multiple indicators reflecting cardiovascular and tissue oxygenation status.
The researchers employed Wistar rats subjected to varying degrees of controlled blood loss to simulate hemorrhagic shock. Forty-five animals were divided into five experimental groups, each representing a different severity level. Throughout these experiments, continuous monitoring of MAP, P_BU_CO2, P_TC_O2, and PPV was conducted, ensuring a comprehensive dataset depicting the physiological state across the shock spectrum.
Central to this investigation was the deployment of the KNN algorithm—a non-parametric method widely celebrated for its simplicity and effectiveness in classification tasks. To optimize the model, the team applied leave-one-out cross-validation, a rigorous approach that examines the dataset iteratively to identify the optimal number of neighbors, denoted as ‘K’. Their analysis revealed that a value of K=3 yielded the best performance, balancing accuracy with generalizability, and minimizing overfitting risks.
In addition to KNN, a comparative model based on the support vector machine (SVM) algorithm was also constructed. SVMs, known for their capacity to handle high-dimensional datasets, have been widely utilized in biomedical applications. By benchmarking KNN against SVM, the study provided a robust evaluation of the relative strengths and weaknesses of these machine learning techniques in shock prediction.
The evaluation metrics used to compare the models were comprehensive. Confusion matrices offered insights into true and false positive classifications at different shock severity levels, while receiver operating characteristic (ROC) curves and their area under the curve (AUC) quantified the models’ discriminative power. Remarkably, the KNN-based model outperformed the SVM counterpart with an accuracy exceeding 94%, complemented by an impressive AUC of 1.0 in the 7:3 training-testing data split and 0.97 in the 6:4 split. Conversely, the SVM model achieved lower accuracies around 84%, though still demonstrating respectable AUC values.
Such high accuracy in predicting hemorrhagic shock severity non-invasively holds profound clinical implications. Early and precise stratification of patients according to shock level can inform timely triage decisions and guide interventions, potentially reducing mortality rates. Moreover, the utilization of non-invasive measurements makes this approach highly applicable in dynamic and resource-limited prehospital settings, where rapid yet reliable assessment tools are scarce.
The integrated physiological parameters harnessed in the model were carefully chosen for their sensitivity to blood loss and tissue perfusion changes. MAP reflects systemic arterial pressure and perfusion adequacy, while P_BU_CO2 offers insight into local CO₂ accumulation in buccal mucosa—a proxy of tissue hypoxia. P_TC_O2 measures oxygen tension through the skin, representing oxygen delivery at peripheral tissues, and PPV indicates volume status and fluid responsiveness, critical during shock states. By combining these metrics, the model overcomes the limitations posed by individual parameters, enhancing predictive robustness.
An additional highlight of the study lies in its methodological rigor, particularly the use of continuous measurement during controlled hemorrhage, allowing for rich temporal data capturing the dynamic physiological changes. This data-driven approach underscores the potential of machine learning algorithms to reinterpret complex biomedical signals far beyond traditional threshold-based diagnostics.
While the current study utilized animal models to establish proof of concept, the findings lay a solid foundation for forthcoming clinical validation. Transitioning KNN-based prediction systems into human prehospital care necessitates adapting sensors and algorithms for real-world, heterogenous patient populations. However, the demonstrated stability and accuracy in a controlled setting inspire optimism about future integration into emergency medical services workflows.
The researchers also reflect on the broader scope of such multi-parameter machine learning approaches in critical care monitoring. As wearable and portable biosensors continue to evolve, combining physiological metrics through intelligent algorithms could herald a new era of personalized, real-time monitoring, capable of anticipating patient deterioration before clinical signs manifest overtly.
In summary, Zhao et al. have introduced an elegantly designed and highly efficient KNN model that leverages key circulatory and tissue oxygenation indicators to predict hemorrhagic shock severity with unprecedented accuracy. This innovation signifies a pivotal step toward non-invasive, data-driven triage tools that could transform prehospital trauma care, improving outcomes through early detection and stratified intervention.
Looking ahead, the fusion of physiological monitoring with advanced analytics poses exciting possibilities across medicine. By embracing multi-dimensional data, predictive models like this may soon extend to a broader array of critical illnesses, enabling clinicians to make faster, more informed decisions and ultimately saving more lives in emergencies.
—
Subject of Research: Non-invasive multi-parameter prediction of hemorrhagic shock severity using machine learning
Article Title: A KNN-based model for non-invasive prediction of hemorrhagic shock severity in prehospital settings: integrating MAP, P_BU_CO2, P_TC_O2, and PPV
Article References: Zhao, P., Pan, W., Zou, X. et al. A KNN-based model for non-invasive prediction of hemorrhagic shock severity in prehospital settings: integrating MAP, PBUCO2, PTCO2, and PPV. BioMed Eng OnLine 24, 62 (2025). https://doi.org/10.1186/s12938-025-01394-5
Image Credits: AI Generated
DOI: https://doi.org/10.1186/s12938-025-01394-5
Tags: cardiovascular assessment techniquescontinuous monitoring in critical caredata-driven approaches in trauma managementearly intervention in severe traumaexperimental models in medical researchimproving patient outcomes in trauma settingsKNN algorithm for hemorrhagic shock predictionmachine learning in medical applicationsnon-invasive trauma care technologyphysiological parameter integration for diagnosticspredictive modeling in hemorrhagic conditionsprehospital emergency care advancements
What's Your Reaction?
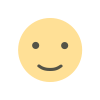
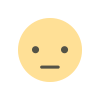
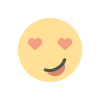
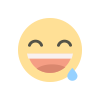
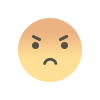
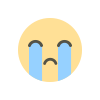
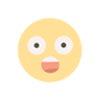