Introducing the Neural Partially Linear Additive Model: A Breakthrough in Data Analysis
Revolutionizing Interpretability in Machine Learning with Neural Partially Linear Additive Models In recent years, the quest for interpretability in machine learning has gained significant traction within the scientific community. While the complexities and intricacies of machine learning models have grown exponentially, so too has the necessity for researchers and practitioners to comprehend the principle workings […]

Revolutionizing Interpretability in Machine Learning with Neural Partially Linear Additive Models
In recent years, the quest for interpretability in machine learning has gained significant traction within the scientific community. While the complexities and intricacies of machine learning models have grown exponentially, so too has the necessity for researchers and practitioners to comprehend the principle workings of these systems. Enter the concept of partially linear additive models (PLAMs), which present a harmonious blend of the simplicity associated with generalized linear models and the flexibility inherent in generalized additive models. This innovative approach aims to tackle critical challenges in interpretability related to feature selection and structure discovery, areas that remain pivotal for effective model implementation.
The limitations of existing PLAMs have spurred researchers to explore methodologies that can enhance fitting capabilities. A noteworthy advancement emerged from a research team led by Han Li, which recently published a groundbreaking study on December 15, 2024, in “Frontiers of Computer Science.” Published in collaboration with Higher Education Press and Springer Nature, the study introduces a novel framework called the Neural Partially Linear Additive Model (NPLAM). This sophisticated model leverages the power of neural networks to discern between significant, linear, and nonlinear features automatically, heralding a new era in the interpretability of machine learning algorithms.
At the heart of NPLAM lies the distinctive combination of neural networks and PLAMs. The use of neural networks significantly enhances the model’s capacity to fit complex data structures compared to traditional spline functions, especially when the same number of parameters are employed. This improved fitting capability allows researchers to harness the intricacies of data without sacrificing the interpretability that often accompanies simpler models. Moreover, the architectural design of NPLAM includes learnable gates and a sparsity regularization term, preserving the functionalities essential for feature selection and structure discovery.
One particularly ingenious aspect of this research involves the analysis of the hypothesis space and optimization challenges present within the framework of PLAMs. By utilizing neural networks, the authors have effectively constructed sub-models capable of addressing each nonlinear feature without the need to predetermine basis functions. This not only augments efficiency but also maintains a minimal accuracy loss—a crucial consideration when dealing with real-world data complexities.
A pivotal innovation within NPLAM is the introduction of two types of gates: a learnable feature selection gate and a structure discovery gate. These gates facilitate a nuanced evaluation of the input data, helping to classify features as either important or linear in nature. The application of a lasso penalization further enhances model selection across three dimensions, ensuring a careful curation of relevant features, delineating which should be fitted linearly and which nonlinearly, and promoting sparse weight distributions throughout the neural network construct.
Theoretical foundations supporting NPLAM are robust, as the researchers establish sample complexity error bounds utilizing Rademacher complexity. This mathematical framework provides a solid basis for understanding the model’s performance and reliability. Empirical evidence presented in the study validates the effectiveness of this dual-gate approach combined with lasso regularization, showcasing how these innovations can proficiently tackle interpretability challenges that plague many complex machine learning models.
Looking forward, the researchers highlight numerous potential avenues for enhancing this work. Future explorations could delve into the interactions among input features, presenting an opportunity to augment the expressiveness of NPLAM. Additionally, the design of subsequent iterations of the model could integrate advanced techniques for feature ranking, refining the model’s capability to deliver interpretations that are both insightful and actionable.
In conclusion, the Neural Partially Linear Additive Model represents a significant leap forward in machine learning’s interpretability landscape. By marrying the structural clarity of partially linear additive models with the dynamic capabilities of neural networks, this innovation paves the way for a new class of machine learning applications. Researchers and industry professionals alike will benefit from understanding these developments, as they enhance the explainability of complex models while simultaneously improving their capacity to extract meaningful insights from intricate data sets.
As we herald this new chapter in machine learning, the implications for fields ranging from healthcare to finance could be profound. By improving interpretability in machine learning, we not only enhance predictive performance but also facilitate accountability and transparency, thus ultimately strengthening the trust in and adoption of these powerful analytical tools.
Subject of Research: Machine Learning Interpretability
Article Title: Neural Partially Linear Additive Model
News Publication Date: December 15, 2024
Web References: Frontiers of Computer Science
References: DOI: 10.1007/s11704-023-2662-3
Image Credits: Liangxuan ZHU, Han LI, Xuelin ZHANG, Lingjuan WU, Hong CHEN
Keywords
Machine Learning, Interpretability, Neural Networks, Partially Linear Additive Models, Feature Selection, Structure Discovery, Model Selection, Lasso Regularization.
What's Your Reaction?
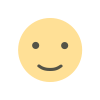
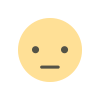
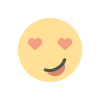
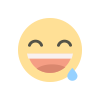
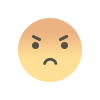
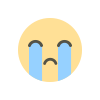
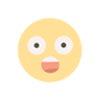